Fault identification for photovoltaic systems using a multi-output deep learning approach
Expert Systems with Applications(2023)
摘要
Fault classification and localization are imperative to maintaining an efficient photovoltaic (PV) system. Due to the environmental factors that PV systems function in, they can be prone to multiple types of fault conditions such as string-to-string (SS), string-to-ground (SG), and open-circuit faults (OC). If left undetected, these faults can lead to power loss and eventually system failures. To help mitigate such outcomes, this paper presents an approach to detect, classify, and locate SS, SG, and OC faults using multi-output deep learning (DL) algorithms: convolutional neural networks (CNN), long short-term memory (LSTM), and bi-directional long short-term memory (Bi-LSTM) networks. The proposed approach reduces the number of sensors needed per string by 50% compared to the previous approaches in the literature. It also allows for increased scalability and the development of a multi-label dataset through an uncoupled modeling scheme. Fault classification and location are achieved with accuracies of 99.94% and 99.54%, respectively. Further, a comparison is conducted among the proposed DL and conventional machine learning algorithms using multiple evaluation metrics. The proposed method is validated by testing multiple datasets and adding noise. The results obtained reflect high reliability and demonstrate the effectiveness and scalability of the proposed multi-output DL approach.
更多查看译文
关键词
photovoltaic systems,deep learning,fault,multi-output
AI 理解论文
溯源树
样例
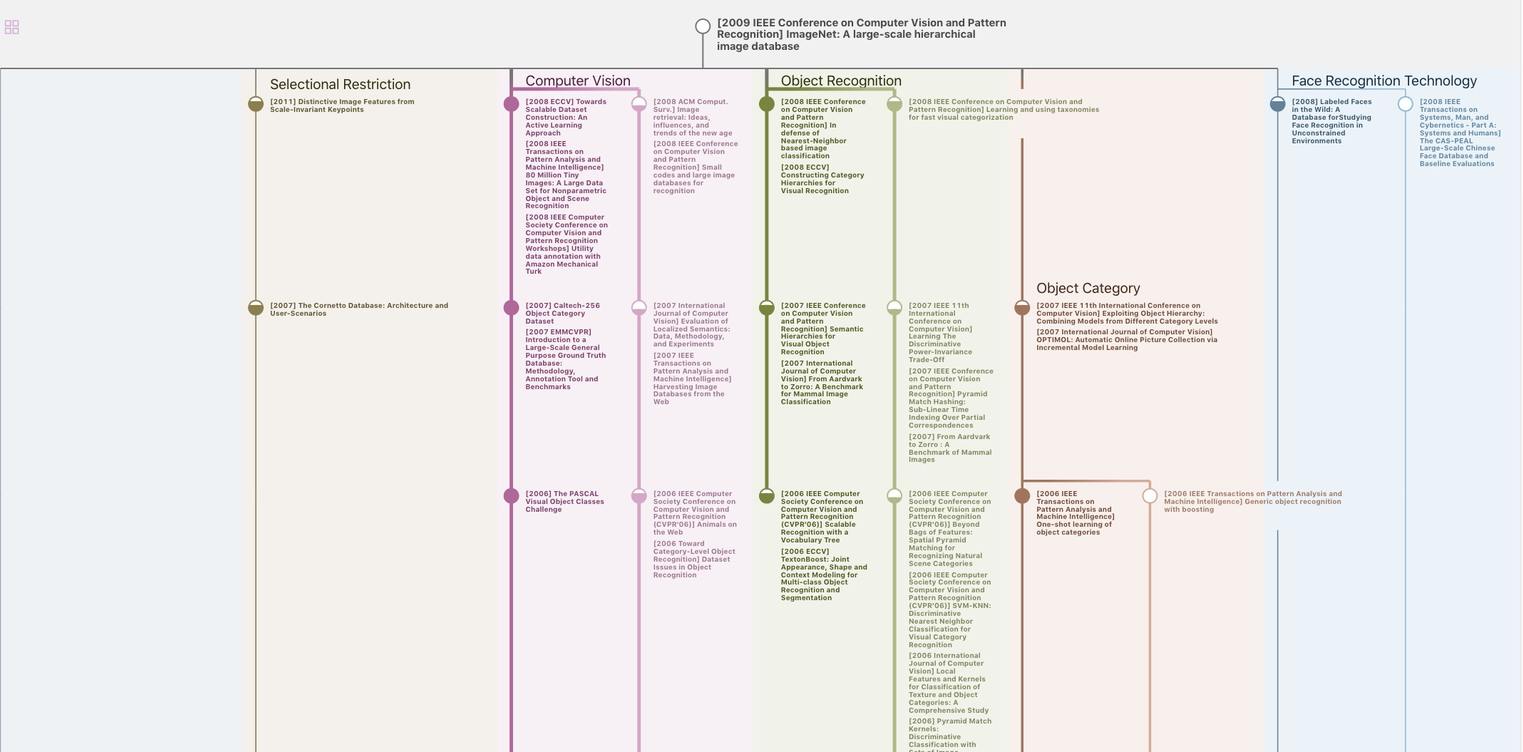
生成溯源树,研究论文发展脉络
Chat Paper
正在生成论文摘要