Evaluating the performance of genomic prediction accounting for effects of single nucleotide polymorphism markers in reproductive traits of Japanese Black cattle
ANIMAL SCIENCE JOURNAL(2023)
摘要
We examined the prediction accuracies of genomic best linear unbiased prediction (GBLUP), various weighted GBLUP according to the degrees of marker effects (WGBLUP) and machine learning (ML) methods, and compared them with traditional BLUP for age at first calving (AFC), calving difficulty (CD), and gestation length in Japanese Black cattle. For WGBLUP, firstly, BayesC and FarmCPU were used to estimate marker effects. Then, we constructed three weighted genomic relationship matrices from information of estimated marker effects in the first step: absolute value of the estimated marker-effect WGBLUP, estimated marker-variance WGBLUP, and genomic-feature WGBLUP. For ML, we applied Gaussian kernel, random forest, extreme gradient boost, and support vector regression. We collected a total of 2583 animals having both phenotypic records and genotypes with 30,105 markers and 16,406 pedigree records. For AFC, prediction accuracies of WGBLUP methods using FarmCPU exceeded BLUP by 25.7%-39.5%. For CD, estimated marker-variance WGBLUP using BayesC achieved the highest prediction accuracy. Among ML methods, extreme gradient boost, support vector regression, and Gaussian kernel increased prediction accuracies by 28.4%, 19.0%, and 36.4% for AFC, CD, and gestation length compared with BLUP, respectively. Thus, prediction performance could be improved using suitable WGBLUP and ML methods according to target reproductive traits for the population used.
更多查看译文
关键词
genomic prediction,Japanese Black cattle,machine learning,reproductive trait,weighted genomic best linear unbiased prediction
AI 理解论文
溯源树
样例
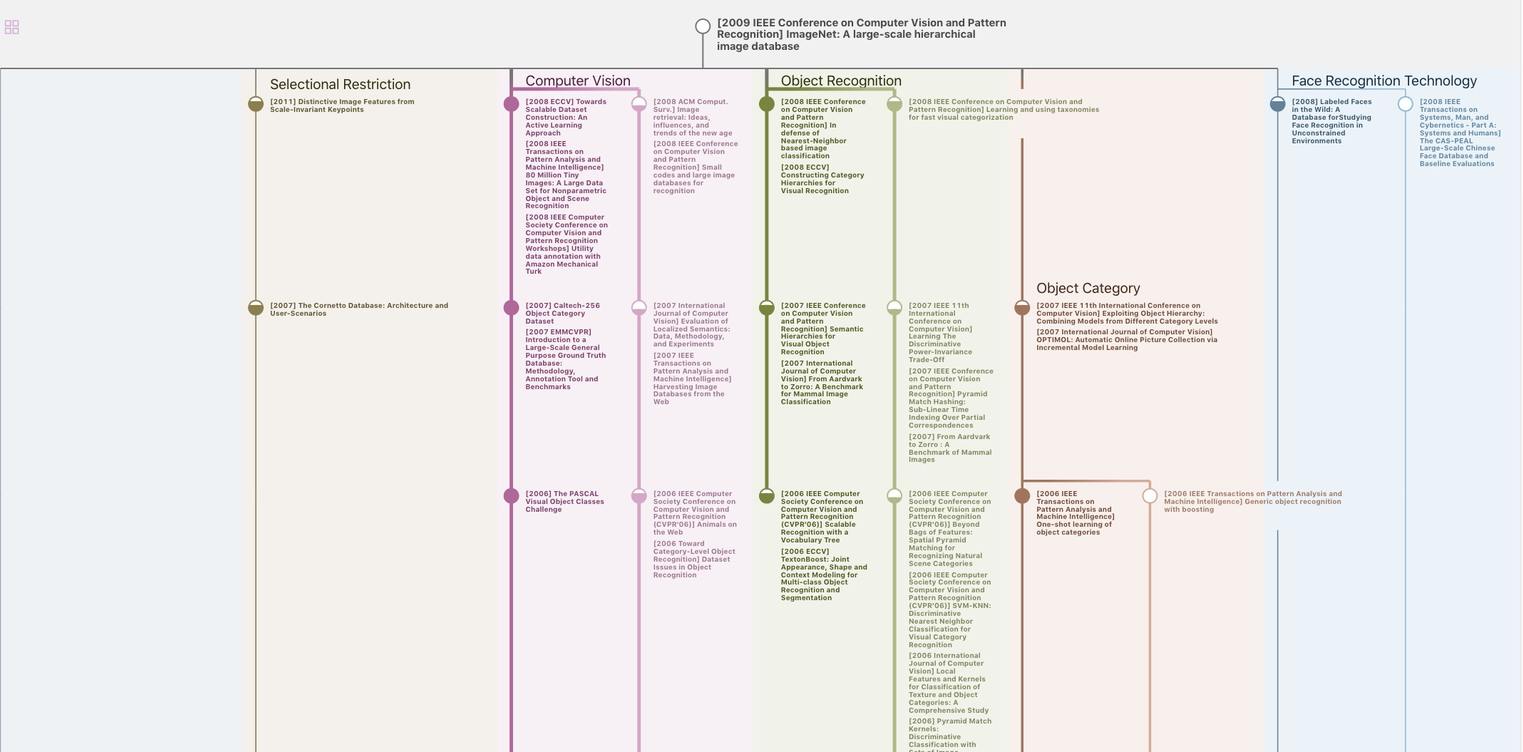
生成溯源树,研究论文发展脉络
Chat Paper
正在生成论文摘要