Strengthening transferability of adversarial examples by adaptive inertia and amplitude spectrum dropout.
Neural networks : the official journal of the International Neural Network Society(2023)
摘要
Deep neural networks are sensitive to adversarial examples and would produce wrong results with high confidence. However, most existing attack methods exhibit weak transferability, especially for adversarially trained models and defense models. In this paper, two methods are proposed to generate highly transferable adversarial examples, namely Adaptive Inertia Iterative Fast Gradient Sign Method (AdaI-FGSM) and Amplitude Spectrum Dropout Method (ASDM). Specifically, AdaI-FGSM aims to integrate adaptive inertia into the gradient-based attack, and leverage the looking ahead property to search for a flatter maximum, which is essential to strengthen the transferability of adversarial examples. By introducing a loss-preserving transformation in the frequency domain, the proposed ASDM with the dropout invariance property can craft the copies of input images to overcome the poor generalization on the surrogate models. Furthermore, AdaI-FGSM and ASDM can be naturally integrated as an efficient gradient-based attack method to yield more transferable adversarial examples. Extensive experimental results on the ImageNet-compatible dataset demonstrate that higher transferability is achieved by our method than some advanced gradient-based attacks.
更多查看译文
关键词
Adversarial examples,Transferability,Gradient-based attack,Adaptive inertia,Amplitude spectrum dropout
AI 理解论文
溯源树
样例
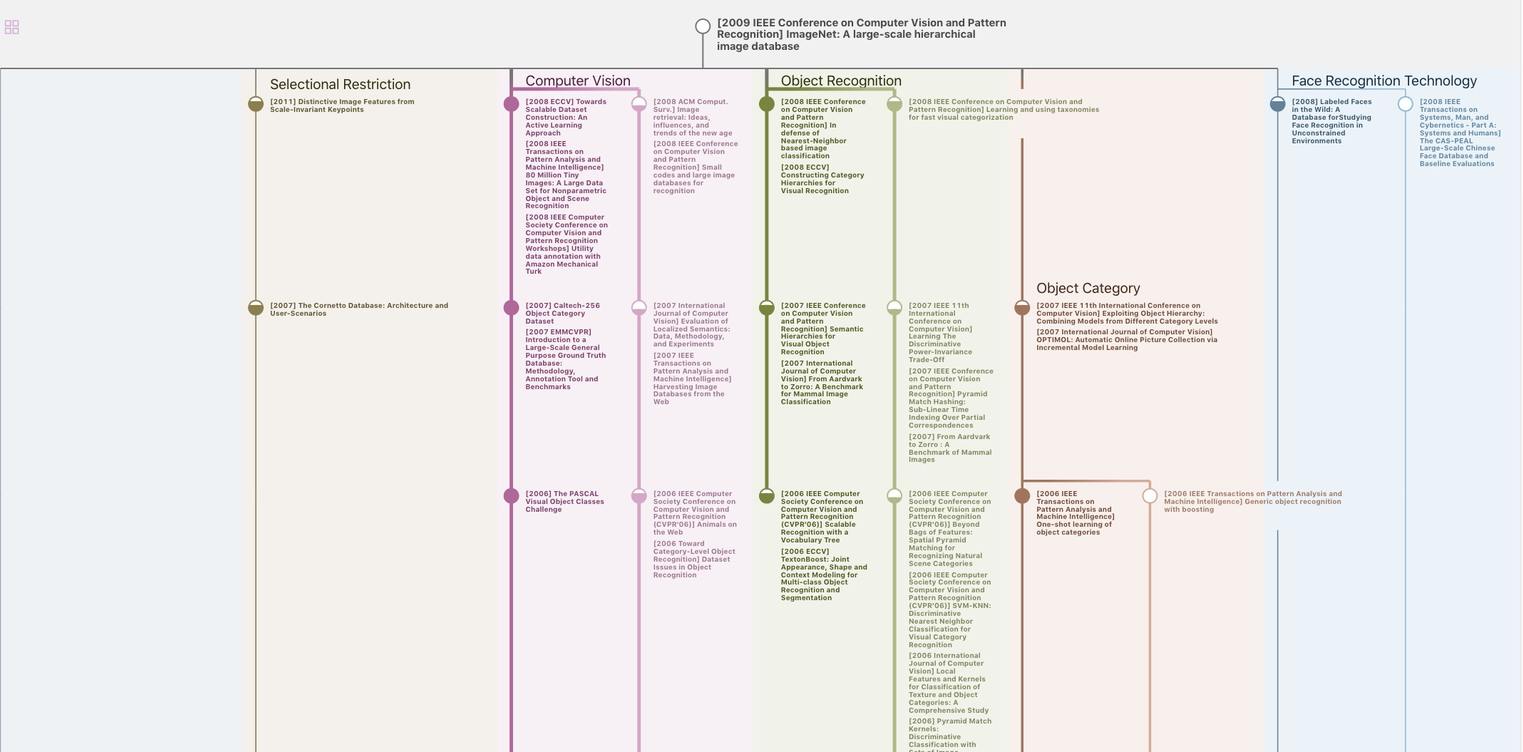
生成溯源树,研究论文发展脉络
Chat Paper
正在生成论文摘要