Objective discrimination of bimodal speech using frequency following responses.
Hearing research(2023)
摘要
Bimodal hearing, in which a contralateral hearing aid is combined with a cochlear implant (CI), provides greater speech recognition benefits than using a CI alone. Factors predicting individual bimodal patient success are not fully understood. Previous studies have shown that bimodal benefits may be driven by a patient's ability to extract fundamental frequency (f0) and/or temporal fine structure cues (e.g., F1). Both of these features may be represented in frequency following responses (FFR) to bimodal speech. Thus, the goals of this study were to: 1) parametrically examine neural encoding of f0 and F1 in simulated bimodal speech conditions; 2) examine objective discrimination of FFRs to bimodal speech conditions using machine learning; 3) explore whether FFRs are predictive of perceptual bimodal benefit. Three vowels (/ε/, /i/, and /ʊ/) with identical f0 were manipulated by a vocoder (right ear) and low-pass filters (left ear) to create five bimodal simulations for evoking FFRs: Vocoder-only, Vocoder +125 Hz, Vocoder +250 Hz, Vocoder +500 Hz, and Vocoder +750 Hz. Perceptual performance on the BKB-SIN test was also measured using the same five configurations. Results suggested that neural representation of f0 and F1 FFR components were enhanced with increasing acoustic bandwidth in the simulated "non-implanted" ear. As spectral differences between vowels emerged in the FFRs with increased acoustic bandwidth, FFRs were more accurately classified and discriminated using a machine learning algorithm. Enhancement of f0 and F1 neural encoding with increasing bandwidth were collectively predictive of perceptual bimodal benefit on a speech-in-noise task. Given these results, FFR may be a useful tool to objectively assess individual variability in bimodal hearing.
更多查看译文
关键词
Cochlear implants,Hearing aids,Bimodal benefits,Frequency following responses,Machine learning
AI 理解论文
溯源树
样例
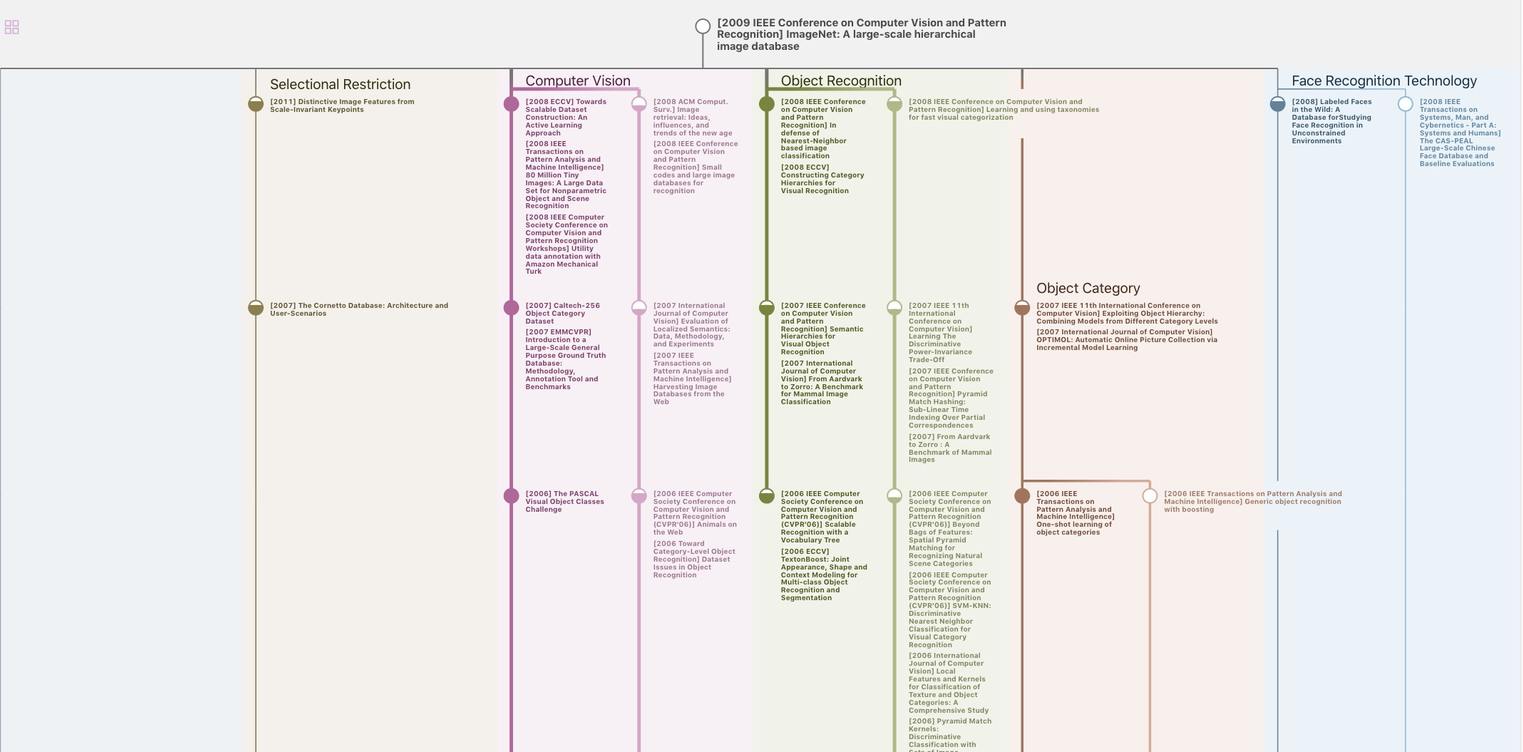
生成溯源树,研究论文发展脉络
Chat Paper
正在生成论文摘要