Deep Learning Classification of Angle Closure based on Anterior Segment OCT
OPHTHALMOLOGY GLAUCOMA(2024)
摘要
Purpose: To assess the performance and generalizability of a convolutional neural network (CNN) model for objective and high -throughput identification of primary angle -closure disease (PACD) as well as PACD stage differentiation on anterior segment swept -source OCT (AS-OCT). Design: Cross-sectional. Participants: Patients from 3 different eye centers across China and Singapore were recruited for this study. Eight hundred forty-one eyes from the 2 Chinese centers were divided into 170 control eyes, 488 PACS, and 183 PAC + PACG eyes. An additional 300 eyes were recruited from Singapore National Eye Center as a testing data set, divided into 100 control eyes, 100 PACS, and 100 PAC + PACG eyes. Methods: Each participant underwent standardized ophthalmic examination and was classified by the presiding physician as either control, primary angle -closure suspect (PACS), primary angle closure (PAC), or primary angle -closure glaucoma (PACG). Deep Learning model was used to train 3 different CNN classifiers: classifier 1 aimed to separate control versus PACS versus PAC + PACG; classifier 2 aimed to separate control versus PACD; and classifier 3 aimed to separate PACS versus PAC + PACG. All classifiers were evaluated on independent validation sets from the same region, China and further tested using data from a different country, Singapore. Main Outcome Measures: Area under receiver operator characteristic curve (AUC), precision, and recall. Results: Classifier 1 achieved an AUC of 0.96 on validation set from the same region, but dropped to an AUC of 0.84 on test set from a different country. Classifier 2 achieved the most generalizable performance with an AUC of 0.96 on validation set and AUC of 0.95 on test set. Classifier 3 showed the poorest performance, with an AUC of 0.83 and 0.64 on test and validation data sets, respectively. Conclusions: Convolutional neural network classifiers can effectively distinguish PACD from controls on AS-OCT with good generalizability across different patient cohorts. However, their performance is moderate when trying to distinguish PACS versus PAC + PACG. Financial Disclosures: The authors have no proprietary or commercial interest in any materials discussed in this article. (c) 2023 Published by Elsevier Inc. on behalf of the American Academy of Ophthalmology
更多查看译文
关键词
AI model generalizability,Angle closure,Artificial intelligence,Disease screening,Disease stratification
AI 理解论文
溯源树
样例
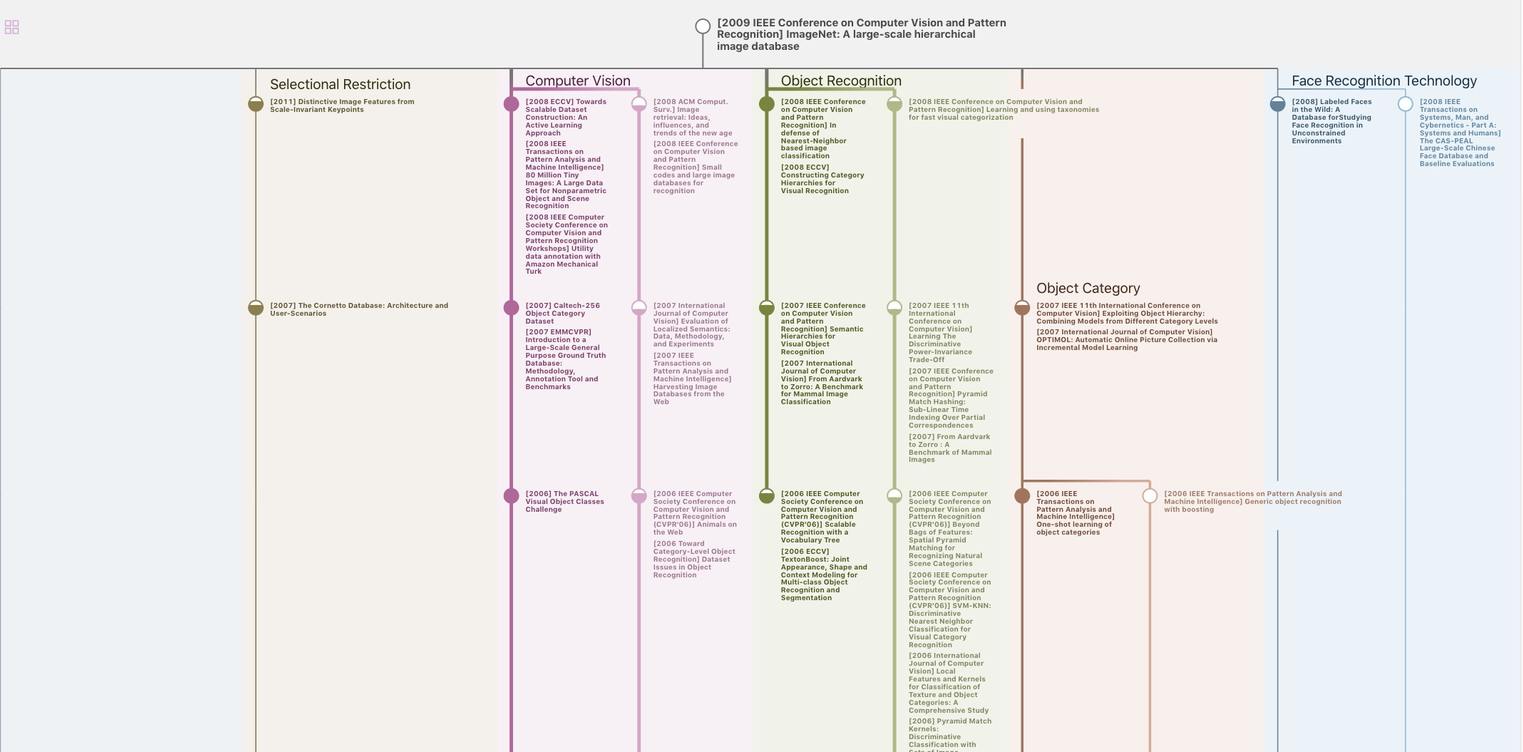
生成溯源树,研究论文发展脉络
Chat Paper
正在生成论文摘要