Toward Personalized Federated Learning Via Group Collaboration in IIoT
IEEE Transactions on Industrial Informatics(2023)
摘要
Despite the rapid growth of successful examples of Federated Learning (FL), it faces the heterogeneity of data, models, and devices in emerging applications of Industrial Internet of Things (IIoT). Existing efforts mainly focus on training multiple personalized models by adopting a global, cluster, or pairwise fashion. However, the global collaboration does not work well in case of the non-IID data distribution, cluster collaboration is often inefficient due to the single cluster pattern and high computational cost, and pairwise collaboration incurs the limitations of collaboration scope and communication efficiency. To address the problems, we propose a novel personalized FL (PFL) framework with the game-theoretic insights, called group collaboration, to overcome the shortcomings of status quo. Specifically, we first formulate the group collaboration in PFL as a multileader multifollower Stackelberg game, and then develop an e-better response to efficiently characterize its unique equilibrium through cautiously proposing a potential function. Since the existing equilibrium may not be optimal, we further design a Robin Hood mechanism by using the idea of transferable utility to improve the performance of the training model. Meanwhile, we also prove that the new mechanism is sustainable and can converge to a stable state with an upper bound of the training loss. Last, extensive experiments on a simulated dataset and four real-world datasets demonstrate the superiority of our proposed approach compared to the state of the art.
更多查看译文
关键词
Federated learning (FL),group collaboration,heterogeneity,industrial Internet of Things (IIoT),potential game
AI 理解论文
溯源树
样例
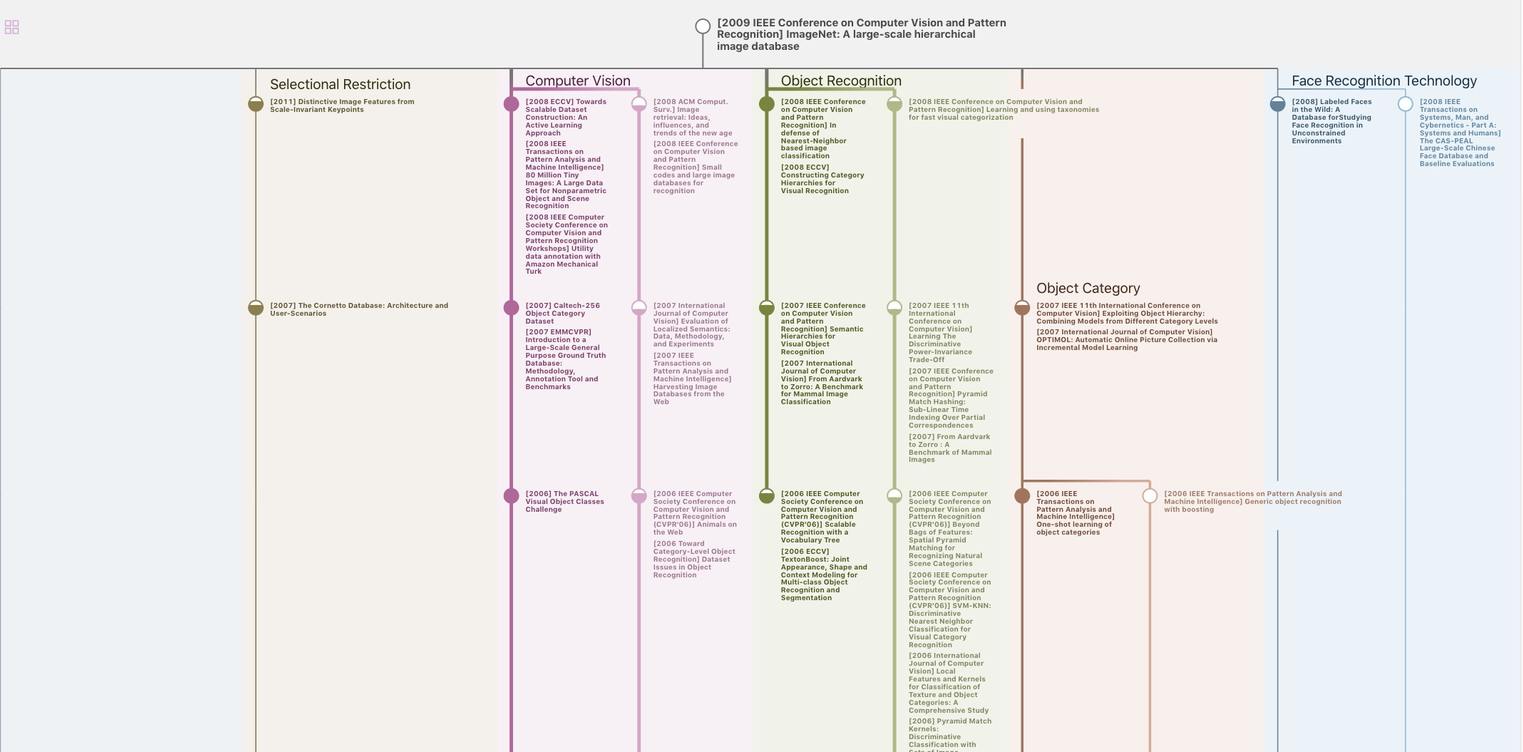
生成溯源树,研究论文发展脉络
Chat Paper
正在生成论文摘要