Denoising Simulated Low-Field MRI (70mT) using Denoising Autoencoders (DAE) and Cycle-Consistent Generative Adversarial Networks (Cycle-GAN)
CoRR(2023)
Abstract
In this work, a denoising Cycle-GAN (Cycle Consistent Generative Adversarial Network) is implemented to yield high-field, high resolution, high signal-to-noise ratio (SNR) Magnetic Resonance Imaging (MRI) images from simulated low-field, low resolution, low SNR MRI images. Resampling and additive Rician noise were used to simulate low-field MRI. Images were utilized to train a Denoising Autoencoder (DAE) and a Cycle-GAN, with paired and unpaired cases. Both networks were evaluated using SSIM and PSNR image quality metrics. This work demonstrates the use of a generative deep learning model that can outperform classical DAEs to improve low-field MRI images and does not require image pairs.
MoreTranslated text
Key words
denoising autoencoders
AI Read Science
Must-Reading Tree
Example
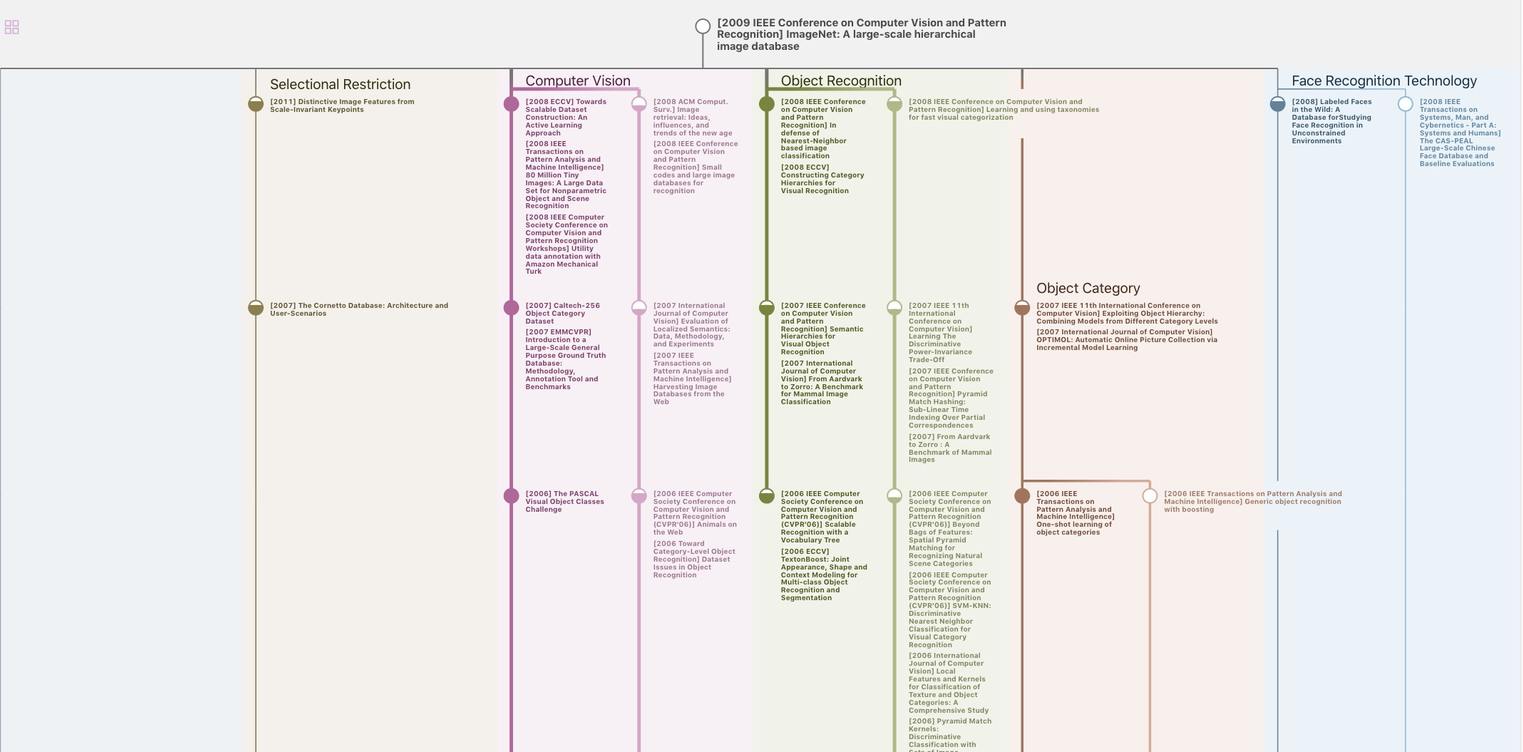
Generate MRT to find the research sequence of this paper
Chat Paper
Summary is being generated by the instructions you defined