Sparse representation for machine learning the properties of defects in 2D materials
npj Computational Materials(2023)
摘要
Two-dimensional materials offer a promising platform for the next generation of (opto-) electronic devices and other high technology applications. One of the most exciting characteristics of 2D crystals is the ability to tune their properties via controllable introduction of defects. However, the search space for such structures is enormous, and ab-initio computations prohibitively expensive. We propose a machine learning approach for rapid estimation of the properties of 2D material given the lattice structure and defect configuration. The method suggests a way to represent configuration of 2D materials with defects that allows a neural network to train quickly and accurately. We compare our methodology with the state-of-the-art approaches and demonstrate at least 3.7 times energy prediction error drop. Also, our approach is an order of magnitude more resource-efficient than its contenders both for the training and inference part.
更多查看译文
关键词
sparse representation,machine learning,defects,materials
AI 理解论文
溯源树
样例
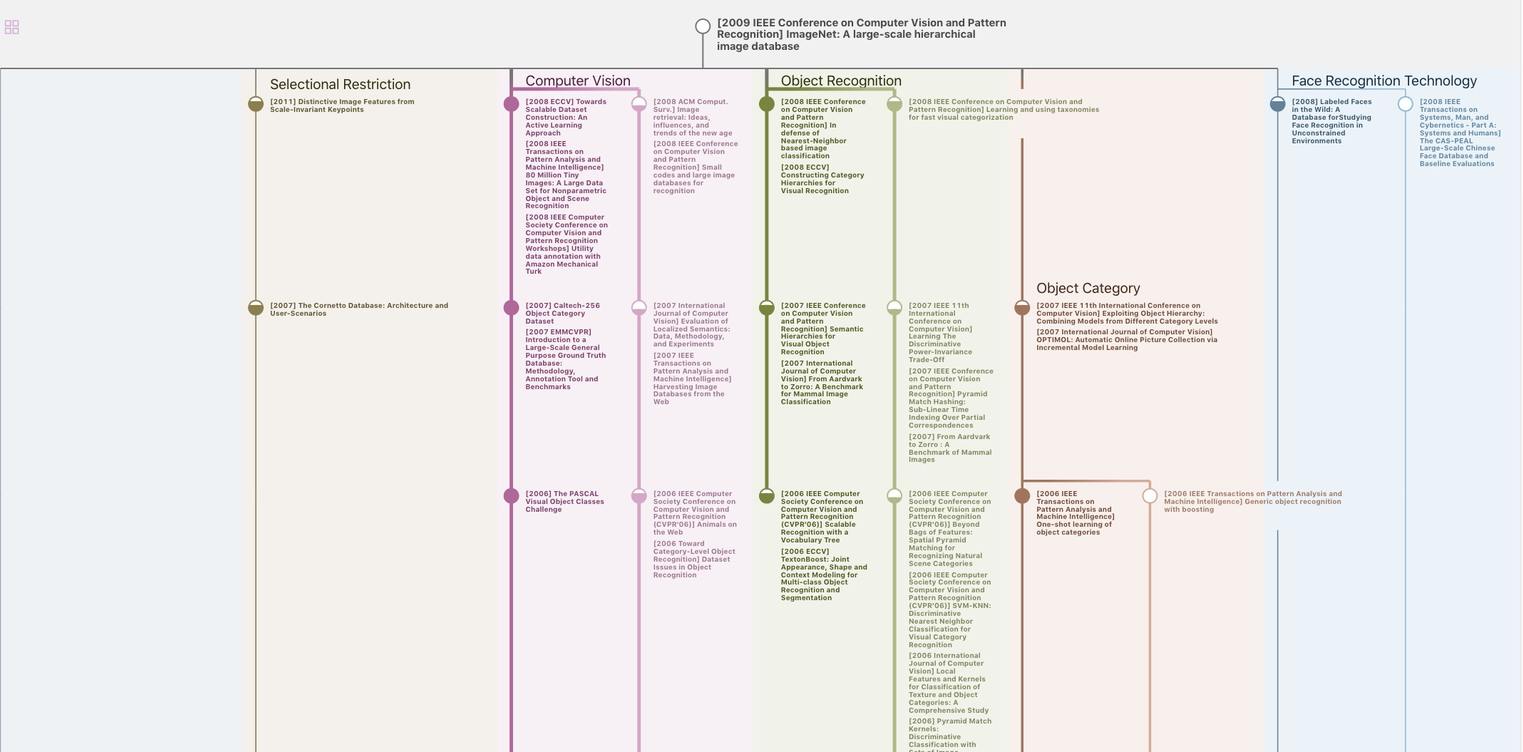
生成溯源树,研究论文发展脉络
Chat Paper
正在生成论文摘要