Parameter-free Spikelet: Discovering Different Length and Warped Time Series Motifs using an Adaptive Time Series Representation.
KDD(2023)
摘要
Over the last two decades, time series motif discovery has emerged as a useful primitive for many downstream analytical tasks, including clustering, classification, rule discovery, segmentation, and summarization. In parallel, it has long been known that Dynamic Time Warping (DTW) is superior to other similarity measures such as Euclidean Distance under most settings. Recently an algorithm to allow scalable DTW motif discovery was proposed; however, it is limited to finding pairs of subsequences whose subsequence lengths are the same. Moreover, that length must be provided by the user ahead of time. In this work, we propose a novel method to discover "warped" motifs whose lengths may differ. Moreover, our method allows input parameters that are not fixed lengths but rather just bounds on the maximum length of motifs to find. This allows us to quickly find different-length motifs without the burdensome trial-and-error of conventional methods. With extensive empirical work, we show that our method is scalable enough for real-world datasets and enables us to find variable-length and "warped" motifs that would otherwise escape the attention of conventional algorithms.
更多查看译文
关键词
Time series,Time series Motifs,Adaptive Representation
AI 理解论文
溯源树
样例
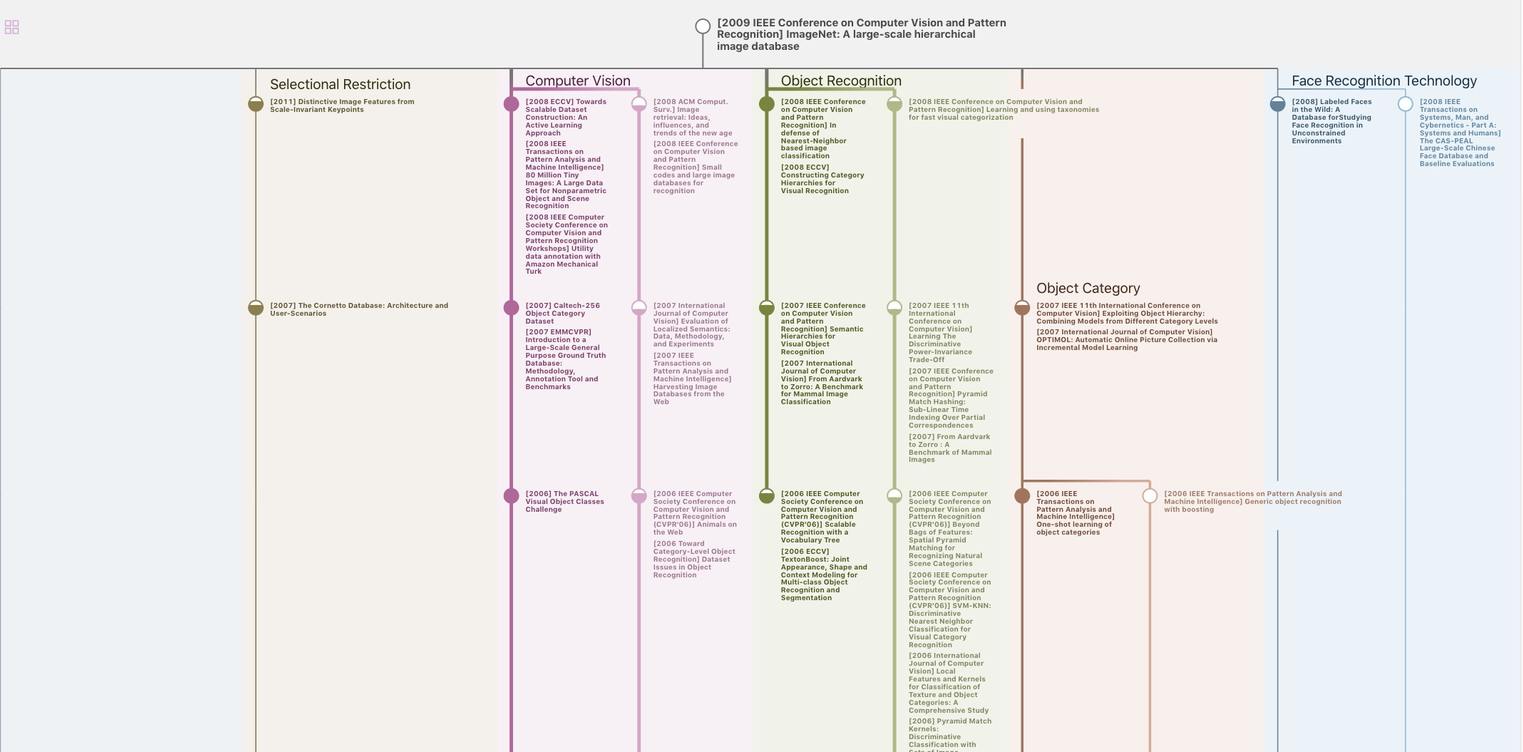
生成溯源树,研究论文发展脉络
Chat Paper
正在生成论文摘要