iETA: A Robust and Scalable Incremental Learning Framework for Time-of-Arrival Estimation.
KDD(2023)
摘要
Time-of-arrival estimation or Estimated Time of Arrival (ETA) has become an indispensable building block of modern intelligent transportation systems. While many efforts have been made for time-of-arrival estimation, most of them have scalability and robustness issues when dealing with real-world large-scale ETA scenarios, where billions of vehicle trajectories and ETA requests have been continuously generating every day. To this end, in this paper, we propose a robust and scalable incremental ETA learning framework, iETA, to continuously exploit spatio-temporal traffic patterns from massive floating-car data and thus achieve better estimation performances. Specifically, we first build an incremental travel time predictor that can be incrementally updated based on newly generated traffic data. The incremental travel time predictor not only reduces the overall learning overhead but also improves the model's robustness toward urban traffic distribution shifts. Then, we propose a historical traffic knowledge consolidation module to preserve critical spatio-temporal knowledge from previous ETA predictors under the incremental learning setting. Moreover, to reduce interference induced by low-quality traffic data, we propose an adversarial training module to improve the learning robustness by proactively mitigating and resisting traffic noise perturbations. Finally, extensive experiments demonstrate the effectiveness and efficiency of the proposed system against state-of-the-art baselines in large-scale ETA scenarios. Most importantly, iETA has been deployed on the Didi Chuxing platform, handling real-time billions of ETA queries every day, and substantially improves the prediction accuracy.
更多查看译文
关键词
Time-of-arrival estimation,traffic prediction,attention,knowledge distillation,adversarial training
AI 理解论文
溯源树
样例
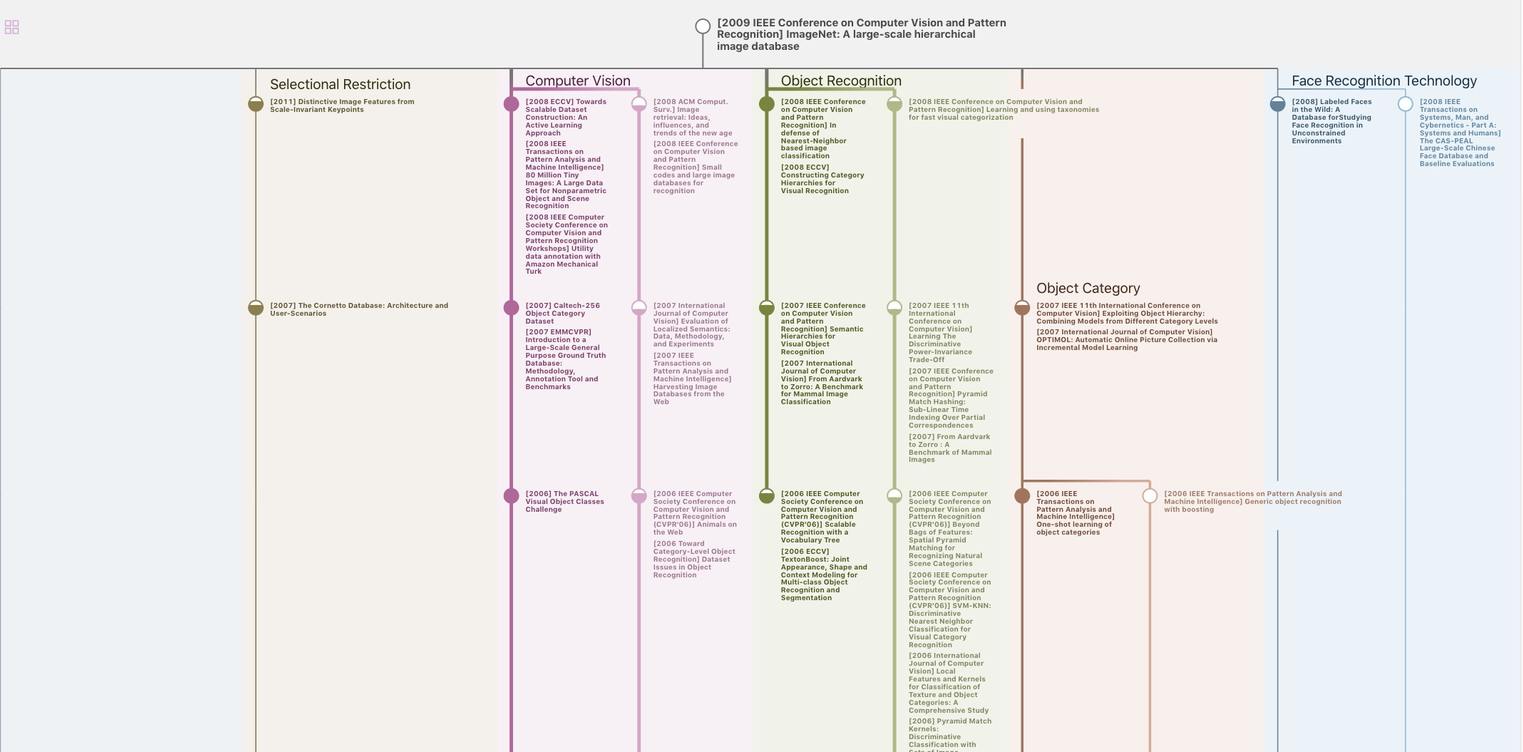
生成溯源树,研究论文发展脉络
Chat Paper
正在生成论文摘要