Online Few-Shot Time Series Classification for Aftershock Detection.
KDD(2023)
摘要
Seismic monitoring systems sift through seismograms in real-time, searching for target events, such as underground explosions. In this monitoring system, a burst of aftershocks (minor earthquakes occur after a major earthquake over days or even years) can be a source of confounding signals. Such a burst of aftershock signals can overload the human analysts of the monitoring system. To alleviate this burden at the onset of a sequence of events (e.g., aftershocks), a human analyst can label the first few of these events and start an online classifier to filter out subsequent aftershock events. We propose an online few-shot classification model FewSig for time series data for the above use case. The framework of FewSig consists of a selective model to identify the high-confidence positive events which are used for updating the models and a general classifier to label the remaining events. Our specific technique uses a selective model based on sliding DTWdistance and a general classifier model based on distance metric learning with Neighborhood Component Analysis (NCA). The algorithm demonstrates surprising robustness when tested on univariate datasets from the UEA/UCR archive. Furthermore, we show two real-world earthquake events where the FewSig reduces the human effort in monitoring applications by filtering out the aftershock events.
更多查看译文
关键词
Earthquake,Aftershock,Seismic Monitoring,Time Series Classification,Few-Shot Learning,Online Learning
AI 理解论文
溯源树
样例
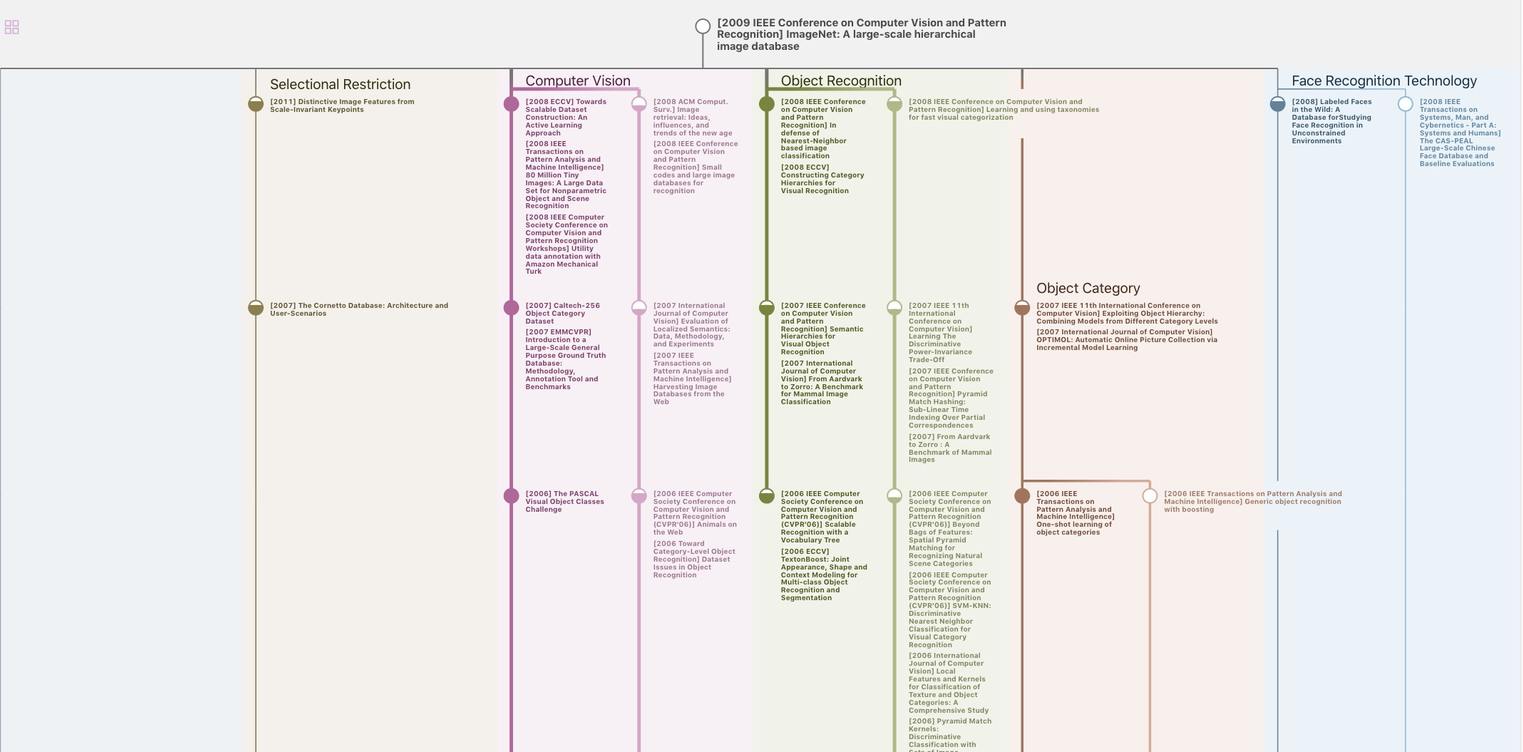
生成溯源树,研究论文发展脉络
Chat Paper
正在生成论文摘要