Domain-Specific Risk Minimization for Domain Generalization.
KDD(2023)
摘要
Domain generalization (DG) approaches typically use the hypothesis learned on source domains for inference on the unseen target domain. However, such a hypothesis can be arbitrarily far from the optimal one for the target domain, induced by a gap termed "adaptivity gap". Without exploiting the domain information from the unseen test samples, adaptivity gap estimation and minimization are intractable, which hinders us to robustify a model to any unknown distribution. In this paper, we first establish a generalization bound that explicitly considers the adaptivity gap. Our bound motivates two strategies to reduce the gap: the first one is ensembling multiple classifiers to enrich the hypothesis space, then we propose effective gap estimation methods for guiding the selection of a better hypothesis for the target. The other method is minimizing the gap directly by adapting model parameters using online target samples. We thus propose Domain-specific Risk Minimization (DRM). During training, DRM models the distributions of different source domains separately; for inference, DRM performs online model steering using the source hypothesis for each arriving target sample. Extensive experiments demonstrate the effectiveness of the proposed DRM for domain generalization. Code is available at: https://github.com/yfzhang114/AdaNPC.
更多查看译文
关键词
Domain Generalization,Test-time Adaptation,Adaptivity gap
AI 理解论文
溯源树
样例
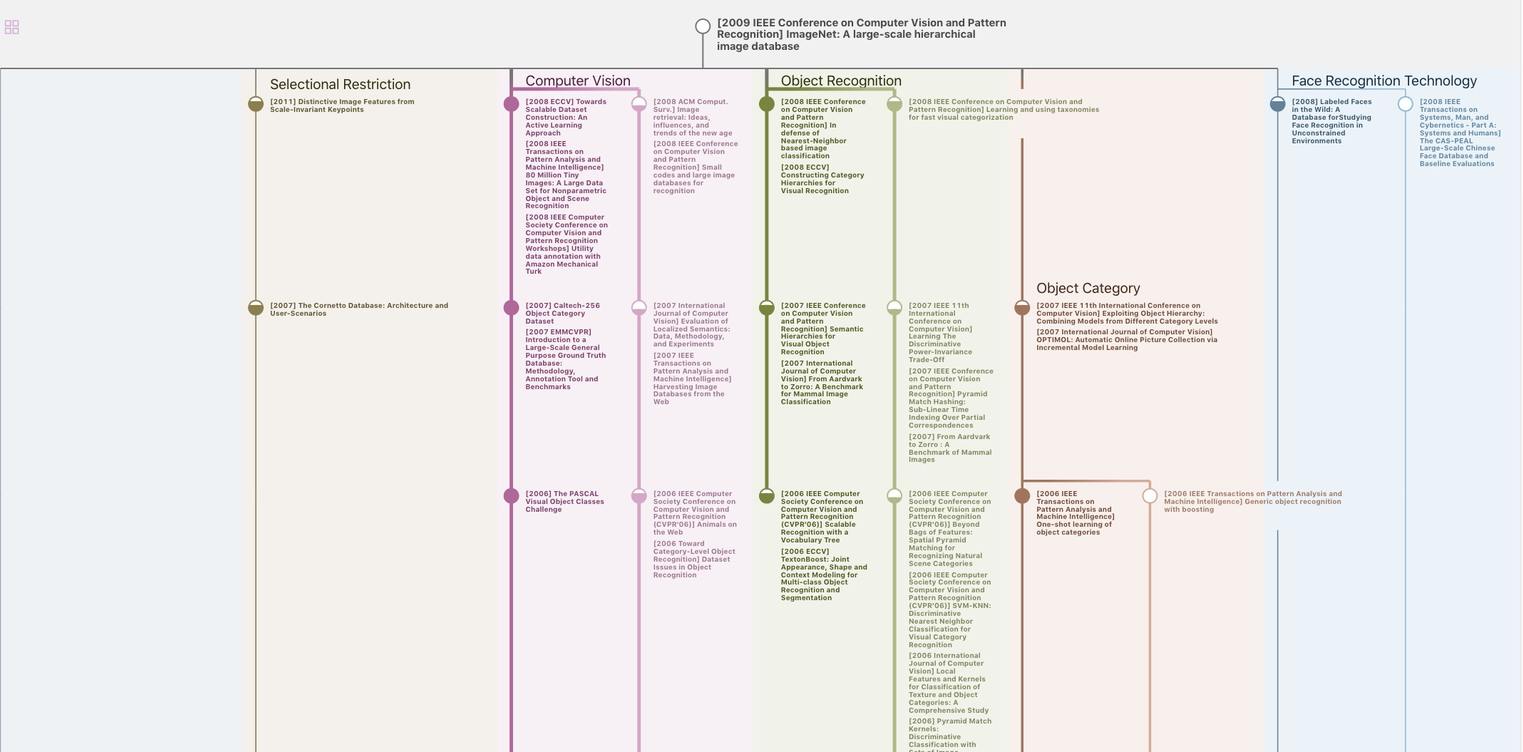
生成溯源树,研究论文发展脉络
Chat Paper
正在生成论文摘要