Entity-aware Multi-task Learning for Query Understanding at Walmart.
KDD(2023)
摘要
Query Understanding (QU) is a fundamental process in E-commerce search engines by extracting the shopping intents of customers. It usually includes a set of different tasks such as named entity recognization and query classification. Traditional approaches often tackle each task separately by its own network, which leads to excessive workload for development and maintenance as well as increased latency and resource usage in large-scale E-commerce platforms. To tackle these challenges, this paper presents a multitask learning approach to query understanding at Walmart. We experimented with several state-of-the-art multi-task learning architectures including MTDNN, MMoE, and PLE. Furthermore, we propose a novel large-scale entity-aware multi-task learning model (EAMT)1 by retrieving entities from engagement data as query context to augment the query representation. To the best of our knowledge, there exists no prior work on multi-task learning for E-commerce query understanding. Comprehensive offline experiments are conducted on industry-scale datasets (up to 965M queries) to illustrate the effectiveness of our approach. The results from online experiments show substantial gains in key accuracy and latency metrics.
更多查看译文
关键词
Multi-task learning,Semi-supervised learning,Query Understanding,E-commerce
AI 理解论文
溯源树
样例
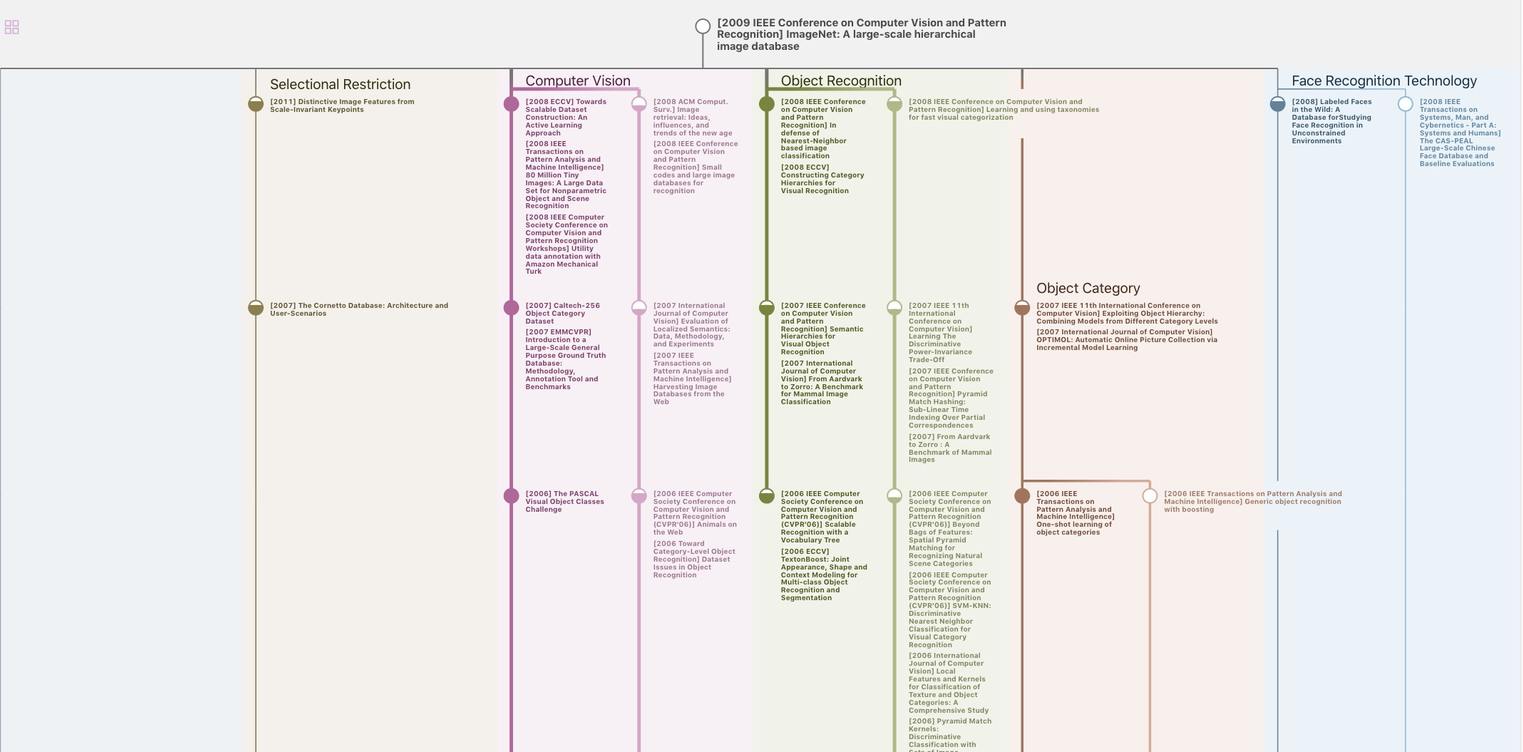
生成溯源树,研究论文发展脉络
Chat Paper
正在生成论文摘要