Sphere: Semi-Supervised Pre-training for Web Search over Heterogeneous Learning to Rank Data.
KDD 2023(2023)
摘要
While Learning to Rank (LTR) models on top of transformers have been widely adopted to achieve decent performance, it is still challenging to train the model with sufficient data as only an extremely small number of query-webpage pairs could be annotated versus trillions of webpages available online and billions of web search queries everyday. In the meanwhile, industry research communities have released a number of open-source LTR datasets with well annotations but incorporating different designs of LTR features/labels (i.e., heterogeneous domains). In this work, inspired by the recent progress in pre-training transformers for performance advantages, we study the problem of pre-training LTR models using both labeled and unlabeled samples, especially we focus on the use of well-annotated samples in heterogeneous open-source LTR datasets to boost the performance of pre-training. Hereby, we propose S 2 phere-Semi-Supervised Pre-training with Heterogeneous LTR data strategies for LTR models using both unlabeled and labeled query-webpage pairs across heterogeneous LTR datasets. S 2 phere consists of a three-step approach: (1) Semi-supervised Feature Extraction Pre-training via Perturbed Contrastive Loss, (2) Cross-domain Ranker Pre-training over Heterogeneous LTR Datasets and (3) End-to-end LTR Fine-tuning via Modular Network Composition. Specifically, given an LTR model composed of a backbone (the feature extractor), a neck (the module to reason the orders) and a head (the predictor of ranking scores), S 2 phere uses unlabeled/labeled data from the search engine to pre-train the backbone in Step (1) via semi-supervised learning; then Step (2) incorporates multiple open-source heterogeneous LTR datasets to improve pre-training of the neck module as shared parameters of cross-domain learning; and finally, S2phere in Step (3) composes the backbone and neck with a randomly-initialized head into a whole LTR model and fine-tunes the model using search engine data with various learning strategies. Extensive experiments have been done with both offline experiments and online A/B Test on top of Baidu search engine. The comparisons against numbers of baseline algorithms confirmed the advantages of S 2 phere in producing high-performance LTR models for web-scale search.
更多查看译文
AI 理解论文
溯源树
样例
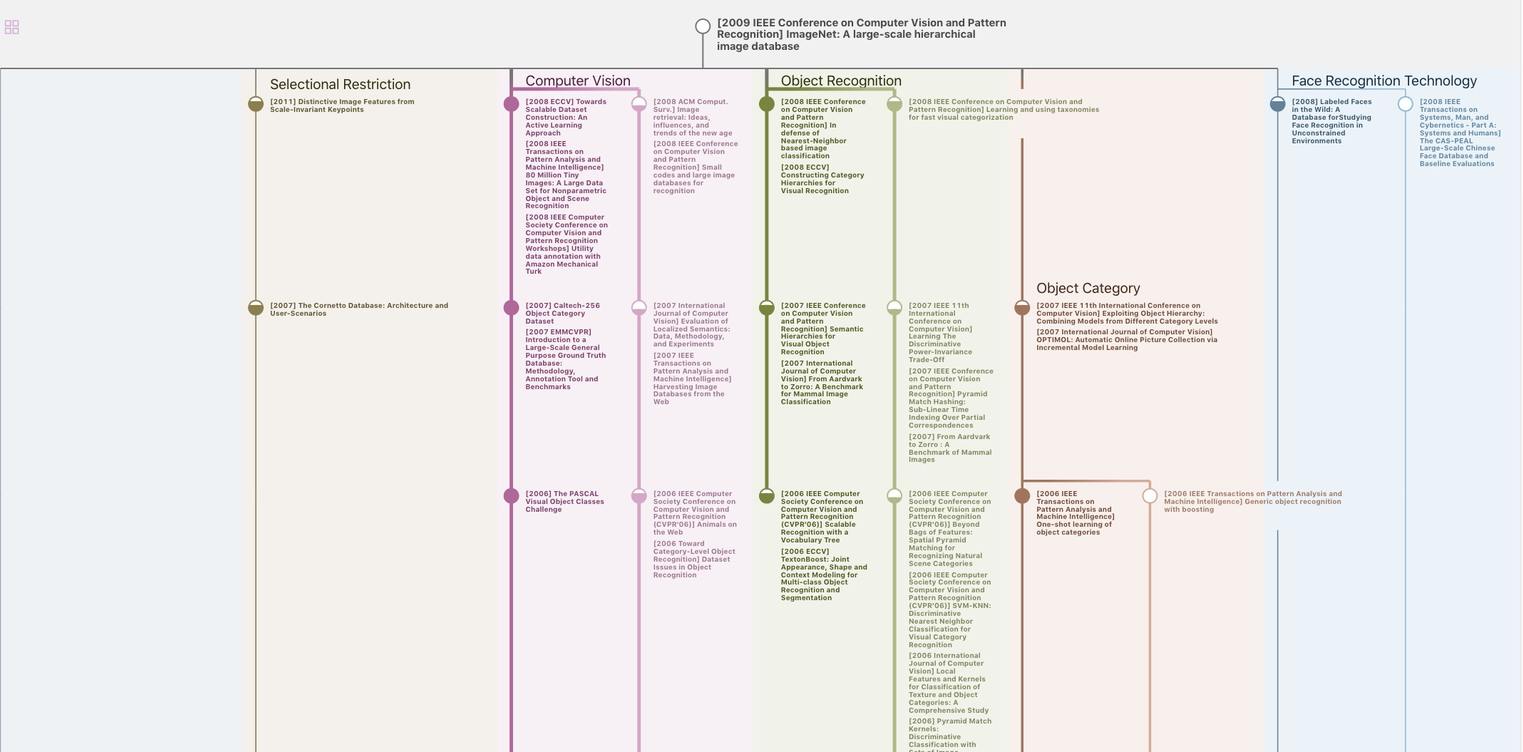
生成溯源树,研究论文发展脉络
Chat Paper
正在生成论文摘要