A Data-Driven Decision Support Framework for Player Churn Analysis in Online Games.
KDD(2023)
摘要
Faced with saturated market and fierce competition of online games, it is of great value to analyze the causes of the player churn for improving the game product, maintaining the player retention. Numerous research efforts on churn analysis have been made into churn prediction, which can achieve a sound accuracy benefiting from the booming of AI technologies. However, game publishers are usually unable to apply high-accuracy prediction methods in practice for preventing or relieving the churn due to the lack of the specific decision support (e.g., why they leave and what to do next). In this study, we fully exploit the expertise in online games and propose a comprehensive data-driven decision support framework for addressing game player churn. We first define the churn analysis in online games from a commercial perspective and elaborate the core demands of game publishers for churn analysis. Then we employ and improve the cutting-edge eXplainable AI (XAI) methods to predict player churn and analyze the potential churn causes. The possible churn causes can finally guide game publishers to make specific decisions of revision or intervention in our designed procedure. We demonstrate the effectiveness and high practical value of the framework by conducting extensive experiments on a real-world large-scale online game, Justice PC1. The whole decision support framework, bringing interesting and valuable insights, also receives positive reviews from the game product teams. Notably, the whole pipeline is readily transplanted to other online systems for decision support to address similar issues.
更多查看译文
关键词
Churn analysis,Explainable AI,Churn prediction,Anchor,TreeSHAP,A/B evaluation
AI 理解论文
溯源树
样例
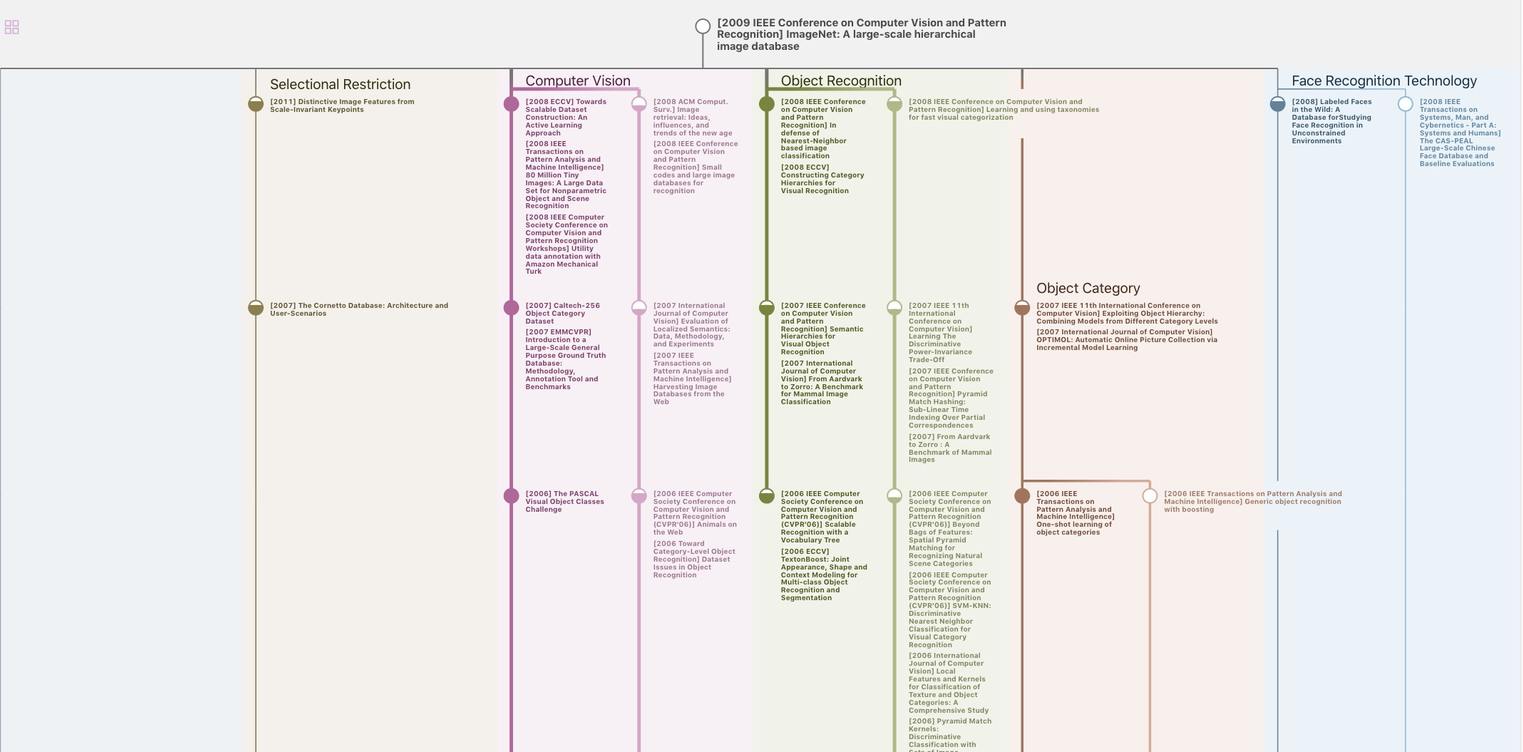
生成溯源树,研究论文发展脉络
Chat Paper
正在生成论文摘要