A Message Passing Neural Network Space for Better Capturing Data-dependent Receptive Fields
KDD 2023(2023)
摘要
Recently, the message passing neural network (MPNN) has attracted a lot of attention, which learns node representations based on the receptive field of the given node. Despite its success in many graph-related tasks, recent studies find that conventional MPNNs are incapable of handling variant receptive fields required in different graphs, and thereby some upgraded MPNNs have been developed. However, these methods are limited to designing a common solution for different graphs, which fails to capture the impact of different graph properties on the receptive fields. To alleviate such issues, we propose a novel MPNN space for data-dependent receptive fields (MpnnDRF), which enables us to dynamically design suitable MPNNs to capture the receptive field for the given graph. More concretely, we systemically investigate the capability of existing designs and propose several key design dimensions to improve them. Then, to fully explore the proposed designs and useful designs in existing works, we propose a novel search space to incorporate them and formulate a search framework. In the empirical study, the proposed MpnnDRF shows very strong robustness against the increased receptive field, which allows MpnnDRF to learn node representations based on a larger perceptual field. Therefore, MpnnDRF consistently achieves outstanding performance on benchmark node and graph classification tasks.
更多查看译文
AI 理解论文
溯源树
样例
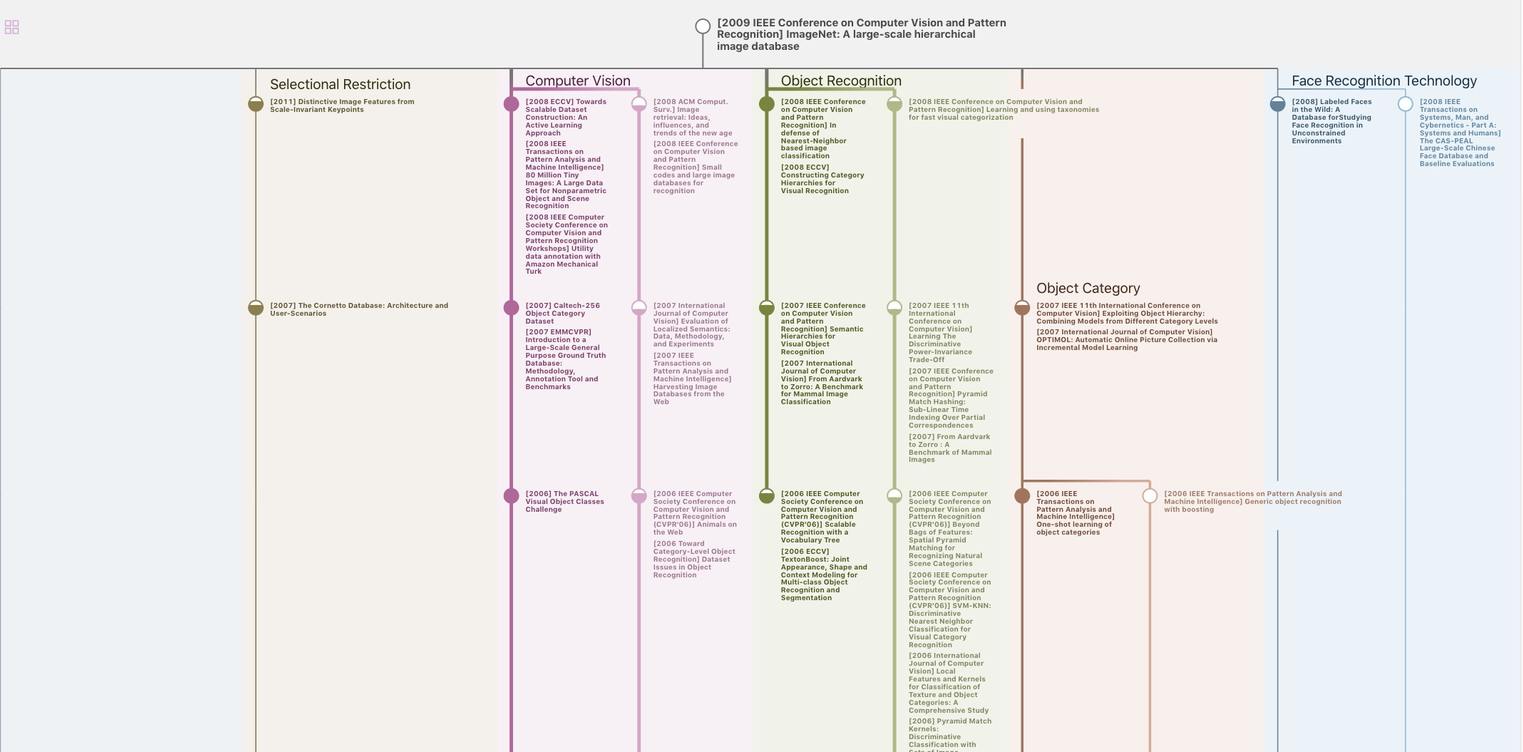
生成溯源树,研究论文发展脉络
Chat Paper
正在生成论文摘要