Learning to Discover Various Simpson's Paradoxes.
KDD(2023)
摘要
Simpson's paradox is a well-known statistical phenomenon that has captured the attention of statisticians, mathematicians, and philosophers for more than a century. The paradox often confuses people when it appears in data, and ignoring it may lead to incorrect decisions. Recent studies have found many examples of Simpson's paradox in social data and proposed a few methods to detect the paradox automatically. However, these methods suffer from many limitations, such as being only suitable for categorical variables or one specific paradox. To address these problems, we develop a learning-based approach to discover various Simpson's paradoxes. Firstly, we propose a framework from a statistical perspective that unifies multiple variants of Simpson's paradox currently known. Secondly, we present a novel loss function, Multi-group Pearson Correlation Coefficient (MPCC), to calculate the association strength of two variables of multiple subgroups. Then, we design a neural network model, coined SimNet, to automatically disaggregate data into multiple subgroups by optimizing the MPCC loss. Experiments on various datasets demonstrate that SimNet can discover various Simpson's paradoxes caused by discrete and continuous variables, even hidden variables. The code is available at https://github.com/ant-research/Learning-to-Discover-Various-Simpson-Paradoxes.
更多查看译文
关键词
Simpson's paradox,neural networks,data mining
AI 理解论文
溯源树
样例
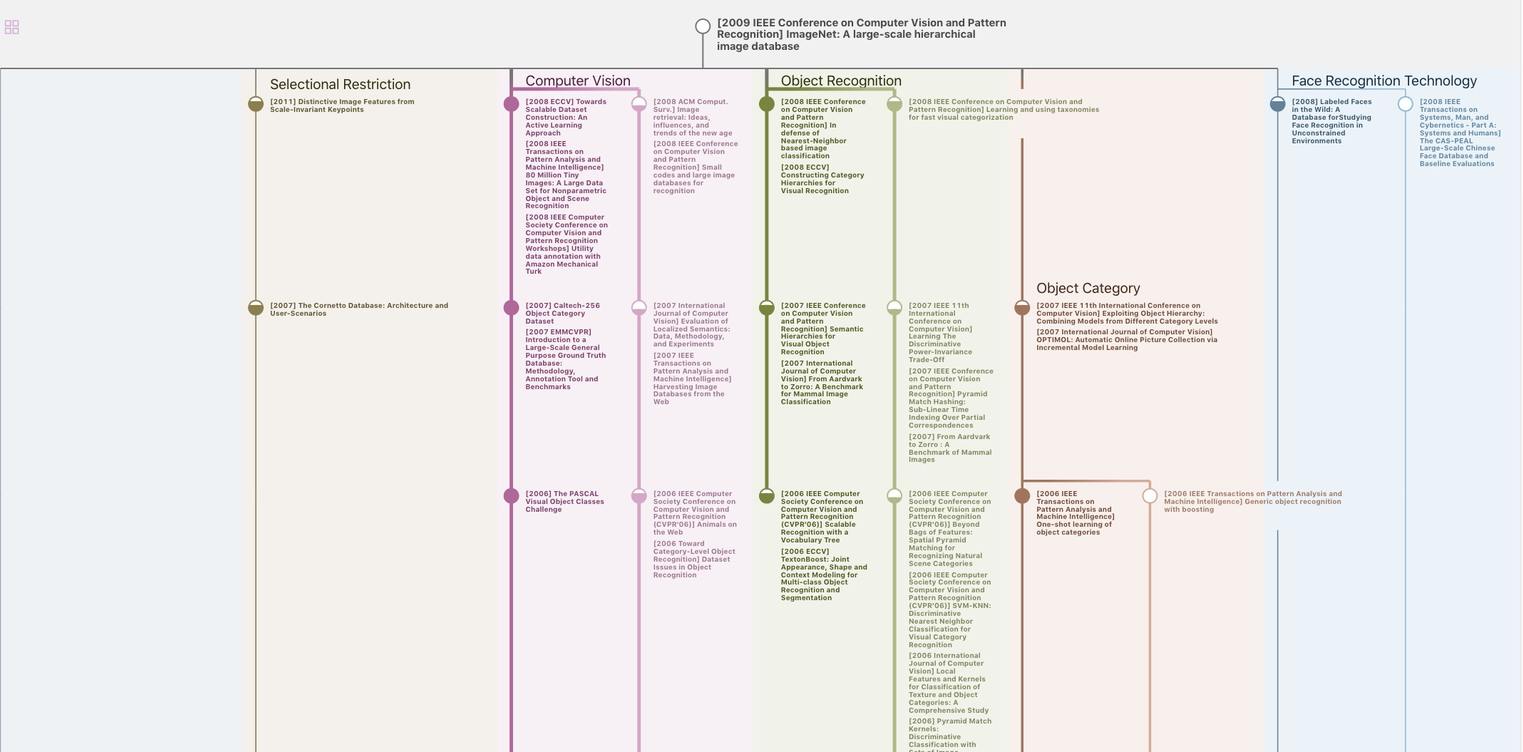
生成溯源树,研究论文发展脉络
Chat Paper
正在生成论文摘要