Learning Discrete Document Representations in Web Search.
KDD(2023)
摘要
Product quantization (PQ) has been usually applied to dense retrieval (DR) of documents thanks to its competitive time, memory efficiency and compatibility with other approximate nearest search (ANN) methods. Originally, PQ was learned to minimize the reconstruction loss, i.e., the distortions between the original dense embeddings and the reconstructed embeddings after quantization. Unfortunately, such an objective is inconsistent with the goal of selecting ground-truth documents for the input query, which may cause a severe loss of retrieval quality. Recent research has primarily concentrated on jointly training the biencoders and PQ to ensure consistency for improved performance. However, it is still difficult to design an approach that can cope with challenges like discrete representation collapse, mining informative negatives, and deploying effective embedding-based retrieval (EBR) systems in a real search engine. In this paper, we propose a Two-stage Multi-task Joint training technique (TMJ) to learn discrete document representations, which is simple and effective for real-world practical applications. In the first stage, the PQ centroid embeddings are regularized by the dense retrieval loss, which ensures the distinguishability of the quantized vectors and preserves the retrieval quality of dense embeddings. In the second stage, a PQ-oriented sample mining strategy is introduced to explore more informative negatives and further improve the performance. Offline evaluations are performed on a public benchmark (MS MARCO) and two private real-world web search datasets, where our method notably outperforms the SOTA PQ methods both in Recall and Mean Reciprocal Ranking (MRR). Besides, online experiments are conducted to validate that our technique can significantly provide high-quality vector quantization. Moreover, our joint training framework has been successfully applied to a billion-scale web search system.
更多查看译文
关键词
PQ,Joint Training,Web Search Engine,Negative Sample Mining
AI 理解论文
溯源树
样例
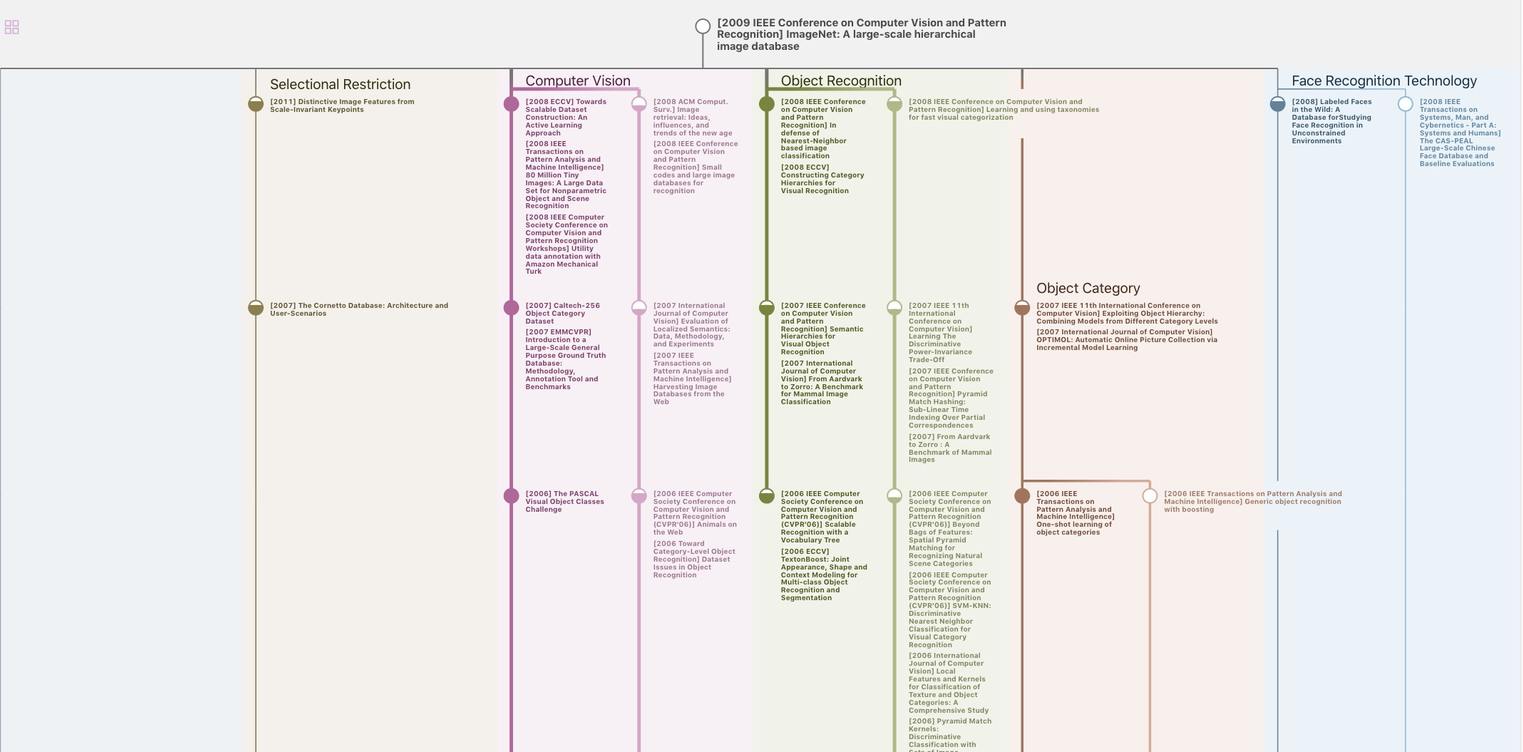
生成溯源树,研究论文发展脉络
Chat Paper
正在生成论文摘要