What's Behind the Mask: Understanding Masked Graph Modeling for Graph Autoencoders.
KDD(2023)
Abstract
The last years have witnessed the emergence of a promising self-supervised learning strategy, referred to as masked autoencoding. However, there is a lack of theoretical understanding of how masking matters on graph autoencoders (GAEs). In this work, we present masked graph autoencoder (MaskGAE), a self-supervised learning framework for graph-structured data. Different from standard GAEs, MaskGAE adopts masked graph modeling (MGM) as a principled pretext task - masking a portion of edges and attempting to reconstruct the missing part with partially visible, unmasked graph structure. To understand whether MGM can help GAEs learn better representations, we provide both theoretical and empirical evidence to comprehensively justify the benefits of this pretext task. Theoretically, we establish close connections between GAEs and contrastive learning, showing that MGM significantly improves the self-supervised learning scheme of GAEs. Empirically, we conduct extensive experiments on a variety of graph benchmarks, demonstrating the superiority of MaskGAE over several state-of-the-arts on both link prediction and node classification tasks.
MoreTranslated text
Key words
Graph Neural Networks,Graph Representation Learning,Graph Self-supervised Learning,Masked Graph Autoencoders
AI Read Science
Must-Reading Tree
Example
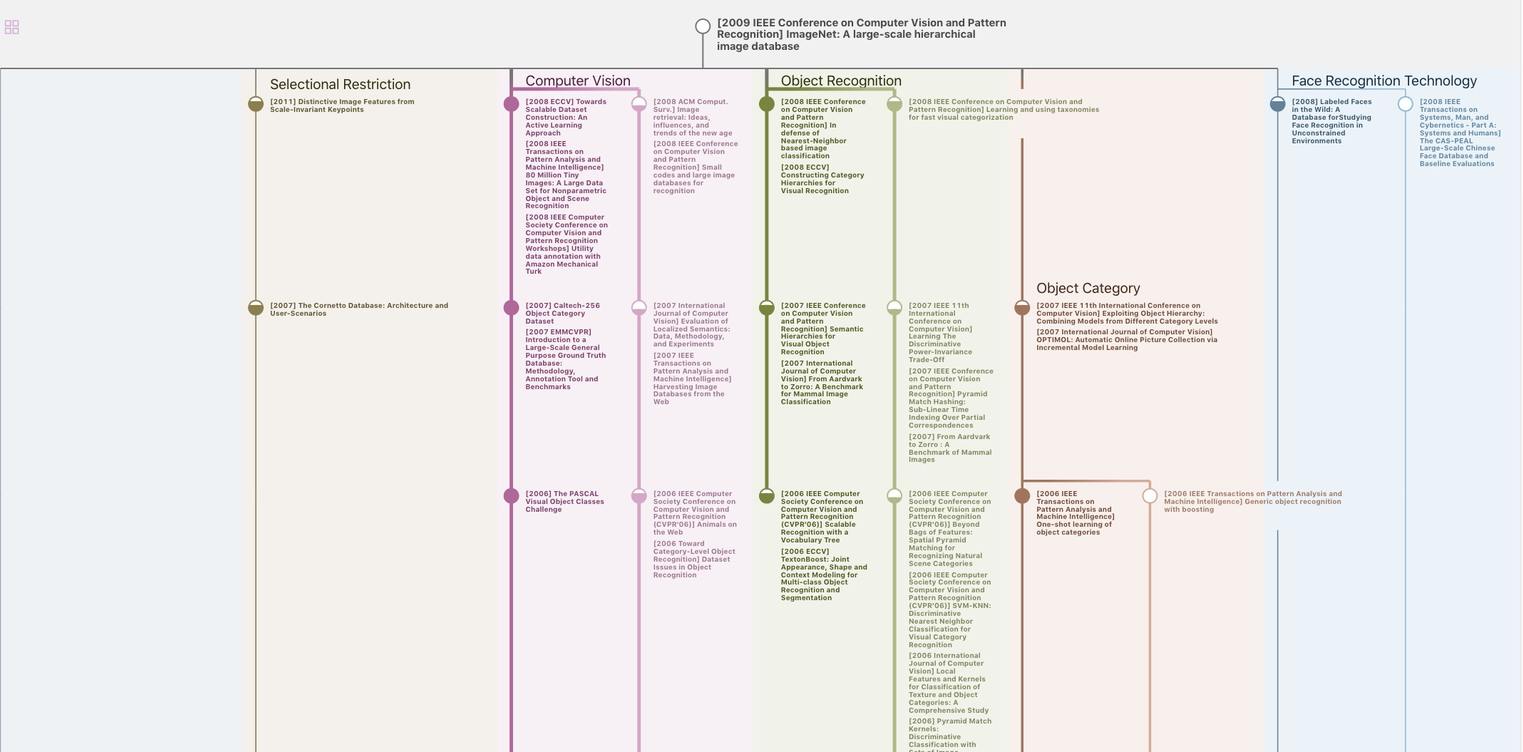
Generate MRT to find the research sequence of this paper
Chat Paper
Summary is being generated by the instructions you defined