Querywise Fair Learning to Rank through Multi-Objective Optimization.
KDD(2023)
摘要
In Learning-to-Rank (LTR) problems, the task of delivering relevant search results and allocating fair exposure to items of a protected group can conflict. Previous works in Fair LTR have attempted to resolve this by combining the objectives of relevant ranking and fair ranking into a single linear combination, but this approach is limited by the nonconvexity of the objective functions and can result in suboptimal relevance in ranking outputs. To address this, we propose a solution using Multi-Objective Optimization (MOO) algorithms. We extend these algorithms to querywise MOO to reduce the exposure disparity, not only on average but also at the query level. Interestingly, for moderate fairness requirements, it improves the relevance of ranking instead of deteriorating. We attribute this improvement to the benefits of multi-task learning and study the effect of fair ranking on the relevant ranking task. Moreover, we significantly improve the computational efficiency compared to previous methods by using the Gumbel max trick to sample the Plackett-Luce distribution. We evaluate our proposed methods on three real-world datasets and show their improvement in relevance ranking over state-of-the-art solutions.
更多查看译文
关键词
Learning to Rank,Fair Ranking,Multi-Objective Optimization
AI 理解论文
溯源树
样例
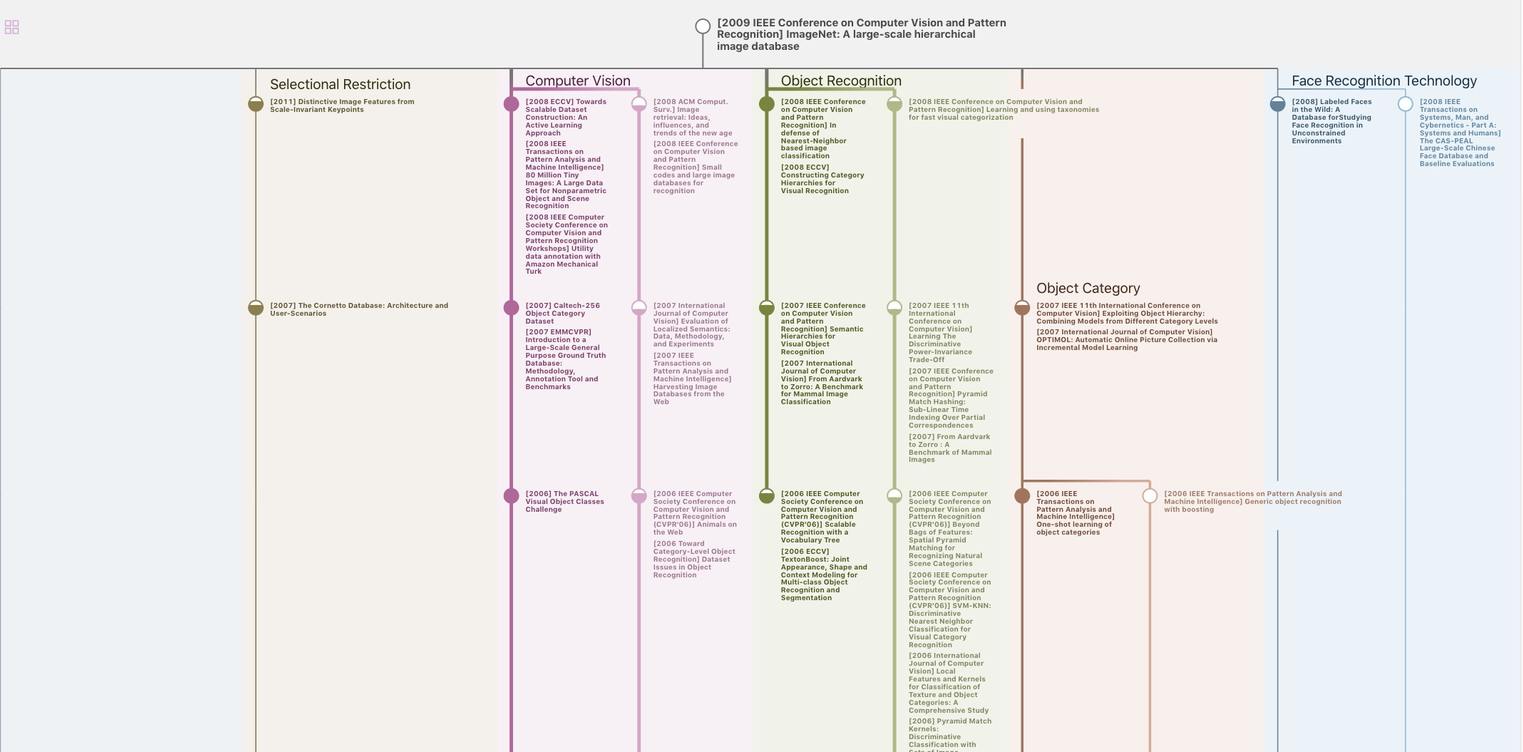
生成溯源树,研究论文发展脉络
Chat Paper
正在生成论文摘要