Physics-informed Machine Learning for Calibrating Macroscopic Traffic Flow Models
CoRR(2023)
摘要
Well-calibrated traffic flow models are fundamental to understanding traffic phenomena and designing control strategies. Traditional calibration has been developed base on optimization methods. In this paper, we propose a novel physics-informed, learning-based calibration approach that achieves performances comparable to and even better than those of optimization-based methods. To this end, we combine the classical deep autoencoder, an unsupervised machine learning model consisting of one encoder and one decoder, with traffic flow models. Our approach informs the decoder of the physical traffic flow models and thus induces the encoder to yield reasonable traffic parameters given flow and speed measurements. We also introduce the denoising autoencoder into our method so that it can handles not only with normal data but also with corrupted data with missing values. We verified our approach with a case study of I-210 E in California.
更多查看译文
关键词
calibrating macroscopic traffic
AI 理解论文
溯源树
样例
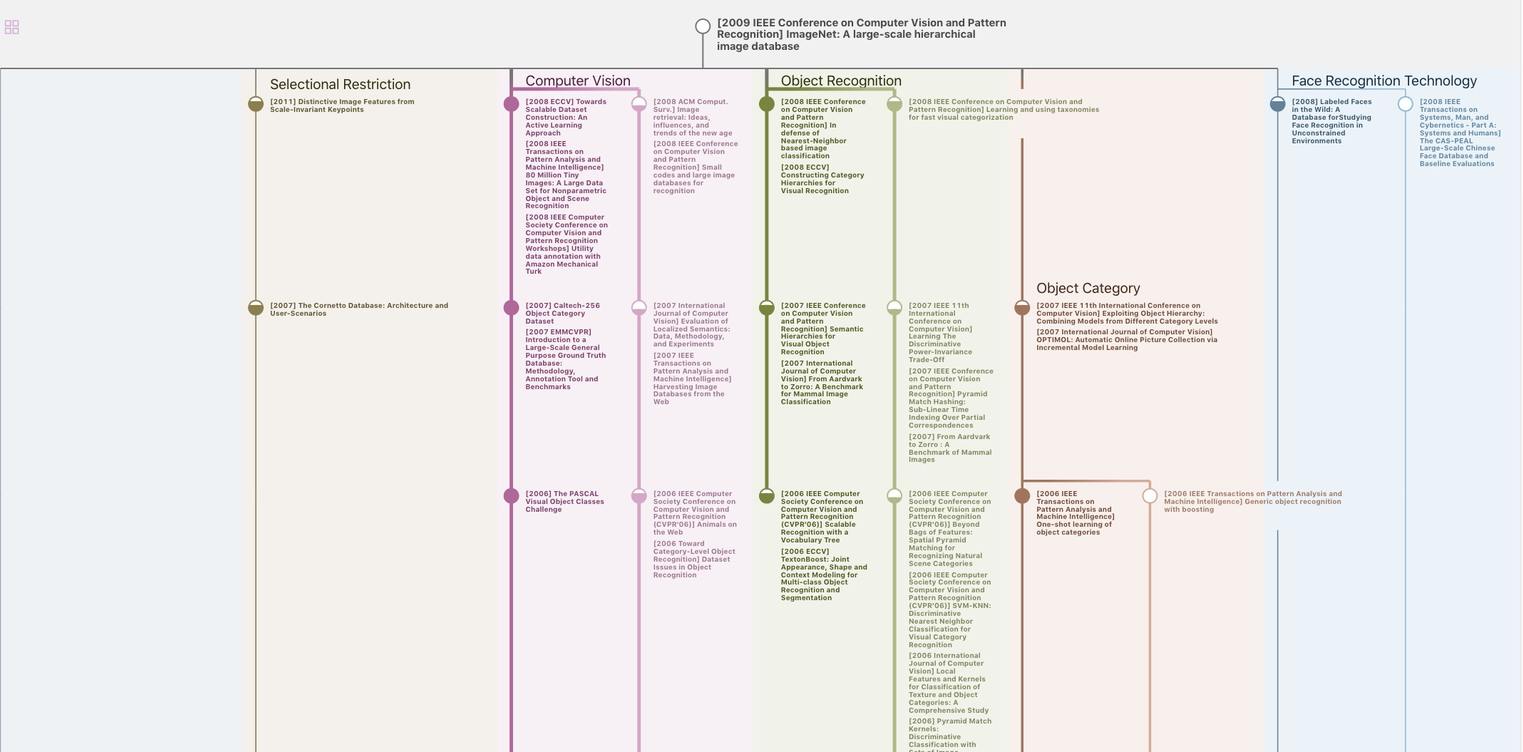
生成溯源树,研究论文发展脉络
Chat Paper
正在生成论文摘要