On the Design of Nonlinear MPC and LPVMPC for Obstacle Avoidance in Autonomous Driving
CoRR(2023)
摘要
In this study, we are concerned with autonomous driving missions when a static obstacle blocks a given reference trajectory. To provide a realistic control design, we employ a model predictive control (MPC) utilizing nonlinear state-space dynamic models of a car with linear tire forces, allowing for optimal path planning and tracking to overtake the obstacle. We provide solutions with two different methodologies. Firstly, we solve a nonlinear MPC (NMPC) problem with a nonlinear optimization framework, capable of considering the nonlinear constraints. Secondly, by introducing scheduling signals, we embed the nonlinear dynamics in a linear parameter varying (LPV) representation with adaptive linear constraints for realizing the nonlinear constraints associated with the obstacle. Consequently, an LPVMPC optimization problem can be solved efficiently as a quadratic programming (QP) that constitutes the main novelty of this work. We test the two methods for a challenging obstacle avoidance task and provide qualitative comparisons. The LPVMPC shows a significant reduction in terms of the computational burden at the expense of a slight loss of performance.
更多查看译文
关键词
adaptive linear constraints,autonomous driving missions,challenging obstacle avoidance task,given reference trajectory,linear parameter,linear tire forces,LPVMPC optimization problem,model predictive control,nonlinear constraints,nonlinear dynamics,nonlinear MPC problem,nonlinear optimization framework,nonlinear state-space dynamic models,optimal path planning,realistic control design,static obstacle blocks,tracking
AI 理解论文
溯源树
样例
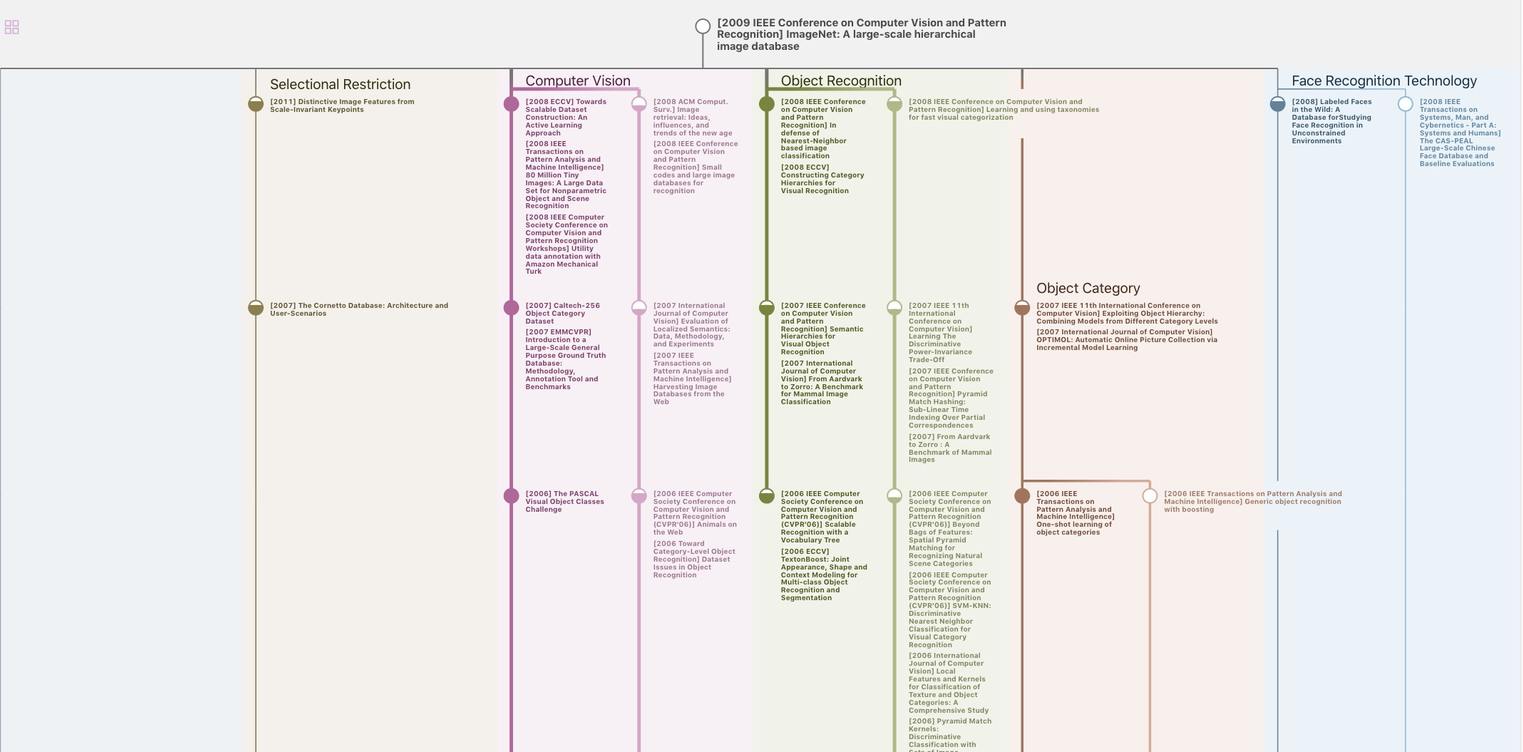
生成溯源树,研究论文发展脉络
Chat Paper
正在生成论文摘要