Deep Unrolling for Nonconvex Robust Principal Component Analysis
CoRR(2023)
摘要
We design algorithms for Robust Principal Component Analysis (RPCA) which consists in decomposing a matrix into the sum of a low rank matrix and a sparse matrix. We propose a deep unrolled algorithm based on an accelerated alternating projection algorithm which aims to solve RPCA in its nonconvex form. The proposed procedure combines benefits of deep neural networks and the interpretability of the original algorithm and it automatically learns hyperparameters. We demonstrate the unrolled algorithm's effectiveness on synthetic datasets and also on a face modeling problem, where it leads to both better numerical and visual performances.
更多查看译文
关键词
RPCA,Sparsity,low-rank,unrolled algorithm,hyperparameters
AI 理解论文
溯源树
样例
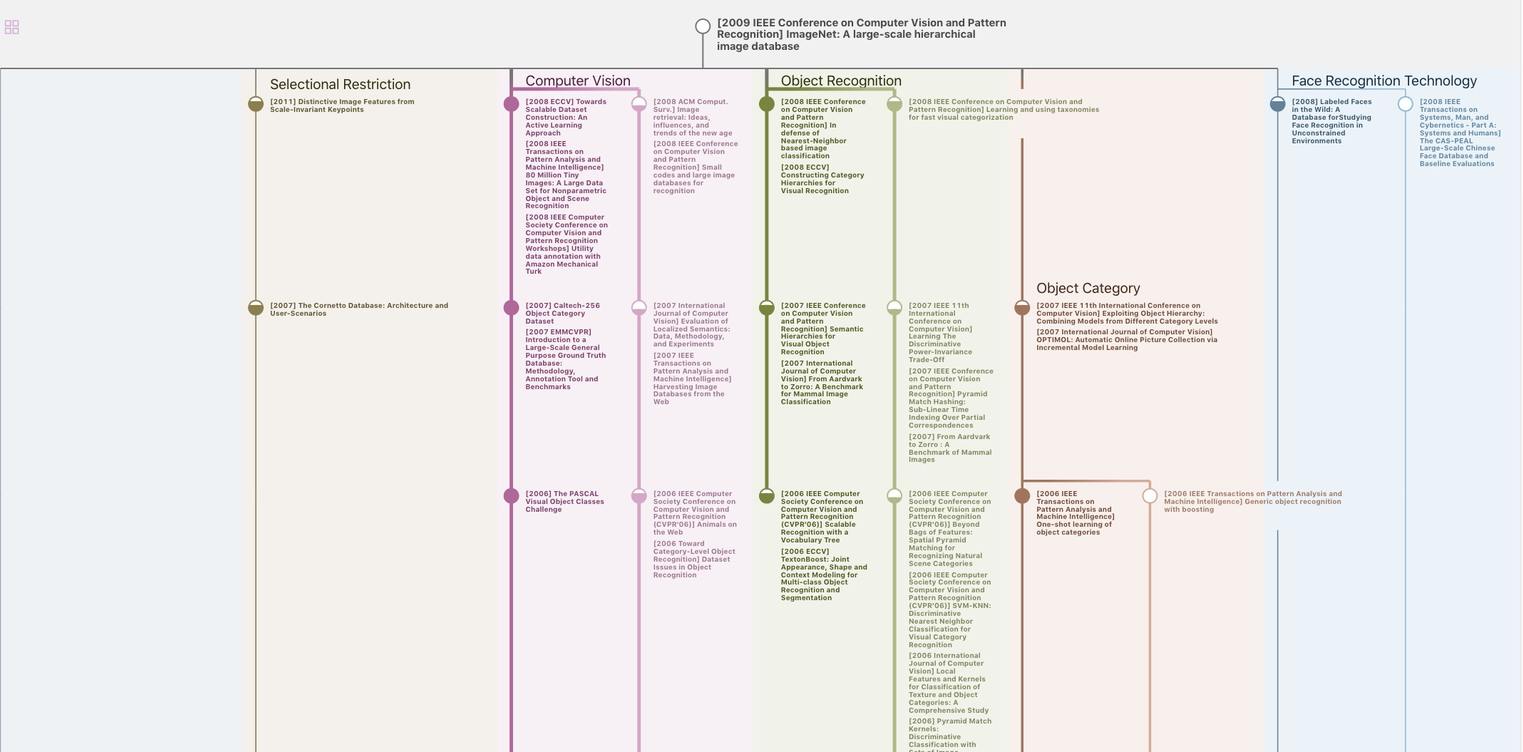
生成溯源树,研究论文发展脉络
Chat Paper
正在生成论文摘要