Assessing the calibration of multivariate probabilistic forecasts
QUARTERLY JOURNAL OF THE ROYAL METEOROLOGICAL SOCIETY(2024)
摘要
Rank and probability integral transform histograms are established tools to assess the calibration of probabilistic forecasts. They not only check whether a forecast is calibrated, but they also reveal what systematic biases (if any) are present in the forecasts. Several extensions of rank histograms have been proposed to evaluate the calibration of probabilistic forecasts for multivariate outcomes. These extensions introduce a so-called pre-rank function that condenses the multivariate forecasts and observations into univariate objects, from which a standard rank histogram can be produced. Existing pre-rank functions typically aim to preserve as much information as possible when condensing the multivariate forecasts and observations into univariate objects. Although this is sensible when conducting statistical tests for multivariate calibration, it can hinder the interpretation of the resulting histograms. In this article, we demonstrate that there are few restrictions on the choice of pre-rank function, meaning forecasters can choose a pre-rank function depending on what information they want to extract concerning forecast performance. We introduce the concept of simple pre-rank functions and provide examples that can be used to assess the mean, spread, and dependence structure of multivariate probabilistic forecasts, as well as pre-rank functions that could be useful when evaluating probabilistic spatial field forecasts. The simple pre-rank functions that we introduce are easy to interpret, easy to implement, and they deliberately provide complementary information, meaning several pre-rank functions can be employed to achieve a more complete understanding of multivariate forecast performance. We then discuss how e-values can be employed to formally test for multivariate calibration over time. This is demonstrated in an application to wind-speed forecasting using the EUPPBench post-processing benchmark dataset. The calibration of multivariate probabilistic forecasts can be assessed by transforming the forecasts and observations to univariate values, from which a rank histogram can be constructed. There are very few restrictions on the choice of transformation, meaning forecasters can choose a transformation depending on what information they want to extract concerning forecast performance. Several transformations are introduced that are applicable in a range of forecasting settings, and it is shown how these transformations provide useful information regarding multivariate forecast performance in both a simulation study and an application to gridded wind-speed forecasts. image
更多查看译文
关键词
ensembles,forecasting (methods),statistical methods
AI 理解论文
溯源树
样例
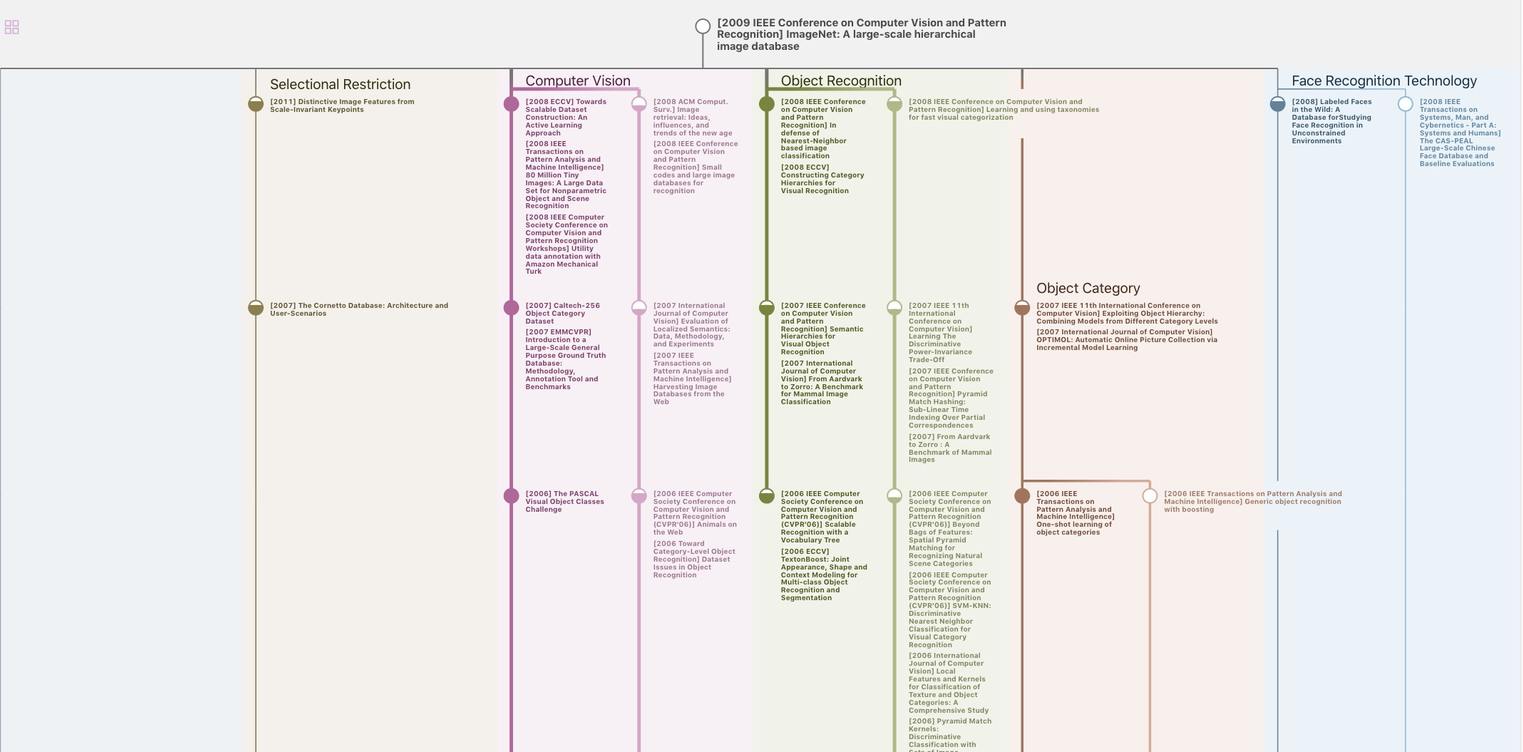
生成溯源树,研究论文发展脉络
Chat Paper
正在生成论文摘要