A Causal Ordering Prior for Unsupervised Representation Learning
CoRR(2023)
摘要
Unsupervised representation learning with variational inference relies heavily on independence assumptions over latent variables. Causal representation learning (CRL), however, argues that factors of variation in a dataset are, in fact, causally related. Allowing latent variables to be correlated, as a consequence of causal relationships, is more realistic and generalisable. So far, provably identifiable methods rely on: auxiliary information, weak labels, and interventional or even counterfactual data. Inspired by causal discovery with functional causal models, we propose a fully unsupervised representation learning method that considers a data generation process with a latent additive noise model (ANM). We encourage the latent space to follow a causal ordering via loss function based on the Hessian of the latent distribution.
更多查看译文
关键词
unsupervised representation learning,causal ordering prior
AI 理解论文
溯源树
样例
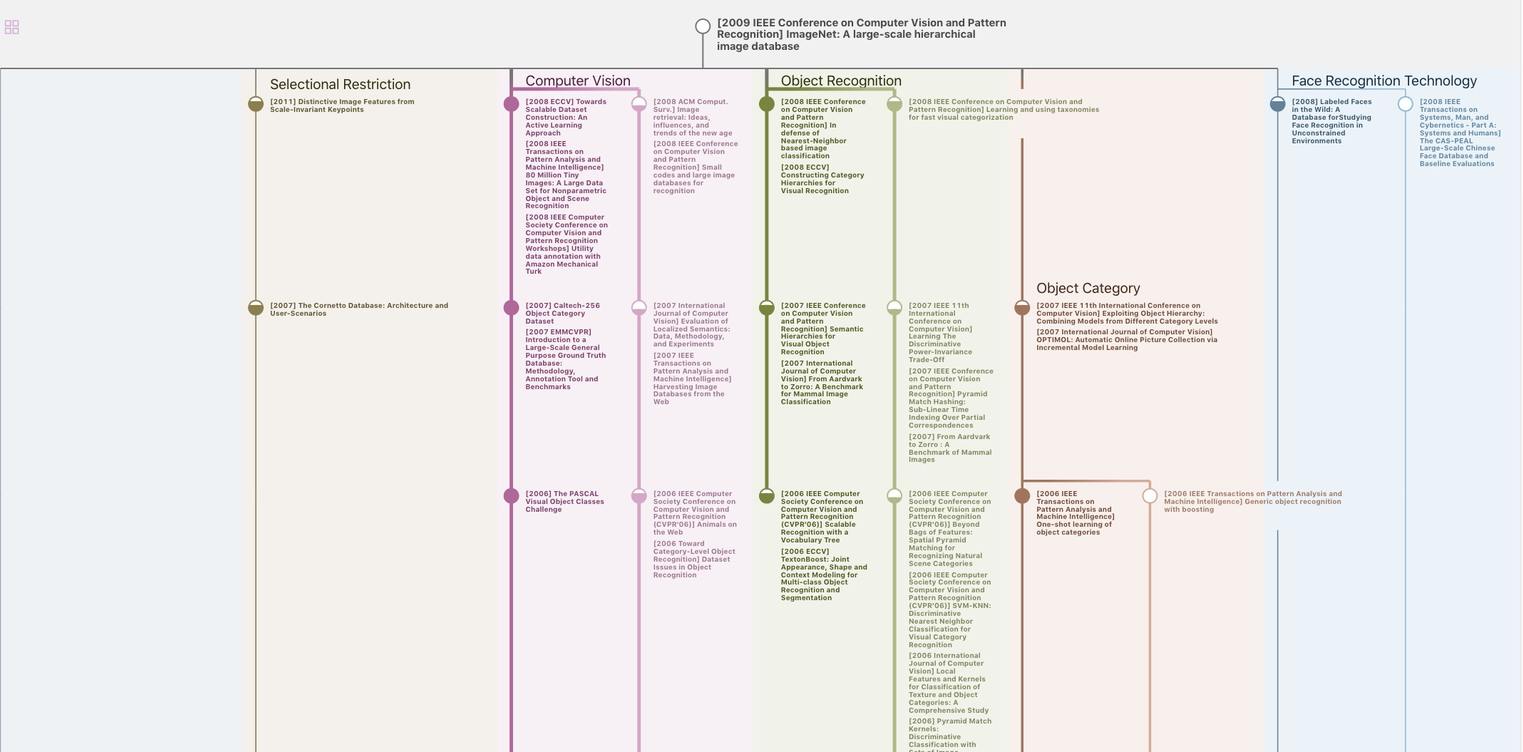
生成溯源树,研究论文发展脉络
Chat Paper
正在生成论文摘要