Procedurally generating rules to adapt difficulty for narrative puzzle games
CoRR(2023)
摘要
This paper focuses on procedurally generating rules and communicating them to players to adjust the difficulty. This is part of a larger project to collect and adapt games in educational games for young children using a digital puzzle game designed for kindergarten. A genetic algorithm is used together with a difficulty measure to find a target number of solution sets and a large language model is used to communicate the rules in a narrative context. During testing the approach was able to find rules that approximate any given target difficulty within two dozen generations on average. The approach was combined with a large language model to create a narrative puzzle game where players have to host a dinner for animals that can’t get along. Future experiments will try to improve evaluation, specialize the language model on children’s literature, and collect multi-modal data from players to guide adaptation.
更多查看译文
关键词
procedural content generation,large language models,genetic algorithm,learning environment,early childhood education
AI 理解论文
溯源树
样例
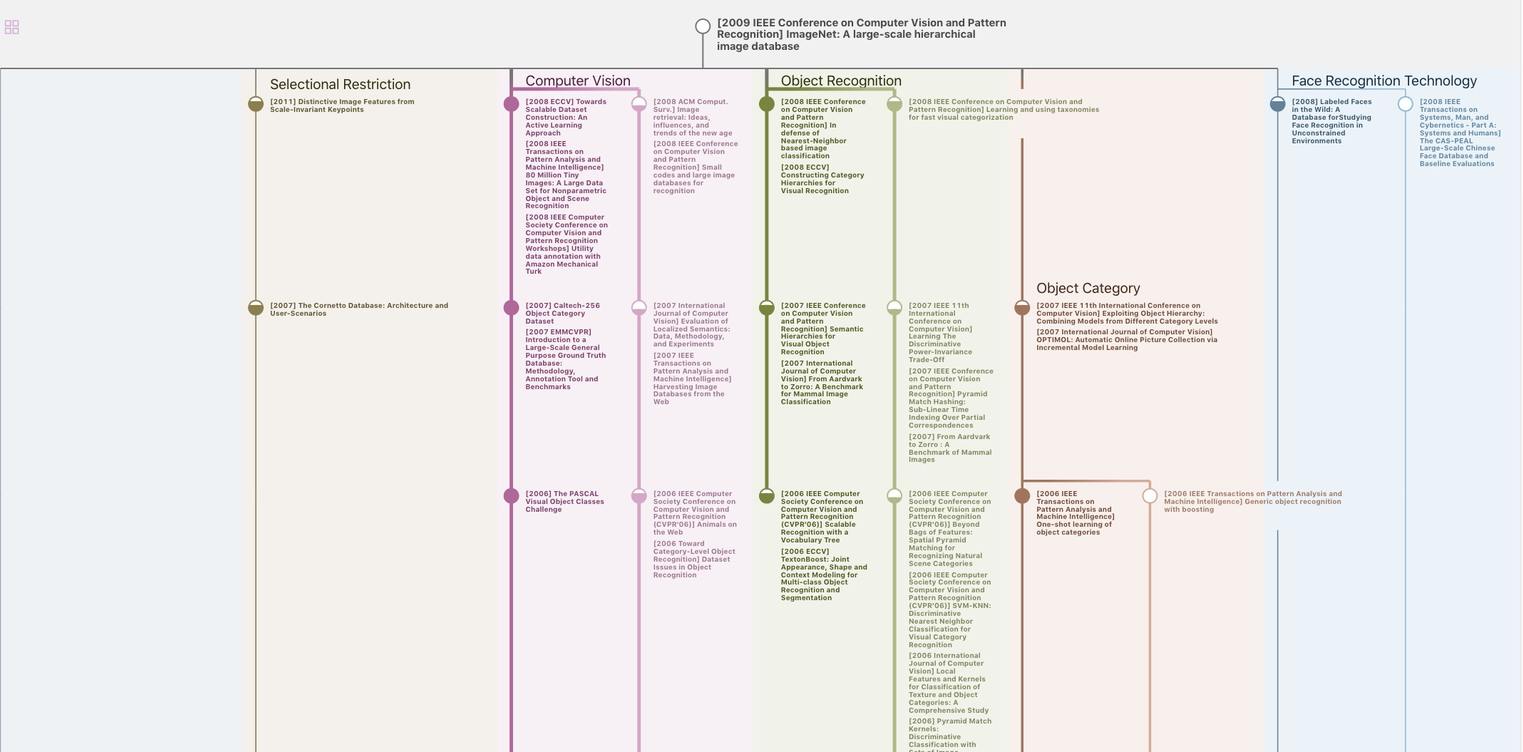
生成溯源树,研究论文发展脉络
Chat Paper
正在生成论文摘要