Uncertainty-Adjusted Recommendation via Matrix Factorization With Weighted Losses.
IEEE transactions on neural networks and learning systems(2023)
Abstract
In a recommender systems (RSs) dataset, observed ratings are subject to unequal amounts of noise. Some users might be consistently more conscientious in choosing the ratings they provide for the content they consume. Some items may be very divisive and elicit highly noisy reviews. In this article, we perform a nuclear-norm-based matrix factorization method which relies on side information in the form of an estimate of the uncertainty of each rating. A rating with a higher uncertainty is considered more likely to be erroneous or subject to large amounts of noise, and therefore more likely to mislead the model. Our uncertainty estimate is used as a weighting factor in the loss we optimize. To maintain the favorable scaling and theoretical guarantees coming with nuclear norm regularization even in this weighted context, we introduce an adjusted version of the trace norm regularizer which takes the weights into account. This regularization strategy is inspired from the weighted trace norm which was introduced to tackle nonuniform sampling regimes in matrix completion. Our method exhibits state-of-the-art performance on both synthetic and real life datasets in terms of various performance measures, confirming that we have successfully used the auxiliary information extracted.
MoreTranslated text
Key words
Matrix completion,recommender systems (RSs),uncertainty estimation,uncertainty-aware learning
AI Read Science
Must-Reading Tree
Example
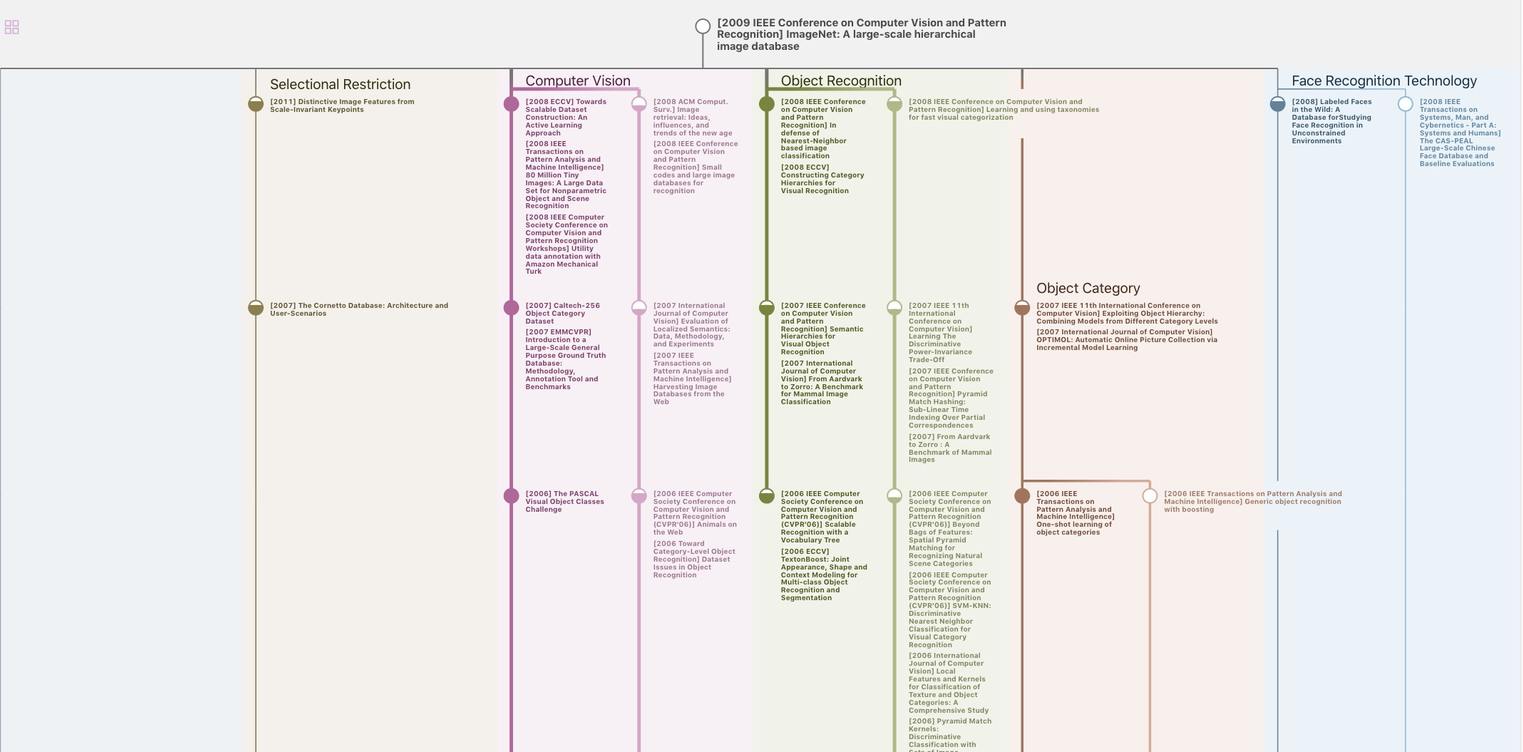
Generate MRT to find the research sequence of this paper
Chat Paper
Summary is being generated by the instructions you defined