Multi-Label Classification With Dual Tail-Node Augmentation for Drug Repositioning.
IEEE/ACM transactions on computational biology and bioinformatics(2023)
摘要
Due to the lengthy and costly process of new drug discovery, increasing attention has been paid to drug repositioning, i.e., identifying new drug-disease associations. Current machine learning methods for drug repositioning mainly leverage matrix factorization or graph neural networks, and have achieved impressive performance. However, they often suffer from insufficient training labels of inter-domain associations, while ignore the intra-domain associations. Moreover, they often neglect the importance of tail nodes that have few known associations, which limits their effectiveness in drug repositioning. In this paper, we propose a novel multi-label classification model with dual Tail-Node Augmentation for Drug Repositioning (TNA-DR). We incorporate disease-disease similarity and drug-drug similarity information into k-nearest neighbor ( kNN) augmentation module and contrastive augmentation module, respectively, which effectively complements the weak supervision of drug-disease associations. Furthermore, before employing the two augmentation modules, we filter the nodes by their degrees, so that the two modules are only applied to tail nodes. We conduct 10-fold cross validation experiments on four different real-world datasets, and our model achieves the state-of-the-art performance on all the four datasets. We also demonstrate our model's capability of identifying drug candidates for new diseases and discovering potential new links between existing drugs and diseases.
更多查看译文
关键词
classification,drug,multi-label,tail-node
AI 理解论文
溯源树
样例
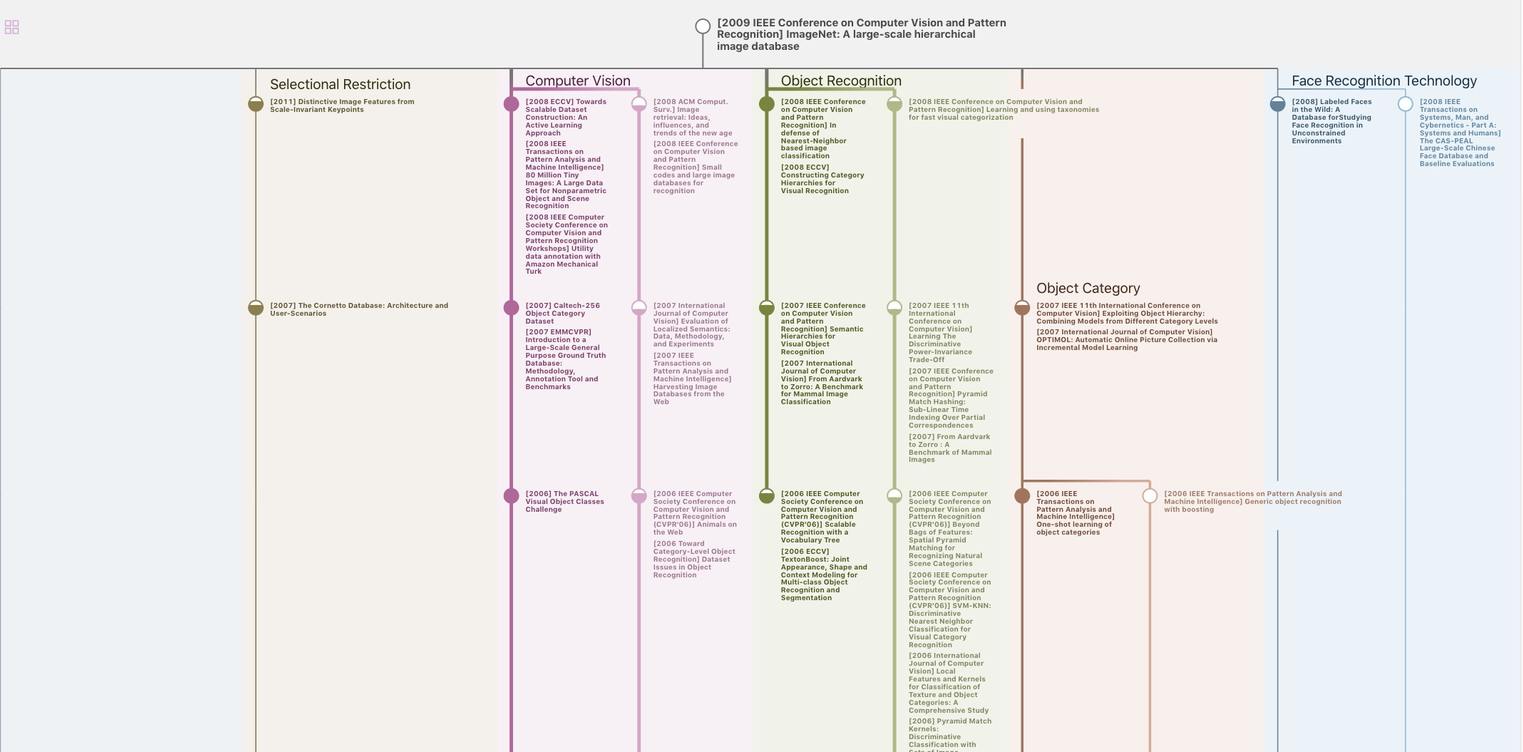
生成溯源树,研究论文发展脉络
Chat Paper
正在生成论文摘要