Development and Validation of a Machine Learning Model to Identify Patients Before Surgery at High Risk for Postoperative Adverse Events
JAMA network open(2023)
摘要
Importance Identifying patients at high risk of adverse outcomes prior to surgery may allow for interventions associated with improved postoperative outcomes; however, few tools exist for automated prediction. Objective To evaluate the accuracy of an automated machine-learning model in the identification of patients at high risk of adverse outcomes from surgery using only data in the electronic health record. Design, Setting, and Participants This prognostic study was conducted among 1477561 patients undergoing surgery at 20 community and tertiary care hospitals in the University of Pittsburgh Medical Center (UPMC) health network. The study included 3 phases: (1) building and validating a model on a retrospective population, (2) testing model accuracy on a retrospective population, and (3) validating the model prospectively in clinical care. A gradient-boosted decision tree machine learning method was used for developing a preoperative surgical risk prediction tool. The Shapley additive explanations method was used for model interpretability and further validation. Accuracy was compared between the UPMC model and National Surgical Quality Improvement Program (NSQIP) surgical risk calculator for predicting mortality. Data were analyzed from September through December 2021. Exposure Undergoing any type of surgical procedure. Main Outcomes and Measures Postoperative mortality and major adverse cardiac and cerebrovascular events (MACCEs) at 30 days were evaluated. Results Among 1477561 patients included in model development (806148 females [54.5%; mean [SD] age, 56.8 [17.9] years), 1016966 patient encounters were used for training and 254242 separate encounters were used for testing the model. After deployment in clinical use, another 206353 patients were prospectively evaluated; an additional 902 patients were selected for comparing the accuracy of the UPMC model and NSQIP tool for predicting mortality. The area under the receiver operating characteristic curve (AUROC) for mortality was 0.972 (95% CI, 0.971-0.973) for the training set and 0.946 (95% CI, 0.943-0.948) for the test set. The AUROC for MACCE and mortality was 0.923 (95% CI, 0.922-0.924) on the training and 0.899 (95% CI, 0.896-0.902) on the test set. In prospective evaluation, the AUROC for mortality was 0.956 (95% CI, 0.953-0.959), sensitivity was 2148 of 2517 patients (85.3%), specificity was 186286 of 203836 patients (91.4%), and negative predictive value was 186286 of 186655 patients (99.8%). The model outperformed the NSQIP tool as measured by AUROC (0.945 [95% CI, 0.914-0.977] vs 0.897 [95% CI, 0.854-0.941], for a difference of 0.048), specificity (0.87 [95% CI, 0.83-0.89] vs 0.68 [95% CI, 0.65-0.69]), and accuracy (0.85 [95% CI, 0.82-0.87] vs 0.69 [95% CI, 0.66, 0.72]). Conclusions and Relevance This study found that an automated machine learning model was accurate in identifying patients undergoing surgery who were at high risk of adverse outcomes using only preoperative variables within the electronic health record, with superior performance compared with the NSQIP calculator. These findings suggest that using this model to identify patients at increased risk of adverse outcomes prior to surgery may allow for individualized perioperative care, which may be associated with improved outcomes.
更多查看译文
关键词
postoperative adverse events,machine learning model,machine learning,adverse events,patients
AI 理解论文
溯源树
样例
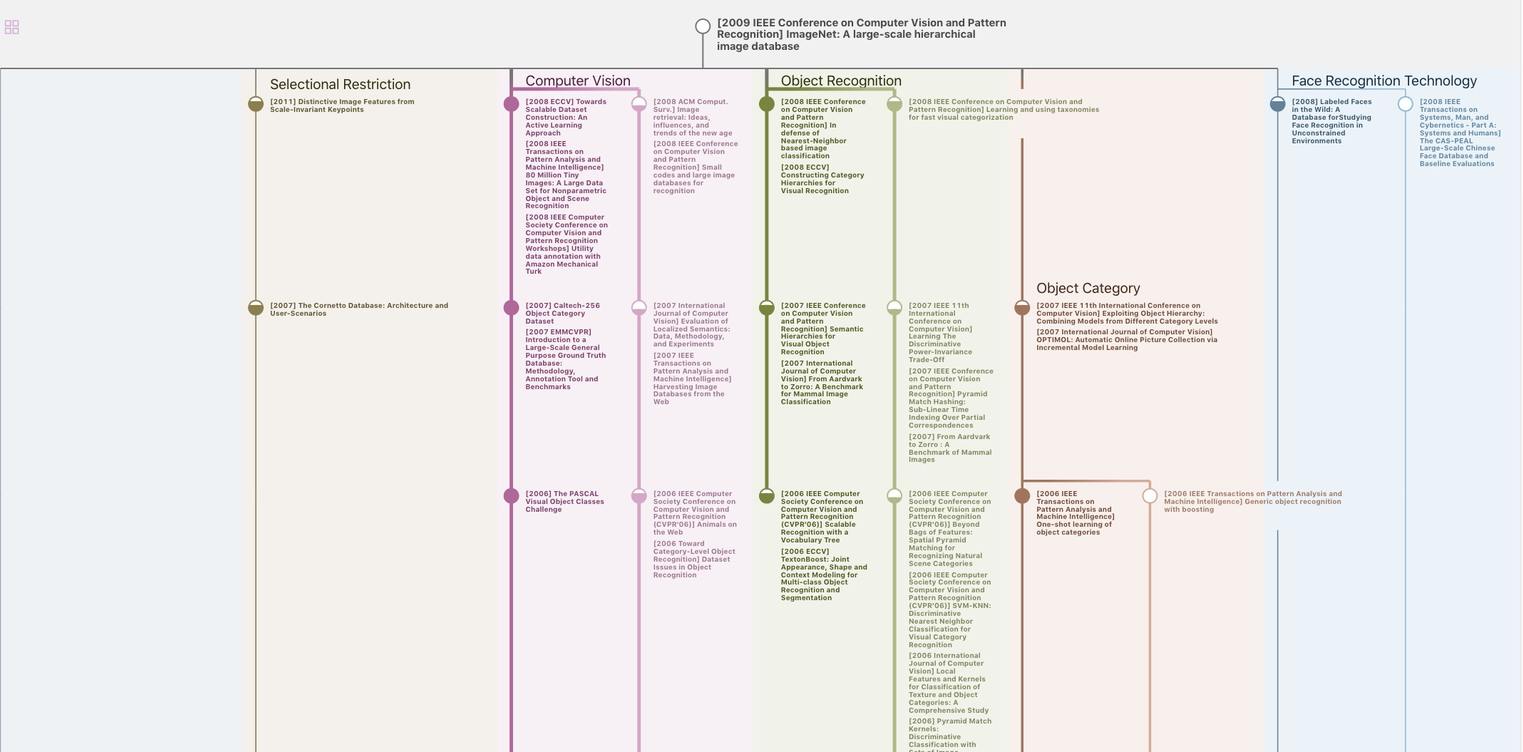
生成溯源树,研究论文发展脉络
Chat Paper
正在生成论文摘要