Deep quantum neural networks on a superconducting processor
Nature Communications(2023)
摘要
Deep learning and quantum computing have achieved dramatic progresses in recent years. The interplay between these two fast-growing fields gives rise to a new research frontier of quantum machine learning. In this work, we report an experimental demonstration of training deep quantum neural networks via the backpropagation algorithm with a six-qubit programmable superconducting processor. We experimentally perform the forward process of the backpropagation algorithm and classically simulate the backward process. In particular, we show that three-layer deep quantum neural networks can be trained efficiently to learn two-qubit quantum channels with a mean fidelity up to 96.0% and the ground state energy of molecular hydrogen with an accuracy up to 93.3% compared to the theoretical value. In addition, six-layer deep quantum neural networks can be trained in a similar fashion to achieve a mean fidelity up to 94.8% for learning single-qubit quantum channels. Our experimental results indicate that the number of coherent qubits required to maintain does not scale with the depth of the deep quantum neural network, thus providing a valuable guide for quantum machine learning applications with both near-term and future quantum devices.
更多查看译文
关键词
deep quantum,neural networks
AI 理解论文
溯源树
样例
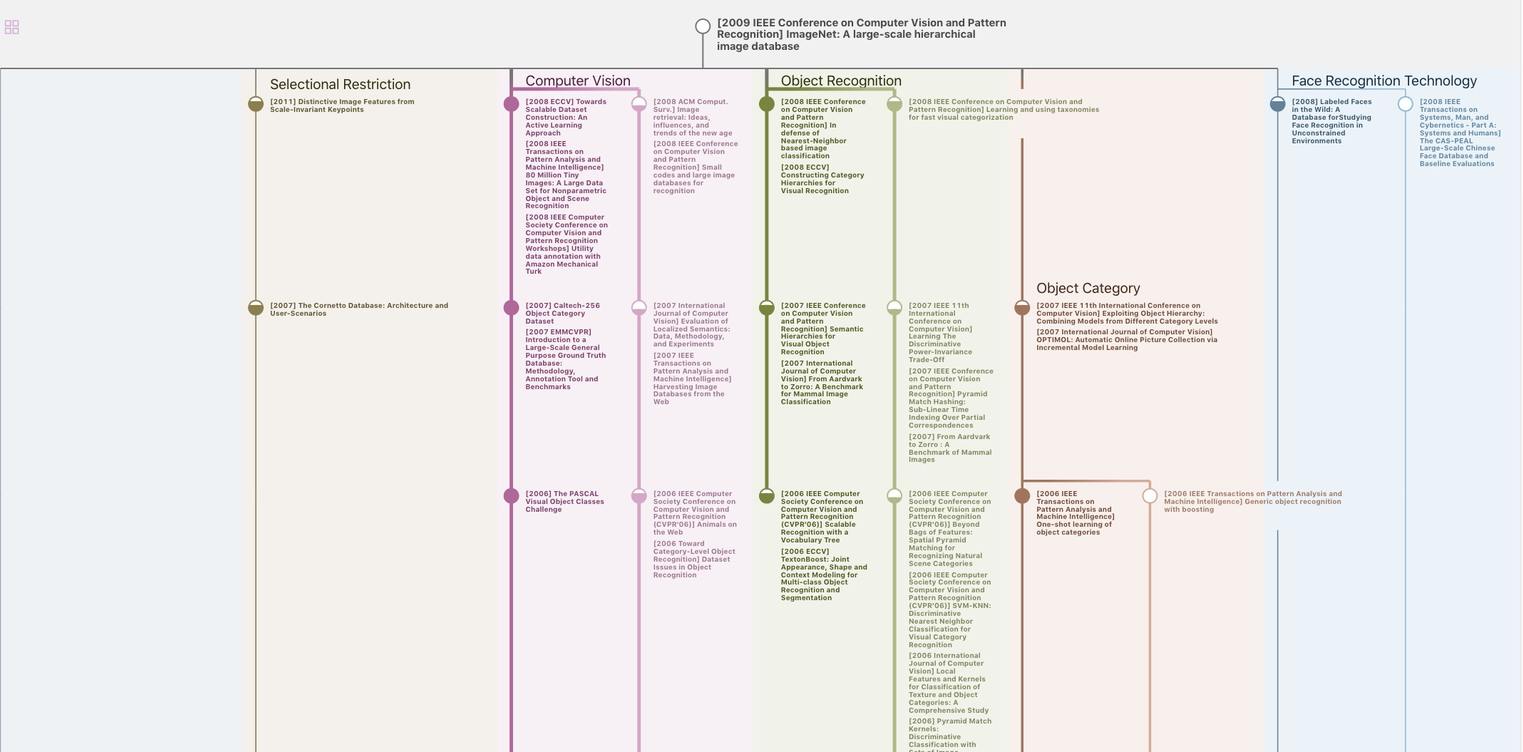
生成溯源树,研究论文发展脉络
Chat Paper
正在生成论文摘要