Wearable movement-tracking data identify Parkinson’s disease years before clinical diagnosis
Nature Medicine(2023)
摘要
Parkinson’s disease is a progressive neurodegenerative movement disorder with a long latent phase and currently no disease-modifying treatments. Reliable predictive biomarkers that could transform efforts to develop neuroprotective treatments remain to be identified. Using UK Biobank, we investigated the predictive value of accelerometry in identifying prodromal Parkinson’s disease in the general population and compared this digital biomarker with models based on genetics, lifestyle, blood biochemistry or prodromal symptoms data. Machine learning models trained using accelerometry data achieved better test performance in distinguishing both clinically diagnosed Parkinson’s disease ( n = 153) (area under precision recall curve (AUPRC) 0.14 ± 0.04) and prodromal Parkinson’s disease ( n = 113) up to 7 years pre-diagnosis (AUPRC 0.07 ± 0.03) from the general population ( n = 33,009) compared with all other modalities tested (genetics: AUPRC = 0.01 ± 0.00, P = 2.2 × 10 −3 ; lifestyle: AUPRC = 0.03 ± 0.04, P = 2.5 × 10 −3 ; blood biochemistry: AUPRC = 0.01 ± 0.00, P = 4.1 × 10 −3 ; prodromal signs: AUPRC = 0.01 ± 0.00, P = 3.6 × 10 −3 ). Accelerometry is a potentially important, low-cost screening tool for determining people at risk of developing Parkinson’s disease and identifying participants for clinical trials of neuroprotective treatments.
更多查看译文
关键词
parkinsons disease,movement-tracking
AI 理解论文
溯源树
样例
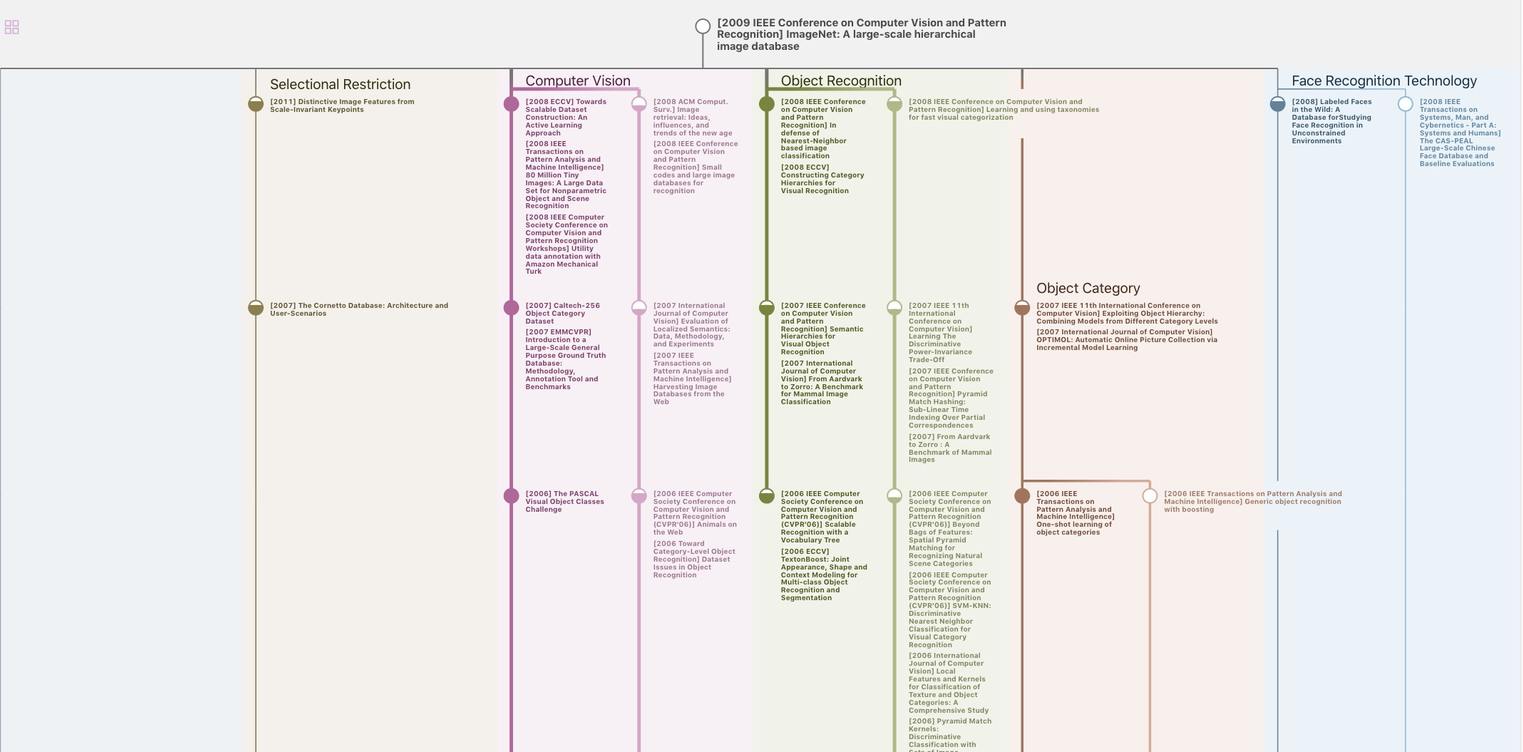
生成溯源树,研究论文发展脉络
Chat Paper
正在生成论文摘要