Iterative Approach of Experiment-Machine Learning for Efficient Optimization of Environmental Catalysts: An Example of NO x Selective Reduction Catalysts
Environmental science & technology(2023)
摘要
Minimal research works on the application of artificialintelligence technology in environmental catalyst development. Thisstudy reports an iteration approach of a data-driven model with labexperiments to develop a novel catalyst of atmospheric pollutantsrapidly. An iterative approachbetween machine learning (ML) and laboratoryexperiments was developed to accelerate the design and synthesis ofenvironmental catalysts (ECs) using selective catalytic reduction(SCR) of nitrogen oxides (NO x ) as an example.The main steps in the approach include training a ML model using therelevant data collected from the literature, screening candidate catalystsfrom the trained model, experimentally synthesizing and characterizingthe candidates, updating the ML model by incorporating the new experimentalresults, and screening promising catalysts again with the updatedmodel. This process is iterated with a goal to obtain an optimizedcatalyst. Using the iterative approach in this study, a novel SCRNO x catalyst with low cost, high activity,and a wide range of application temperatures was found and successfullysynthesized after four iterations. The approach is general enoughthat it can be readily extended for screening and optimizing the designof other environmental catalysts and has strong implications for thediscovery of other environmental materials.
更多查看译文
关键词
environmental catalysts,machine learning,iteration between machine learning and experiments,nitrogenoxides,selective catalytic reduction
AI 理解论文
溯源树
样例
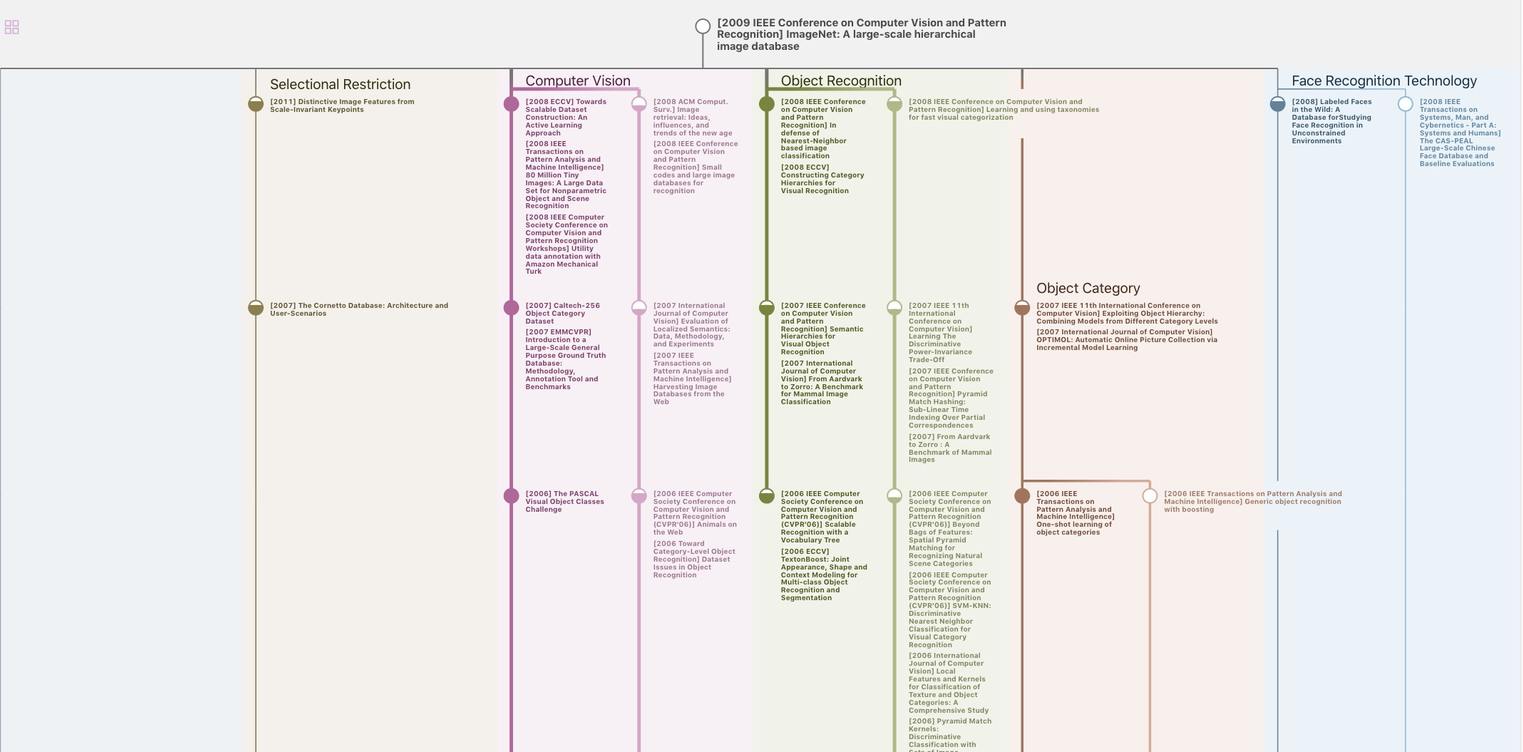
生成溯源树,研究论文发展脉络
Chat Paper
正在生成论文摘要