Applying an interpretable machine learning framework to study mobility inequity in the recovery phase of COVID-19 pandemic
Travel behaviour & society(2023)
摘要
The COVID-19 pandemic is a public health crisis that also fuels the pervasive social inequity in the United States. Existing studies have extensively analyzed the inequity issues on mobility across different demographic groups during the lockdown phase. However, it is unclear whether the mobility inequity is perennial and will continue into the mobility recovery phase. This study utilizes ride-hailing data from Jan 1st, 2019, to Mar 31st, 2022, in Chicago to analyze the impact of various factors, such as demographic, land use, and transit connectivity, on mobility inequity in the different recovery phases. Instead of commonly used statistical methods, this study leverages advanced time-series clustering and an interpretable machine learning algorithm. The result demonstrates that inequity still exists in the mobility recovery phase of the COVID-19 pandemic, and the degree of mobility inequity in different recovery phases is varied. Furthermore, mobility inequity is more likely to exist in the census tract with more families without children, lower health insurance coverage, inflexible workstyle, more African Americans, higher poverty rate, fewer commercial land use, and higher Gini index. This study aims to further the understanding of the social inequity issue during the mobility recovery phase of the COVID-19 pandemic and help governments propose proper policies to tackle the unequal impact of the pandemic.
更多查看译文
关键词
COVID-19 pandemic,Mobility inequity,Time-series clustering,Interpretable machine learning model
AI 理解论文
溯源树
样例
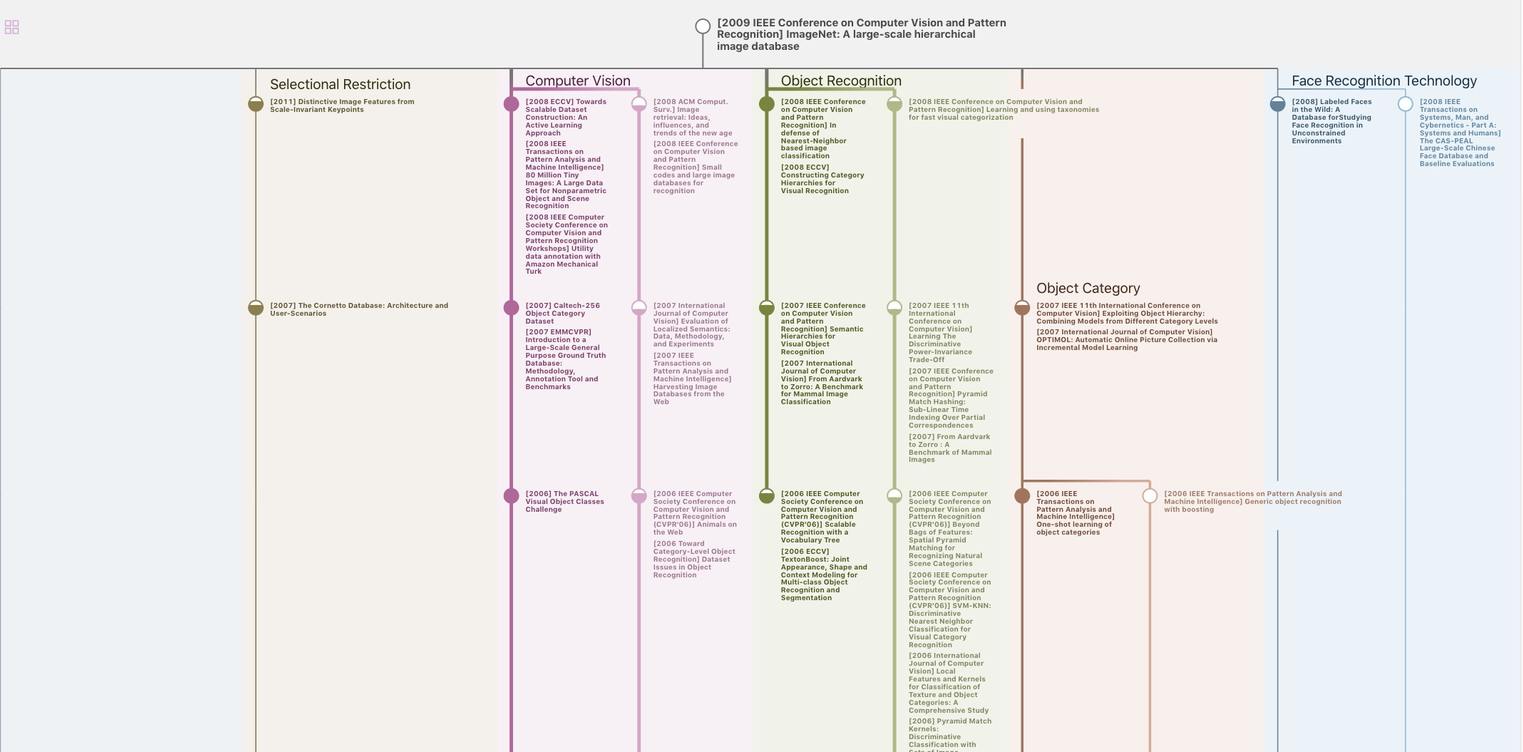
生成溯源树,研究论文发展脉络
Chat Paper
正在生成论文摘要