Recurrent and Convolution Neural Networks for Sequential Multispectral Optoacoustic Tomography (MSOT) Imaging.
Journal of biophotonics(2023)
摘要
Multispectral optoacoustic tomography (MSOT) is a beneficial technique for diagnosing and analyzing biological samples since it provides meticulous details in anatomy and physiology. However, acquiring high through-plane resolution volumetric MSOT is time-consuming. Here, we propose deep learning based on hybrid recurrent and convolution neural networks to generate sequential cross-sectional images for a MSOT system. This system provides three modalities (MSOT, ultrasound, and optoacoustic imaging of a specific exogenous contrast agent) in a single scan. This study used ICG-conjugated nanoworms particles (NWs-ICG) as the contrast agent. Instead of acquiring seven images with a step size of 0.1 mm, we can receive two images with a step size of 0.6 mm as input images for the proposed deep learning model. The deep learning model can generate other five images with the step size of 0.1 mm between these two input images meaning we can reduce acquisition time by approximately 71%. This article is protected by copyright. All rights reserved.
更多查看译文
关键词
convolutional neural networks, multispectral optoacoustic tomography, recurrent neural networks, volumetric imaging
AI 理解论文
溯源树
样例
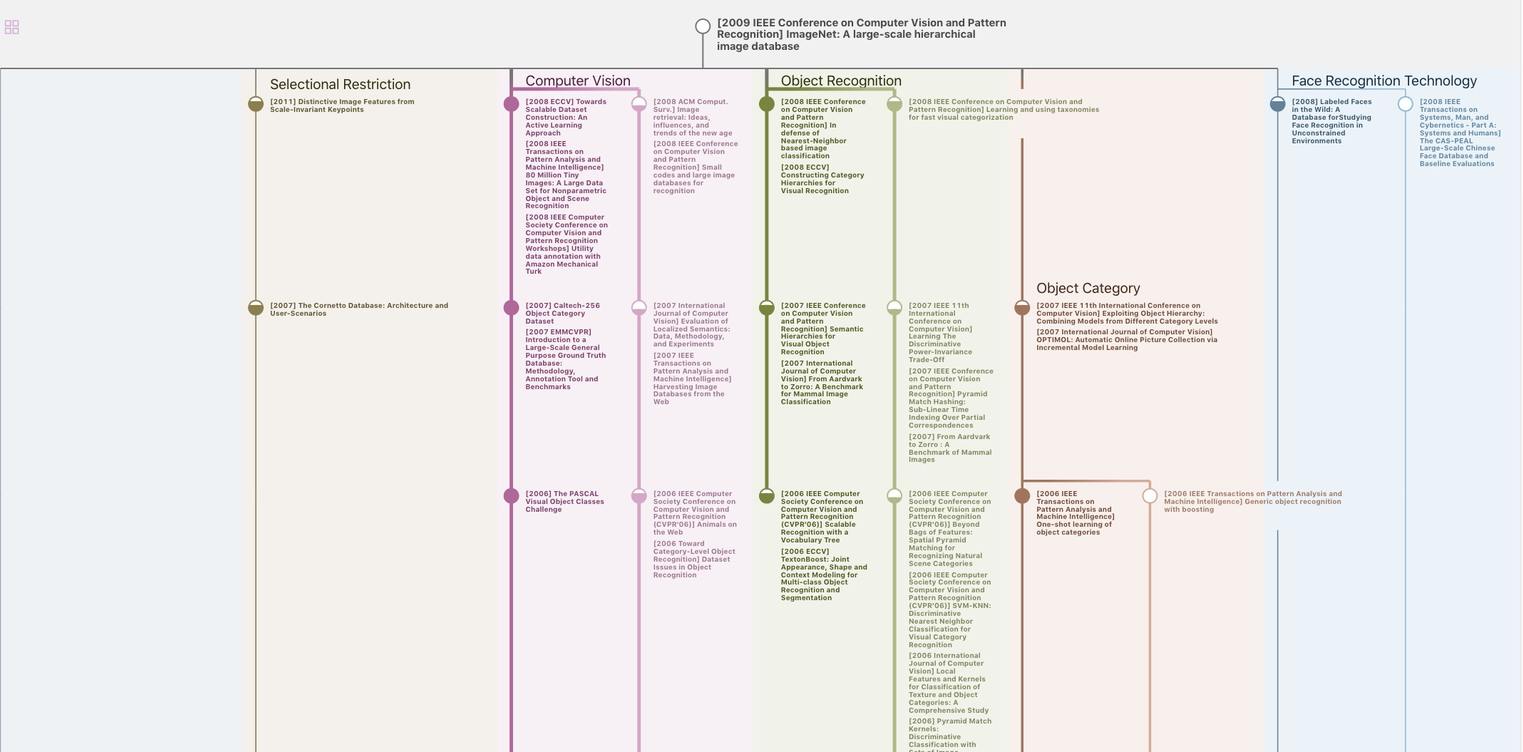
生成溯源树,研究论文发展脉络
Chat Paper
正在生成论文摘要