Can 1.25 mm thin-section images generated with Deep Learning Image Reconstruction technique replace standard-of-care 5 mm images in abdominal CT?
Abdominal radiology (New York)(2023)
摘要
Background CT image reconstruction has evolved from filtered back projection to hybrid- and model-based iterative reconstruction. Deep learning-based image reconstruction is a relatively new technique that uses deep convolutional neural networks to improve image quality. Objective To evaluate and compare 1.25 mm thin-section abdominal CT images reconstructed with deep learning image reconstruction (DLIR) with 5 mm thick images reconstructed with adaptive statistical iterative reconstruction (ASIR-V). Methods This retrospective study included 52 patients (31 F; 56.9±16.9 years) who underwent abdominal CT scans between August-October 2019. Image reconstruction was performed to generate 5 mm images at 40% ASIR-V and 1.25 mm DLIR images at three strengths (low [DLIR-L], medium [DLIR-M], and high [DLIR-H]). Qualitative assessment was performed to determine image noise, contrast, visibility of small structures, sharpness, and artifact based on a 5-point-scale. Image preference determination was based on a 3-point-scale. Quantitative assessment included measurement of attenuation, image noise, and contrast-to-noise ratios (CNR). Results Thin-section images reconstructed with DLIR-M and DLIR-H yielded better image quality scores than 5 mm ASIR-V reconstructed images. Mean qualitative scores of DLIR-H for noise (1.77 ± 0.71), contrast (1.6 ± 0.68), small structure visibility (1.42 ± 0.66), sharpness (1.34 ± 0.55), and image preference (1.11 ± 0.34) were the best (p<0.05). DLIR-M yielded intermediate scores. All DLIR reconstructions showed superior ratings for artifacts compared to ASIR-V (p<0.05), whereas each DLIR group performed comparably (p>0.05, 0.405-0.763). In the quantitative assessment, there were no significant differences in attenuation values between all reconstructions (p>0.05). However, DLIR-H demonstrated the lowest noise (9.17 ± 3.11) and the highest CNR (CNR liver = 26.88 ± 6.54 and CNR portal vein = 7.92 ± 3.85) (all p<0.001). Conclusion DLIR allows generation of thin-section (1.25 mm) abdominal CT images, which provide improved image quality with higher inter-reader agreement compared to 5 mm thick images reconstructed with ASIR-V. Clinical Impact Improved image quality of thin-section CT images reconstructed with DLIR has several benefits in clinical practice, such as improved diagnostic performance without radiation dose penalties. Graphical Abstract
更多查看译文
关键词
Computed tomography,Deep learning image reconstruction,ASIR,Abdomen
AI 理解论文
溯源树
样例
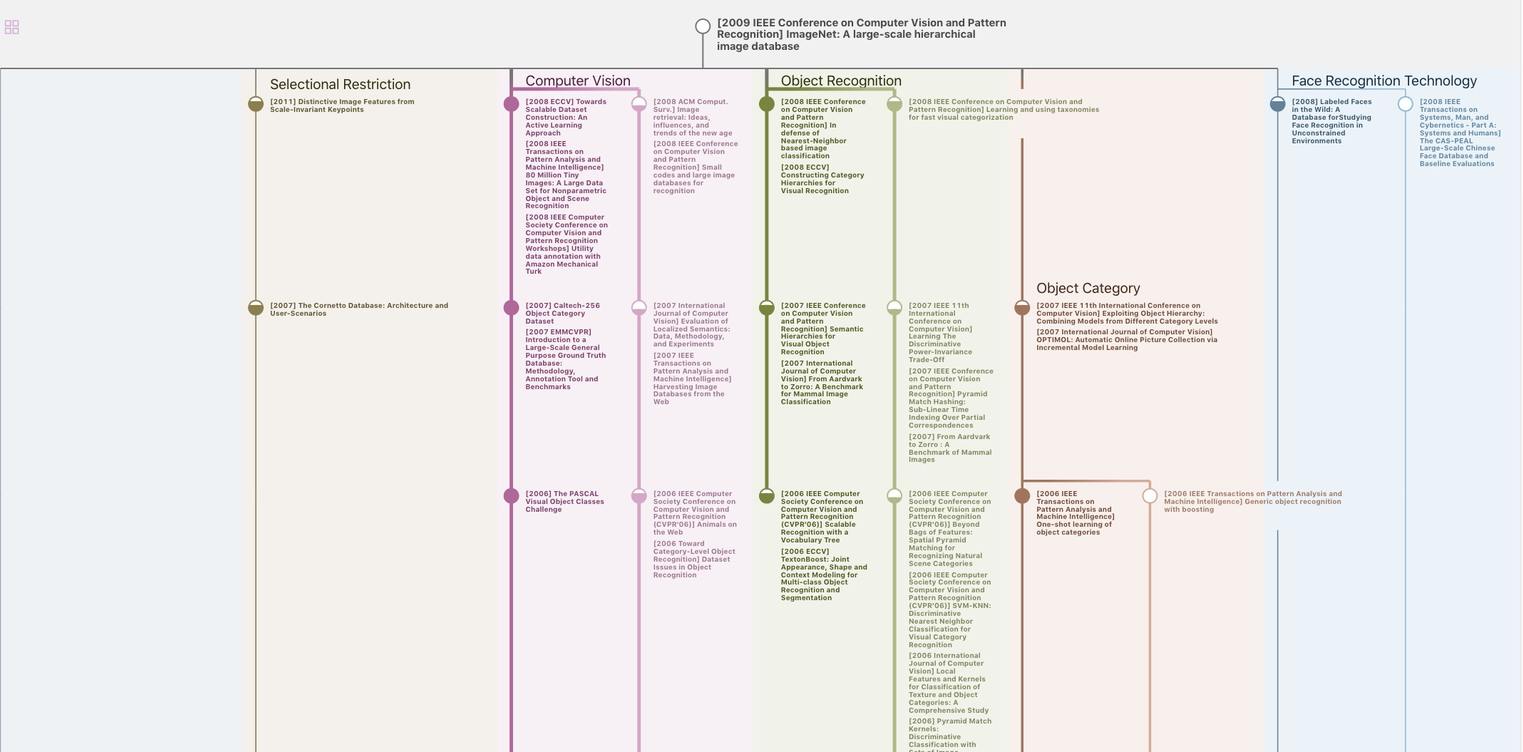
生成溯源树,研究论文发展脉络
Chat Paper
正在生成论文摘要