Deep learning approach for differentiating indeterminate adrenal masses using CT imaging
Abdominal radiology (New York)(2023)
摘要
Purpose Distinguishing stage 1–2 adrenocortical carcinoma (ACC) and large, lipid poor adrenal adenoma (LPAA) via imaging is challenging due to overlapping imaging characteristics. This study investigated the ability of deep learning to distinguish ACC and LPAA on single time-point CT images. Methods Retrospective cohort study from 1994 to 2022. Imaging studies of patients with adrenal masses who had available adequate CT studies and histology as the reference standard by method of adrenal biopsy and/or adrenalectomy were included as well as four patients with LPAA determined by stability or regression on follow-up imaging. Forty-eight (48) subjects with pathology-proven, stage 1–2 ACC and 43 subjects with adrenal adenoma >3 cm in size demonstrating a mean non-contrast CT attenuation > 20 Hounsfield Units centrally were included. We used annotated single time-point contrast-enhanced CT images of these adrenal masses as input to a 3D Densenet121 model for classifying as ACC or LPAA with five-fold cross-validation. For each fold, two checkpoints were reported, highest accuracy with highest sensitivity (accuracy focused) and highest sensitivity with the highest accuracy (sensitivity focused). Results We trained a deep learning model (3D Densenet121) to predict ACC versus LPAA. The sensitivity-focused model achieved mean accuracy: 87.2% and mean sensitivity: 100%. The accuracy-focused model achieved mean accuracy: 91% and mean sensitivity: 96%. Conclusion Deep learning demonstrates promising results distinguishing between ACC and large LPAA using single time-point CT images. Before being widely adopted in clinical practice, multicentric and external validation are needed.
更多查看译文
关键词
Adrenocortical carcinoma,Lipid poor adrenal adenoma,Computed tomography,Deep learning
AI 理解论文
溯源树
样例
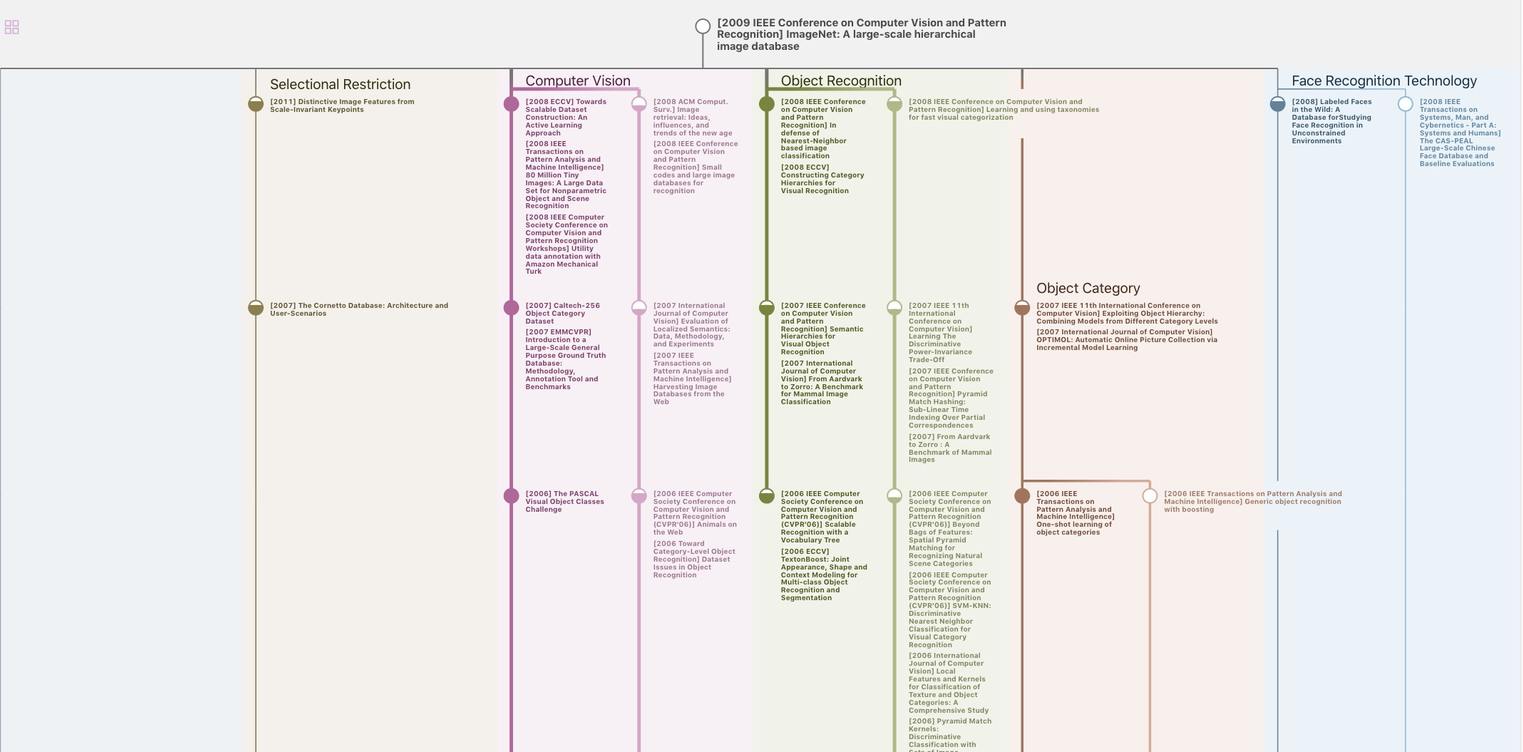
生成溯源树,研究论文发展脉络
Chat Paper
正在生成论文摘要