Network communications flexibly predict visual contents that enhance representations for faster visual categorization.
The Journal of neuroscience : the official journal of the Society for Neuroscience(2023)
Abstract
Models of visual cognition generally assume that brain networks predict the contents of a stimulus to facilitate its subsequent categorization. However, understanding prediction and categorization at a network level has remained challenging, partly because we need to reverse engineer their information processing mechanisms from the dynamic neural signals. Here, we used connectivity measures that can isolate the communications of a specific content to reconstruct these network mechanisms in each individual participant (N=11, both sexes). Each was cued to the spatial location (left vs. right) and contents (Low vs. High Spatial Frequency, LSF vs. HSF) of a predicted Gabor stimulus that they then categorized. Using each participant's concurrently measured MEG, we reconstructed networks that predict and categorize LSF vs. HSF contents for behavior. We found that predicted contents flexibly propagate top-down from temporal to lateralized occipital cortex, depending on task demands, under supervisory control of prefrontal cortex. When they reach lateralized occipital cortex, predictions enhance the bottom-up LSF vs. HSF representations of the stimulus, all the way from occipital-ventral-parietal to pre-motor cortex, in turn producing faster categorization behavior. Importantly, content communications are subsets (i.e. 55-75%) of the signal-to-signal communications typically measured between brain regions. Hence, our study isolates functional networks that process the information of cognitive functions.An enduring cognitive hypothesis states that our perception is partly influenced by the bottom-up sensory input, but also by top-down expectations. However, cognitive explanations of the dynamic brain networks mechanisms that flexibly predict and categorize the visual input according to task-demands remain elusive. We addressed them in a predictive experimental design, by isolating the network communications of cognitive contents from all other communications. Our methods revealed a Prediction Network that flexibly communicates contents from temporal to lateralized occipital cortex, with explicit frontal control, and an occipital-ventral-parietal-frontal Categorization Network that represents more sharply the predicted contents from the shown stimulus, leading to faster behavior. Our framework and results therefore shed a new light of cognitive information processing on dynamic brain activity.
MoreTranslated text
Key words
enhance representations,network
AI Read Science
Must-Reading Tree
Example
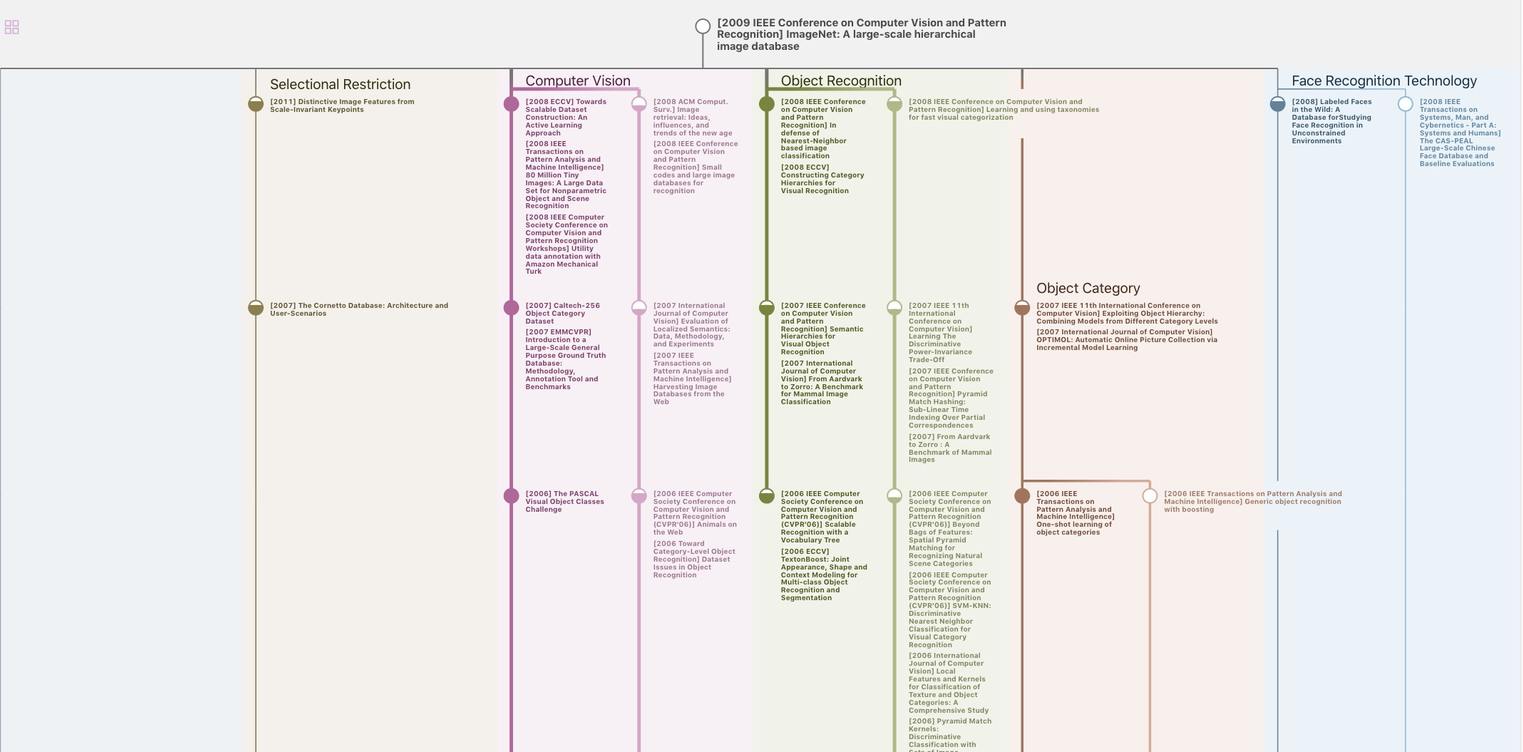
Generate MRT to find the research sequence of this paper
Chat Paper
Summary is being generated by the instructions you defined