Editorial for "A Lightweight Convolutional Neural Network Based on Dynamic Level-Set Loss Function for Spine MR Image Segmentation".
Journal of magnetic resonance imaging JMRI(2023)
摘要
Journal of Magnetic Resonance ImagingEarly View Editorial Editorial for "A Lightweight Convolutional Neural Network Based on Dynamic Level-Set Loss Function for Spine MR Image Segmentation" Hayaru Shouno PhD, Corresponding Author Hayaru Shouno PhD [email protected] orcid.org/0000-0002-2412-0184 Graduate School of Informatics and Engineering, The University of Electro-Communications, Chofu, Tokyo, Japan[email protected]Search for more papers by this authorTomohisa Okada MD, PhD, Tomohisa Okada MD, PhD orcid.org/0000-0003-2312-5677 Human Brain Research Center, Graduate School of Medicine, Kyoto University, Kyoto, JapanSearch for more papers by this author Hayaru Shouno PhD, Corresponding Author Hayaru Shouno PhD [email protected] orcid.org/0000-0002-2412-0184 Graduate School of Informatics and Engineering, The University of Electro-Communications, Chofu, Tokyo, Japan[email protected]Search for more papers by this authorTomohisa Okada MD, PhD, Tomohisa Okada MD, PhD orcid.org/0000-0003-2312-5677 Human Brain Research Center, Graduate School of Medicine, Kyoto University, Kyoto, JapanSearch for more papers by this author First published: 27 June 2023 https://doi.org/10.1002/jmri.28878 Evidence Level: 5 Technical Efficacy: Stage 1 Read the full textAboutPDF ToolsRequest permissionExport citationAdd to favoritesTrack citation ShareShare Give accessShare full text accessShare full-text accessPlease review our Terms and Conditions of Use and check box below to share full-text version of article.I have read and accept the Wiley Online Library Terms and Conditions of UseShareable LinkUse the link below to share a full-text version of this article with your friends and colleagues. Learn more.Copy URL Share a linkShare onEmailFacebookTwitterLinkedInRedditWechat No abstract is available for this article. References 1He S, Li Q, Li X, Zhang M. Dynamic level-set loss function based lightweight convolutional neural network for spine MR image segmentation. J Magn Reson Imaging. https://doi.org/10.1002/jmri.28877. 2Zheng G, Chu C, Belavý DL, et al. Evaluation and comparison of 3D intervertebral disc localization and segmentation methods for 3D T2 MR data: A grand challenge. Med Image Anal 2017; 35: 327-344. 3He S, Li Q, Li X, Zhang M. LSW-Net: A lightweight convolutional neural network based on dynamic level-set loss function for spine MR image segmentation. J Magn Reson Imaging 2023; Epub ahead of print. 4He S, Li Q, Li X, Zhang M. An optimized segmentation convolutional neural network with dynamic energy loss function for 3D reconstruction of lumbar spine MR images. Comput Biol Med 2023; 160:106839. 5Uteri T, Rankovi V, Milovanovi V, Kovaevi V, Rasuli L, Filipovi N. A deep learning model for automatic detection and classification of disc herniation in magnetic resonance images. IEEE J Biomed Health 2022; 26: 6036-6046. 6Sollmann N, Löffler MT, Kronthaler S, et al. MRI-based quantitative osteoporosis imaging at the spine and femur. J Magn Reson Imaging 2021; 54: 12-35. 7Yang B, Wendland MF, O'Connell GD. Direct quantification of intervertebral disc water content using MRI. J Magn Reson Imaging 2020; 52: 1152-1162. 8Wang M, Tsang A, Tam V, Chan D, Cao P, Wu EX. Multiparametric MR investigation of proteoglycan diffusivity, T2 relaxation, and concentration in an ex vivo model of intervertebral disc degeneration. J Magn Reson Imaging 2020; 51: 1390-1400. 9Menon RG, Zibetti MVW, Pendola M, Regatte RR. Measurement of three-dimensional internal dynamic strains in the intervertebral disc of the lumbar spine with mechanical loading and golden-angle radial sparse parallel-magnetic resonance imaging. J Magn Reson Imaging 2021; 54: 486-496. Early ViewOnline Version of Record before inclusion in an issue ReferencesRelatedInformation
更多查看译文
AI 理解论文
溯源树
样例
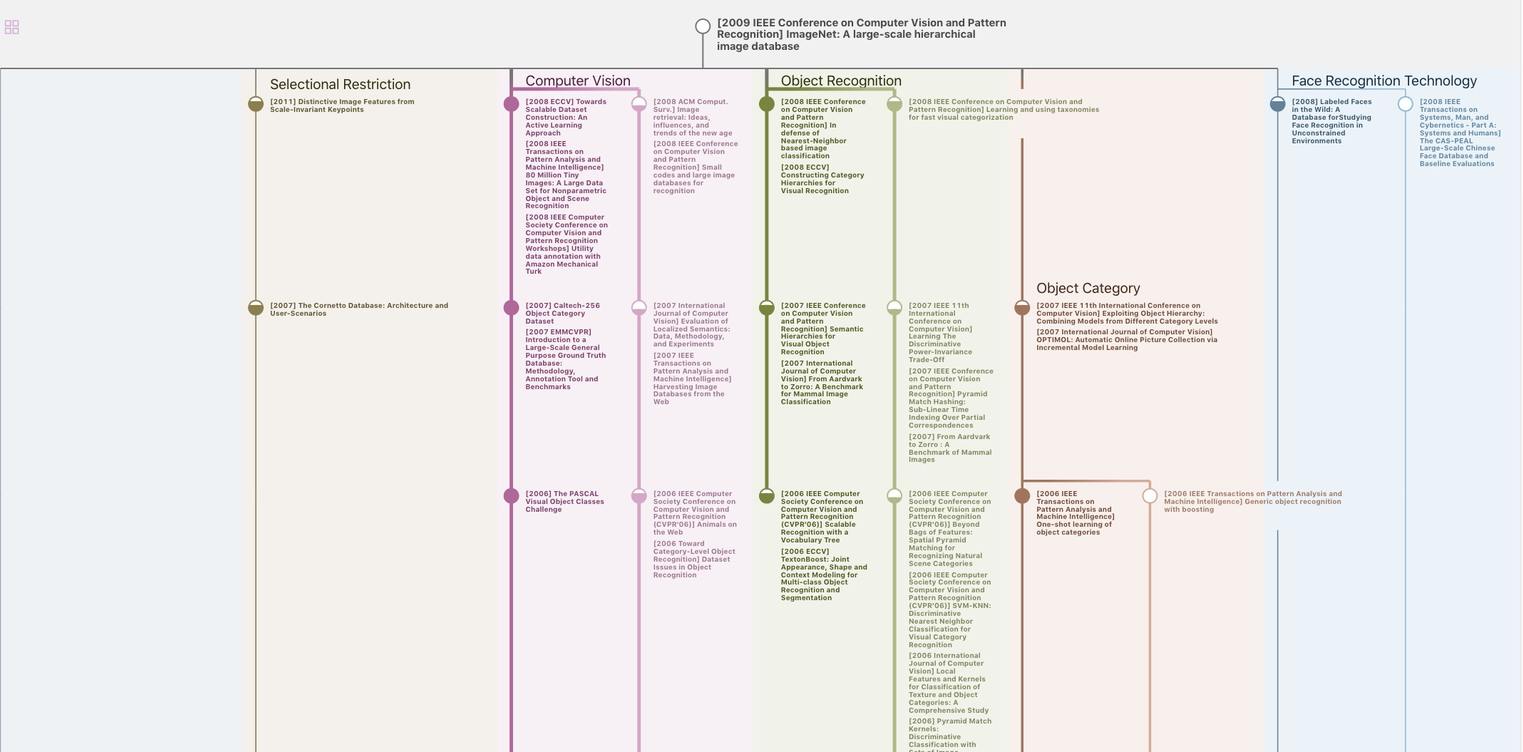
生成溯源树,研究论文发展脉络
Chat Paper
正在生成论文摘要