Machine learning for malignant versus benign focal liver lesions on US and CEUS: a meta-analysis
Abdominal radiology (New York)(2023)
摘要
Objectives To perform a meta-analysis of the diagnostic performance of learning (ML) algorithms (conventional and deep learning algorithms) for the classification of malignant versus benign focal liver lesions (FLLs) on US and CEUS. Methods Available databases were searched for relevant published studies through September 2022. Studies met eligibility criteria if they evaluate the diagnostic performance of ML for the classification of malignant and benign focal liver lesions on US and CEUS. The pooled per-lesion sensitivities and specificities for each modality with 95% confidence intervals were calculated. Results A total of 8 studies on US, 11 on CEUS, and 1 study evaluating both methods met the inclusion criteria with a total of 34,245 FLLs evaluated. The pooled sensitivity and specificity of ML for the malignancy classification of FLLs were 81.7% (95% CI, 77.2–85.4%) and 84.8% (95% CI, 76.0–90.8%) for US, compared to 87.1% (95% CI, 81.8–91.0%) and 87.0% (95% CI, 83.1–90.1%) for CEUS. In the subgroup analysis of studies that evaluated deep learning algorithms, the sensitivity and specificity of CEUS ( n = 4) increased to 92.4% (95% CI, 88.5–95.0%) and 88.2% (95% CI, 81.1–92.9%). Conclusions The diagnostic performance of ML algorithms for the malignant classification of FLLs was high for both US and CEUS with overall similar sensitivity and specificity. The similar performance of US may be related to the higher prevalence of DL models in that group. Graphical abstract
更多查看译文
关键词
US,CEUS,Machine learning,Artificial intelligence,Focal liver lesions
AI 理解论文
溯源树
样例
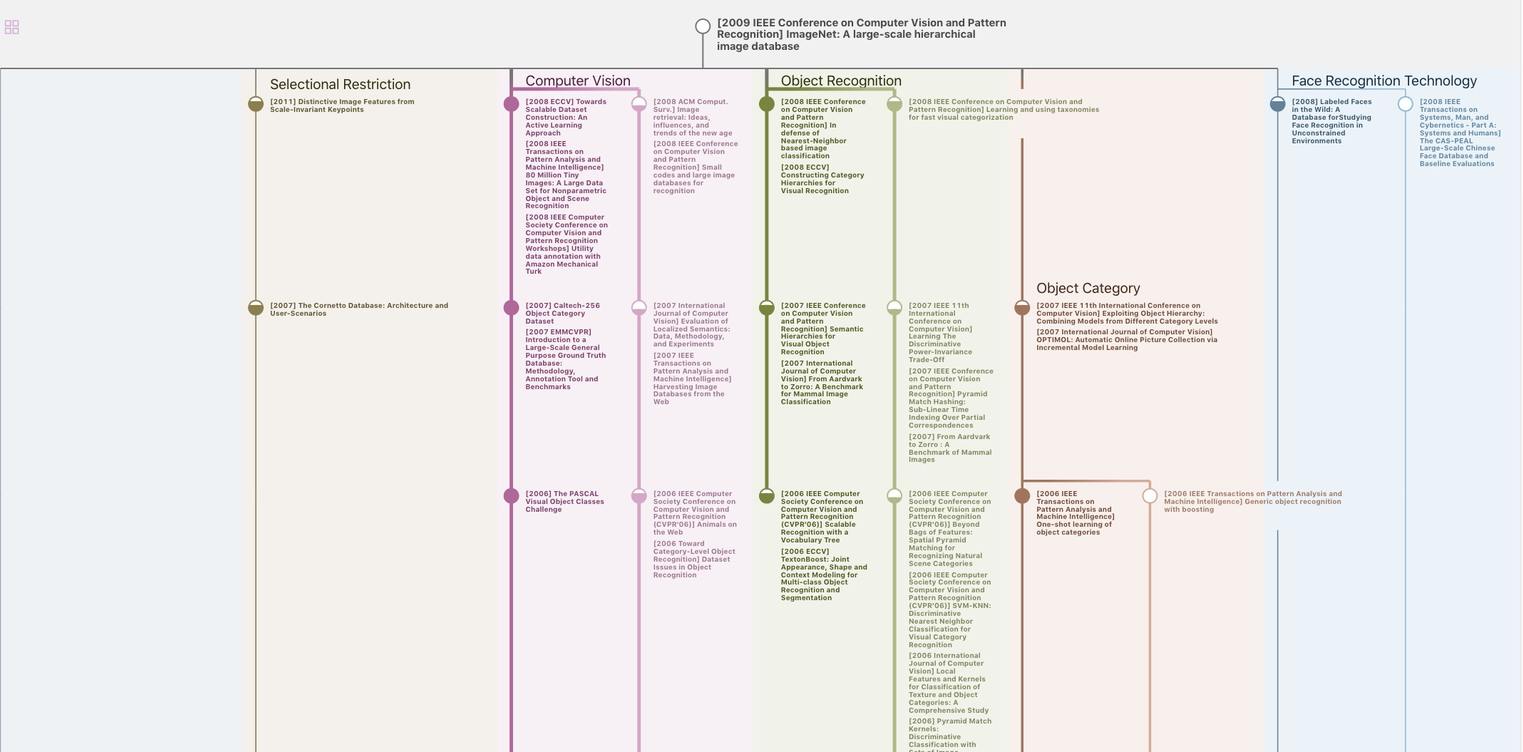
生成溯源树,研究论文发展脉络
Chat Paper
正在生成论文摘要