A patient-specific deep learning framework for 3D motion estimation and volumetric imaging during lung cancer radiotherapy.
Physics in medicine and biology(2023)
摘要
Respiration introduces a constant source of irregular motion that poses a significant challenge for the precise irradiation of thoracic and abdominal cancers. Current real-time motion management strategies require dedicated systems that are not available in most radiotherapy centers. In this paper we introduce Voxelmap, a patient-specific deep learning framework that achieves 3D motion estimation and volumetric imaging using the data and resources available in standard clinical settings. Here we provide proof-of-principle for this framework using imaging data from two lung cancer patients. Using 2D images as input and 3D-3D Elastix registrations as ground-truth, Voxelmap was able to continuously predict 3D tumor motion with mean errors of 0.1 ± 0.5, -0.6 ± 0.8, and 0.0 ± 0.2 mm along the left-right, superior-inferior, and anterior-posterior axes respectively. Voxelmap also predicted 3D thoracoabdominal motion with mean errors of -0.1 ± 0.3, -0.1 ± 0.6, and -0.2 ± 0.2 mm respectively. Moreover, volumetric imaging was achieved with mean average error 0.0003, root-mean-squared error 0.0007, structural similarity 1.0 and peak-signal-to-noise ratio 65.8. The results of this study demonstrate the possibility of achieving 3D motion estimation and volumetric imaging during lung cancer radiotherapy.
更多查看译文
关键词
lung cancer radiotherapy,volumetric imaging,deep learning,3d motion estimation,patient-specific
AI 理解论文
溯源树
样例
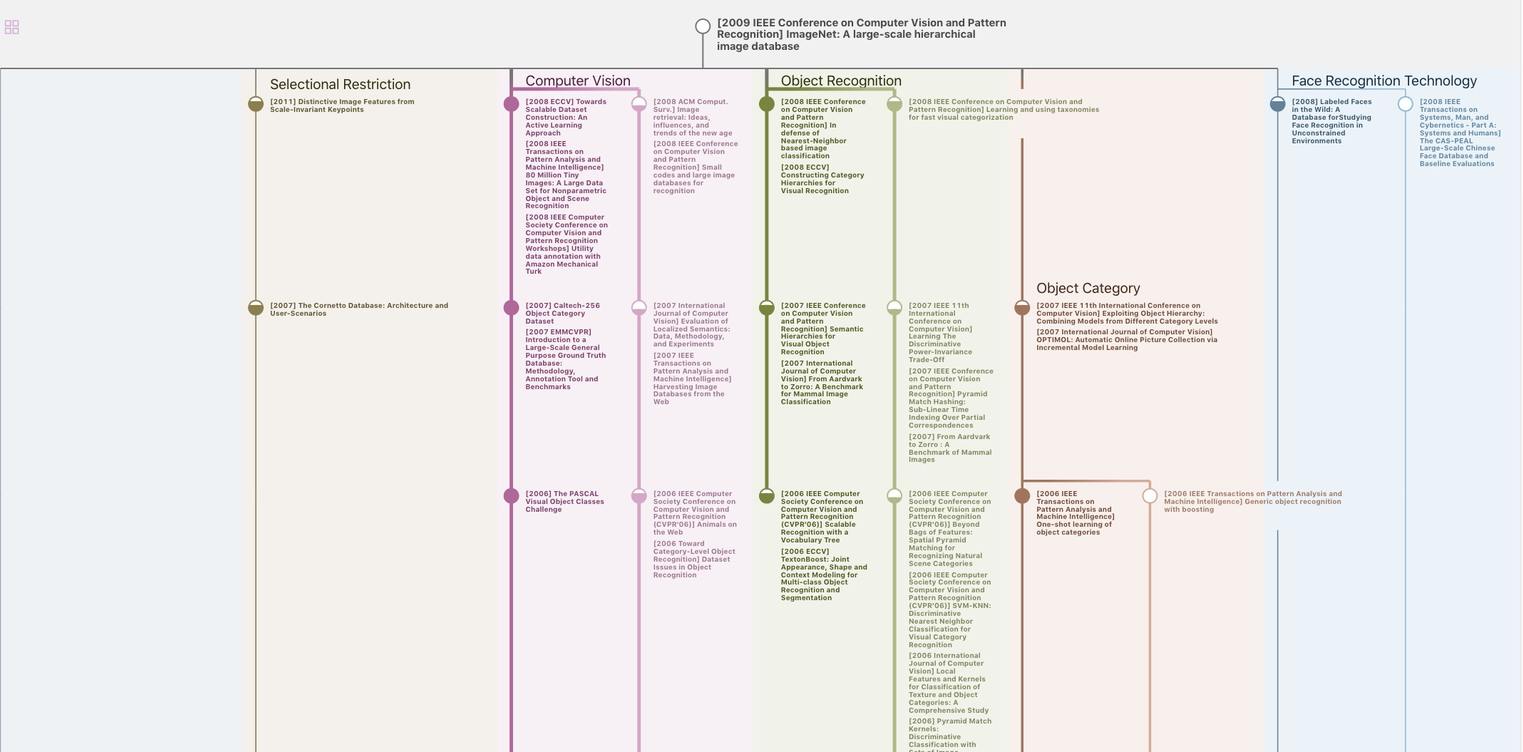
生成溯源树,研究论文发展脉络
Chat Paper
正在生成论文摘要