Assessment of encoder-decoder-based segmentation models for thyroid ultrasound images
Medical & biological engineering & computing(2023)
摘要
Encoder-decoder-based semantic segmentation models classify image pixels into the corresponding class, such as the ROI (region of interest) or background. In the present study, simple / dilated convolution / series / directed acyclic graph (DAG)-based encoder-decoder semantic segmentation models have been implemented, i.e., SegNet (VGG16), SegNet (VGG19), U-Net, mobileNetv2, ResNet18, ResNet50, Xception and Inception networks for the segment TTUS(Thyroid Tumor Ultrasound) images. Transfer learning has been used to train these segmentation networks using original and despeckled TTUS images. The performance of the networks has been calculated using mIoU and mDC metrics. Based on the exhaustive experiments, it has been observed that ResNet50-based segmentation model obtained the best results objectively with values 0.87 for mIoU, 0.94 for mDC, and also according to radiologist opinion on shape, margin, and echogenicity characteristics of segmented lesions. It is noted that the segmentation model, namely ResNet50, provides better segmentation based on objective and subjective assessment. It may be used in the healthcare system to identify thyroid nodules accurately in real time. Graphical Abstract
更多查看译文
关键词
thyroid ultrasound images,segmentation models,encoder-decoder-based
AI 理解论文
溯源树
样例
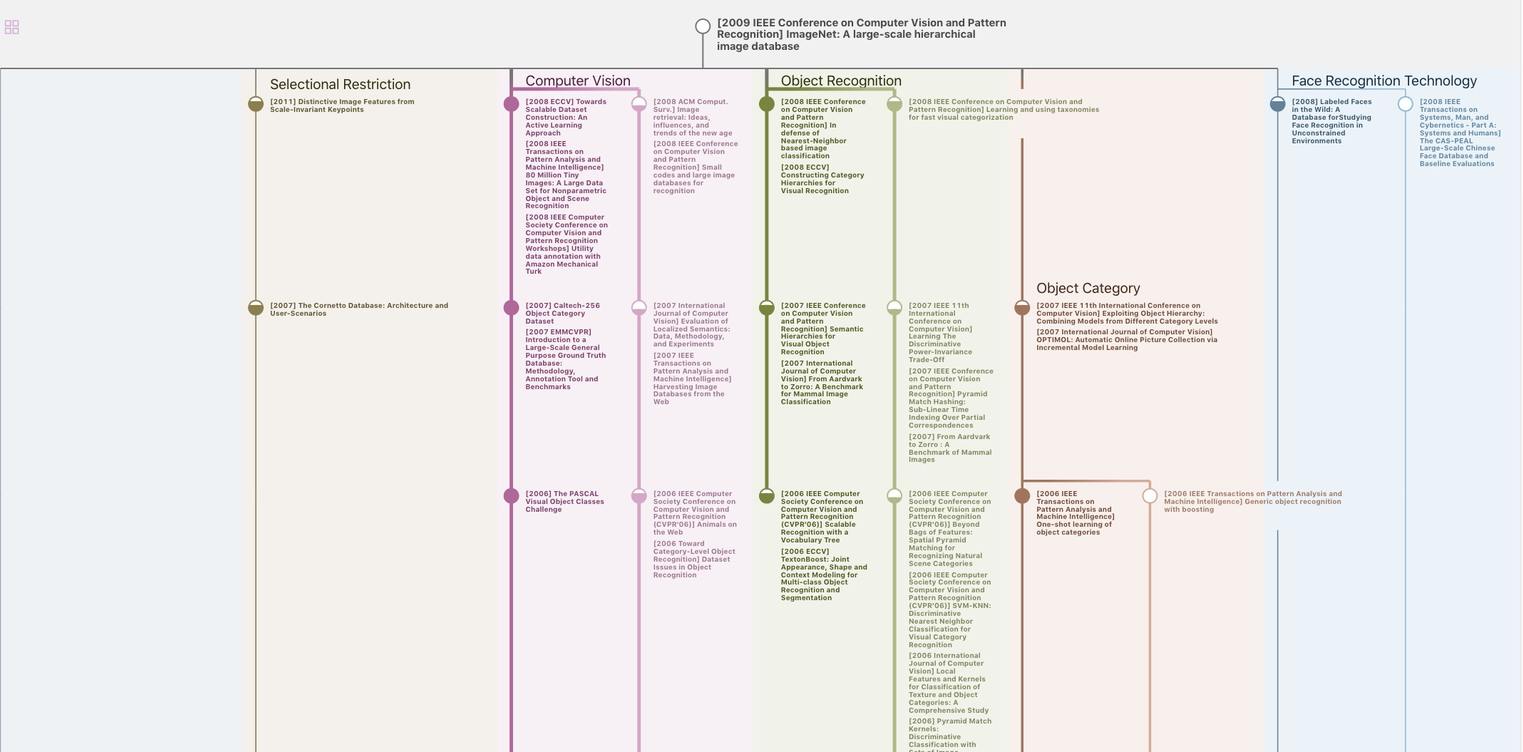
生成溯源树,研究论文发展脉络
Chat Paper
正在生成论文摘要