Towards Interpretable Multimodal Predictive Models for Early Mortality Prediction of Hemorrhagic Stroke Patients.
AMIA Joint Summits on Translational Science proceedings. AMIA Joint Summits on Translational Science(2023)
摘要
The increasing death rate over the past eight years due to stroke has prompted clinicians to look for data-driven decision aids. Recently, deep-learning-based prediction models trained with fine-grained electronic health record (EHR) data have shown superior promise for health outcome prediction. However, the use of EHR-based deep learning models for hemorrhagic stroke outcome prediction has not been extensively explored. This paper proposes an ensemble deep learning framework to predict early mortality among ICU patients with hemorrhagic stroke. The proposed ensemble model achieved an accuracy of 83%, which was higher than the fusion model and other baseline models (logistic regression, decision tree, random forest, and XGBoost). Moreover, we used SHAP values for interpretation of the ensemble model to identify important features for the prediction. In addition, this paper follows the MINIMAR (MINimum Information for Medical AI Reporting) standard, presenting an important step towards building trust among the AI system and clinicians.
更多查看译文
关键词
interpretable multimodal predictive models,early mortality prediction,hemorrhagic stroke patients
AI 理解论文
溯源树
样例
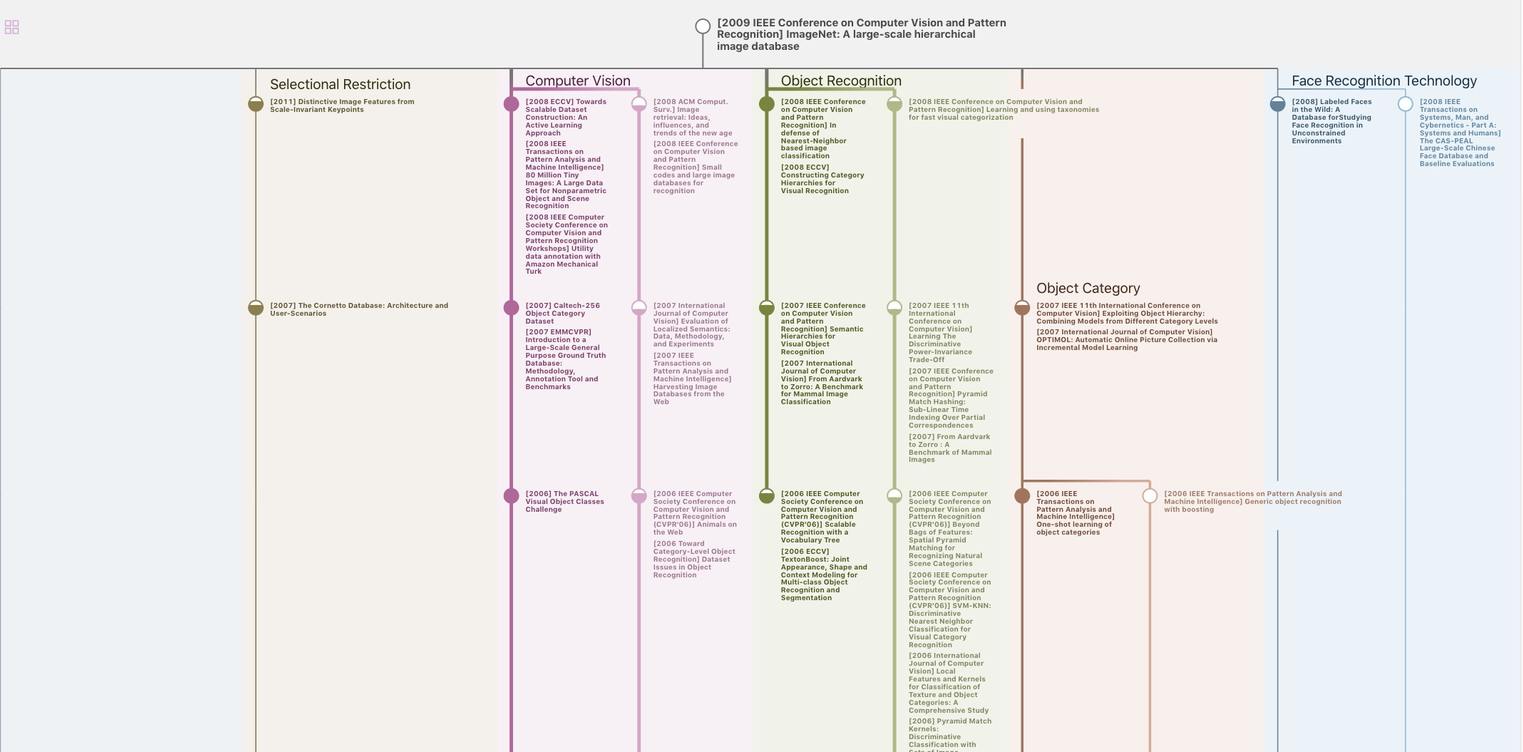
生成溯源树,研究论文发展脉络
Chat Paper
正在生成论文摘要