Behind-the-Meter Load and PV Disaggregation via Deep Spatiotemporal Graph Generative Sparse Coding With Capsule Network
IEEE transactions on neural networks and learning systems(2023)
摘要
Nowadays, rooftop photovoltaic (PV) panels are getting enormous attention as clean and sustainable sources of energy due to the increasing energy demand, depreciating phys-ical assets, and global environmental challenges. In residential areas, the large-scale integration of these generation resources influences the customer load profile and introduces uncertainty to the distribution system's net load. Since such resources are typically located behind the meter (BtM), an accurate estimation of BtM load and PV power will be crucial for distribution network operation. This article proposes the spatiotemporal graph sparse coding (SC) capsule network that incorporates SC into deep generative graph modeling and capsule networks for accurate BtM load and PV generation estimation. A set of neighboring residential units are modeled as a dynamic graph in which the edges represent the correlation among their net demands. A generative encoder-decoder model, i.e., spectral graph convolution (SGC) attention peephole long short-term memory (PLSTM), is devised to extract the highly nonlinear spatiotemporal patterns from the formed dynamic graph. Later, to enrich the latent space sparsity, a dictionary is learned in the hidden layer of the proposed encoder-decoder, and the corre-sponding sparse codes are procured. Such sparse representation is used by a capsule network to estimate the BtM PV generation and the load of the entire residential units. Experimental results on two real-world energy disaggregation (ED) datasets, Pecan Street and Ausgrid, demonstrate more than 9.8% and 6.3% root mean square error (RMSE) improvements in BtM PV and load estimation over the state-of-the-art, respectively.
更多查看译文
关键词
Load modeling,Spatiotemporal phenomena,Feature extraction,Hidden Markov models,Correlation,Estimation,Encoding,Behind-the-meter (BtM) disaggregation,capsule networks,graph generative,long short-term memory (LSTM),sparse coding (SC),spatiotemporal correlations
AI 理解论文
溯源树
样例
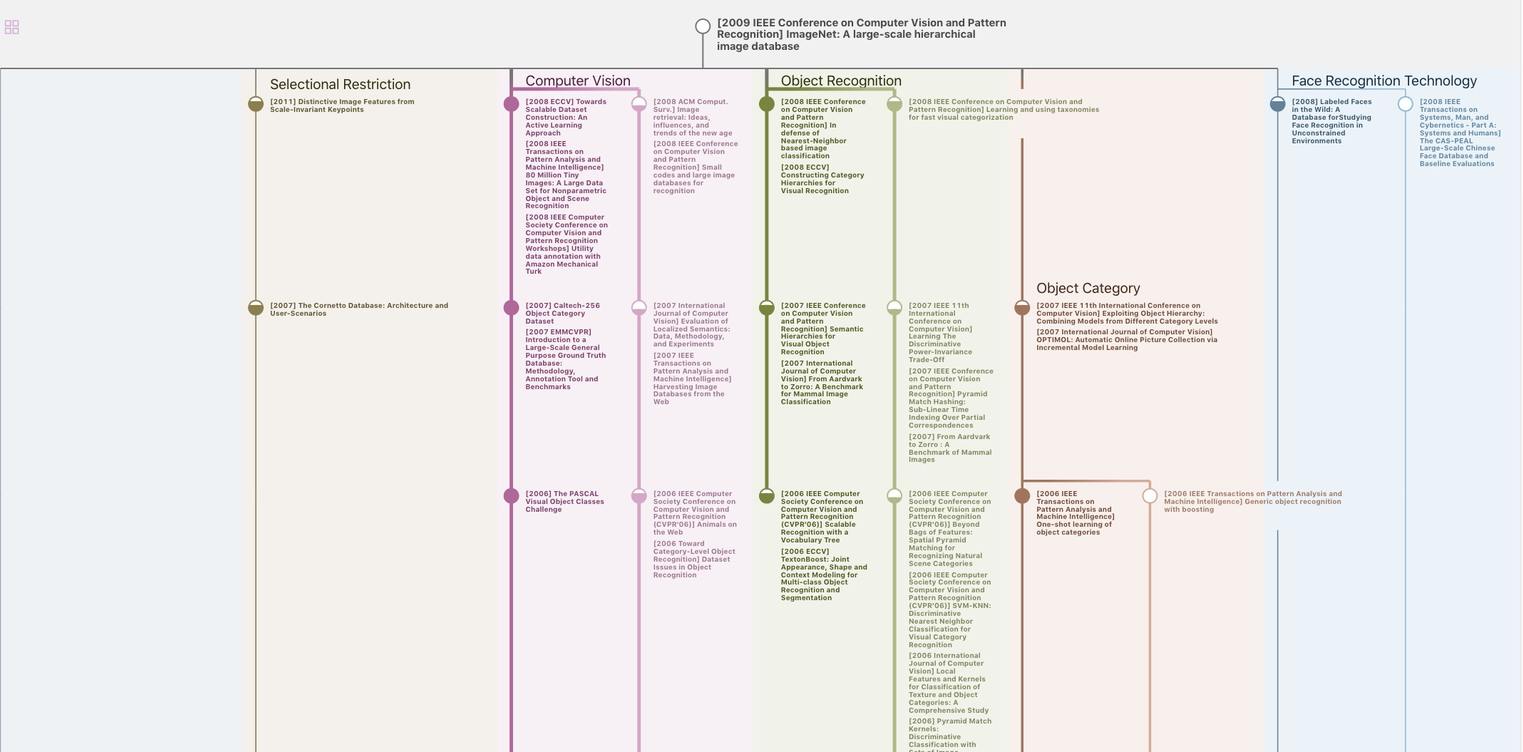
生成溯源树,研究论文发展脉络
Chat Paper
正在生成论文摘要