Artificial Intelligence Applied to Contrast-enhanced Mammography: Exploring Uncharted Territory.
Radiology(2023)
Abstract
HomeRadiologyVol. 307, No. 5 PreviousNext Reviews and CommentaryEditorialArtificial Intelligence Applied to Contrast-enhanced Mammography: Exploring Uncharted TerritoryManisha Bahl , Synho DoManisha Bahl , Synho DoAuthor AffiliationsFrom the Department of Radiology, Massachusetts General Hospital, 55 Fruit St, WAC 240, Boston, MA 02114.Address correspondence to M.B. (email: [email protected]).Manisha Bahl Synho DoPublished Online:Jun 20 2023https://doi.org/10.1148/radiol.231140MoreSectionsFull textPDF ToolsImage ViewerAdd to favoritesCiteTrack CitationsPermissionsReprints ShareShare onFacebookTwitterLinked In References1. Jochelson MS, Lobbes MBI. Contrast-enhanced mammography: state of the art. Radiology 2021;299(1):36–48. Link, Google Scholar2. Ghaderi KF, Phillips J, Perry H, Lotfi P, Mehta TS. Contrast-enhanced mammography: current applications and future directions. RadioGraphics 2019;39(7):1907–1920. Link, Google Scholar3. AI Central. American College of Radiology Data Science Institute. https://aicentral.acrdsi.org. Accessed April 21, 2023. Google Scholar4. Bahl M. Artificial intelligence: a primer for breast imaging radiologists. J Breast Imaging 2020;2(4):304–314. Crossref, Medline, Google Scholar5. Hosny A, Aerts HJ, Mak RH. Handcrafted versus deep learning radiomics for prediction of cancer therapy response. Lancet Digit Health 2019;1(3):e106–e107. Crossref, Medline, Google Scholar6. Beuque MPL, Lobbes MBI, van Wijk Y, et al. Combining deep learning and handcrafted radiomics for classification of suspicious lesions on contrast-enhanced mammograms. Radiology 2023;307(5):e221843. Link, Google Scholar7. Jones MA, Faiz R, Qiu Y, Zheng B. Improving mammography lesion classification by optimal fusion of handcrafted and deep transfer learning features. Phys Med Biol 2022;67(5): https://doi.org/10.1088/1361-6560/ac5297. Crossref, Google Scholar8. Zheng T, Lin F, Li X, et al. Deep learning-enabled fully automated pipeline system for segmentation and classification of single-mass breast lesions using contrast-enhanced mammography: a prospective, multicentre study. EclinicalMedicine 2023;58:101913. Crossref, Medline, Google Scholar9. Grimm LJ, Rahbar H, Abdelmalak M, Hall AH, Ryser MD. Ductal carcinoma in situ: state-of-the-art review. Radiology 2022;302(2):246–255. Link, Google Scholar10. Pötsch N, Vatteroni G, Clauser P, Helbich TH, Baltzer PAT. Contrast-enhanced mammography versus contrast-enhanced breast MRI: a systematic review and meta-analysis. Radiology 2022;305(1):94–103. Link, Google ScholarArticle HistoryReceived: May 2 2023Revision requested: May 16 2023Revision received: May 18 2023Accepted: May 19 2023Published online: June 20 2023 FiguresReferencesRelatedDetailsAccompanying This ArticleCombining Deep Learning and Handcrafted Radiomics for Classification of Suspicious Lesions on Contrast-enhanced MammogramsJun 20 2023RadiologyRecommended Articles RSNA Education Exhibits RSNA Case Collection Vol. 307, No. 5 Metrics Altmetric Score PDF download
MoreTranslated text
AI Read Science
Must-Reading Tree
Example
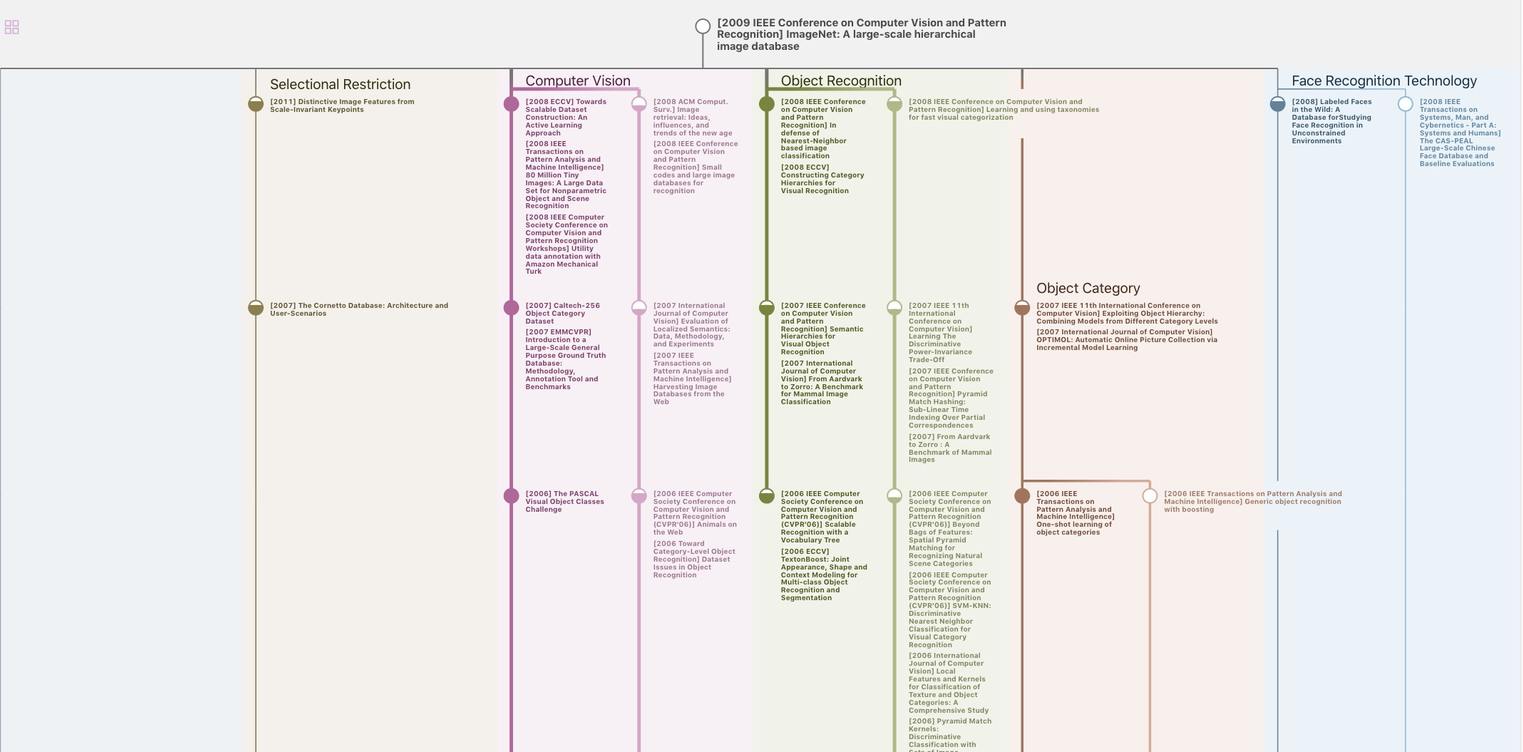
Generate MRT to find the research sequence of this paper
Chat Paper
Summary is being generated by the instructions you defined