Causal decomposition maps: An exploratory tool for designing area-level interventions aimed at reducing health disparities
Biometrical journal. Biometrische Zeitschrift(2023)
摘要
Methods for decomposition analyses have been developed to partition between-group differences into explained and unexplained portions. In this paper, we introduce the concept of causal decomposition maps, which allow researchers to test the effect of area-level interventions on disease maps before implementation. These maps quantify the impact of interventions that aim to reduce differences in health outcomes between groups and illustrate how the disease map might change under different interventions. We adapt a new causal decomposition analysis method for the disease mapping context. Through the specification of a Bayesian hierarchical outcome model, we obtain counterfactual small area estimates of age-adjusted rates and reliable estimates of decomposition quantities. We present two formulations of the outcome model, with the second allowing for spatial interference of the intervention. Our method is utilized to determine whether the addition of gyms in different sets of rural ZIP codes could reduce any of the rural-urban difference in age-adjusted colorectal cancer incidence rates in Iowa ZIP codes.
更多查看译文
关键词
Bayesian hierarchical models,counterfactual outcomes,decomposition analysis,disease mapping,spatial interference
AI 理解论文
溯源树
样例
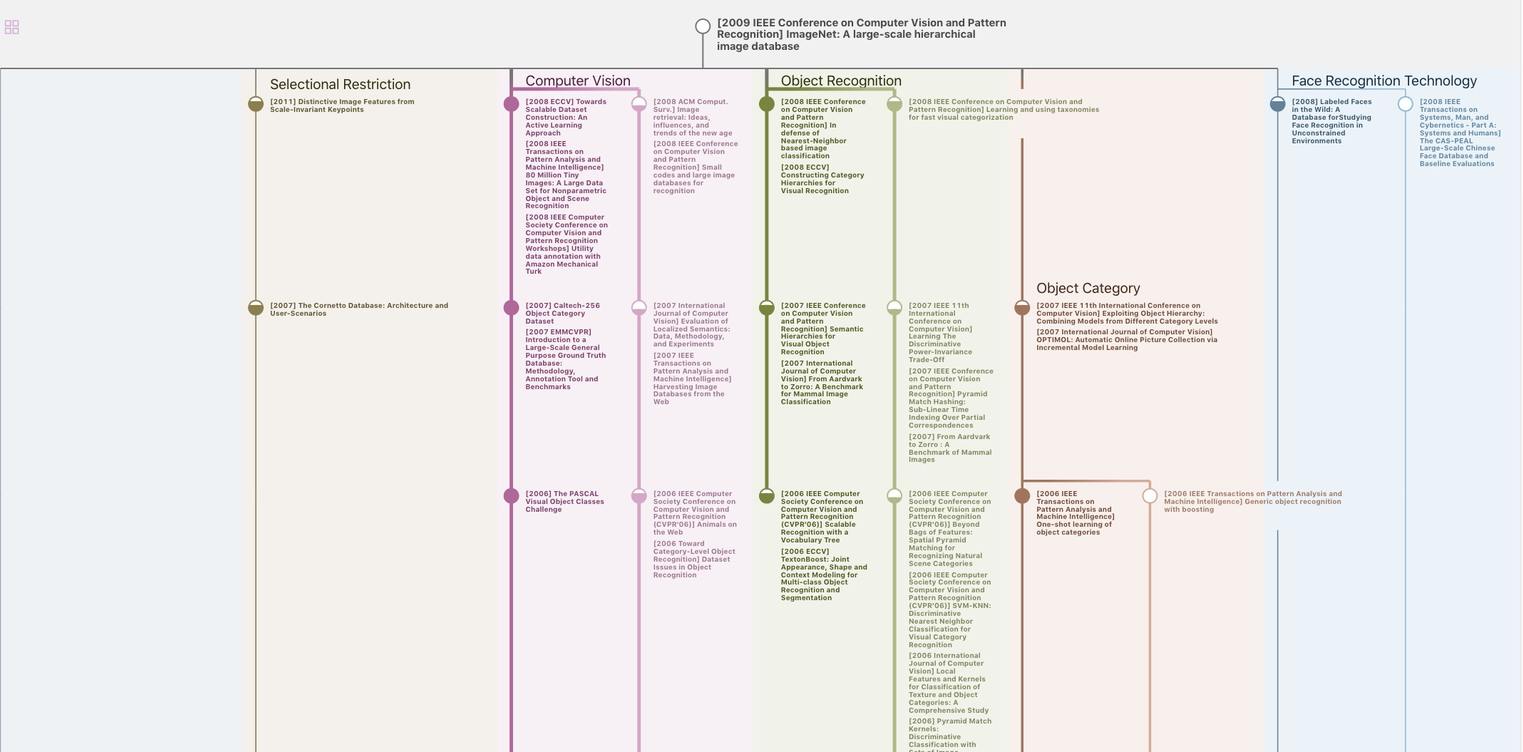
生成溯源树,研究论文发展脉络
Chat Paper
正在生成论文摘要