Prediction of Knee Joint Compartmental Loading Maxima Utilizing Simple Subject Characteristics and Neural Networks
Annals of biomedical engineering(2023)
Abstract
Joint loading may affect the development of osteoarthritis, but patient-specific load estimation requires cumbersome motion laboratory equipment. This reliance could be eliminated using artificial neural networks (ANNs) to predict loading from simple input predictors. We used subject-specific musculoskeletal simulations to estimate knee joint contact forces for 290 subjects during over 5000 stance phases of walking and then extracted compartmental and total joint loading maxima from the first and second peaks of the stance phase. We then trained ANN models to predict the loading maxima from predictors that can be measured without motion laboratory equipment (subject mass, height, age, gender, knee abduction-adduction angle, and walking speed). When compared to the target data, our trained models had NRMSEs (RMSEs normalized to the mean of the response variable) between 0.14 and 0.42 and Pearson correlation coefficients between 0.42 and 0.84. The loading maxima were predicted most accurately using the models trained with all predictors. We demonstrated that prediction of knee joint loading maxima may be possible without laboratory-measured motion capture data. This is a promising step in facilitating knee joint loading predictions in simple environments, such as a physician’s appointment. In future, the rapid measurement and analysis setup could be utilized to guide patients in rehabilitation to slow development of joint disorders, such as osteoarthritis.
MoreTranslated text
Key words
Knee joint,Contact force,Compartmental loading,Knee osteoarthritis,Gait analysis,Artificial neural networks,OpenSim
AI Read Science
Must-Reading Tree
Example
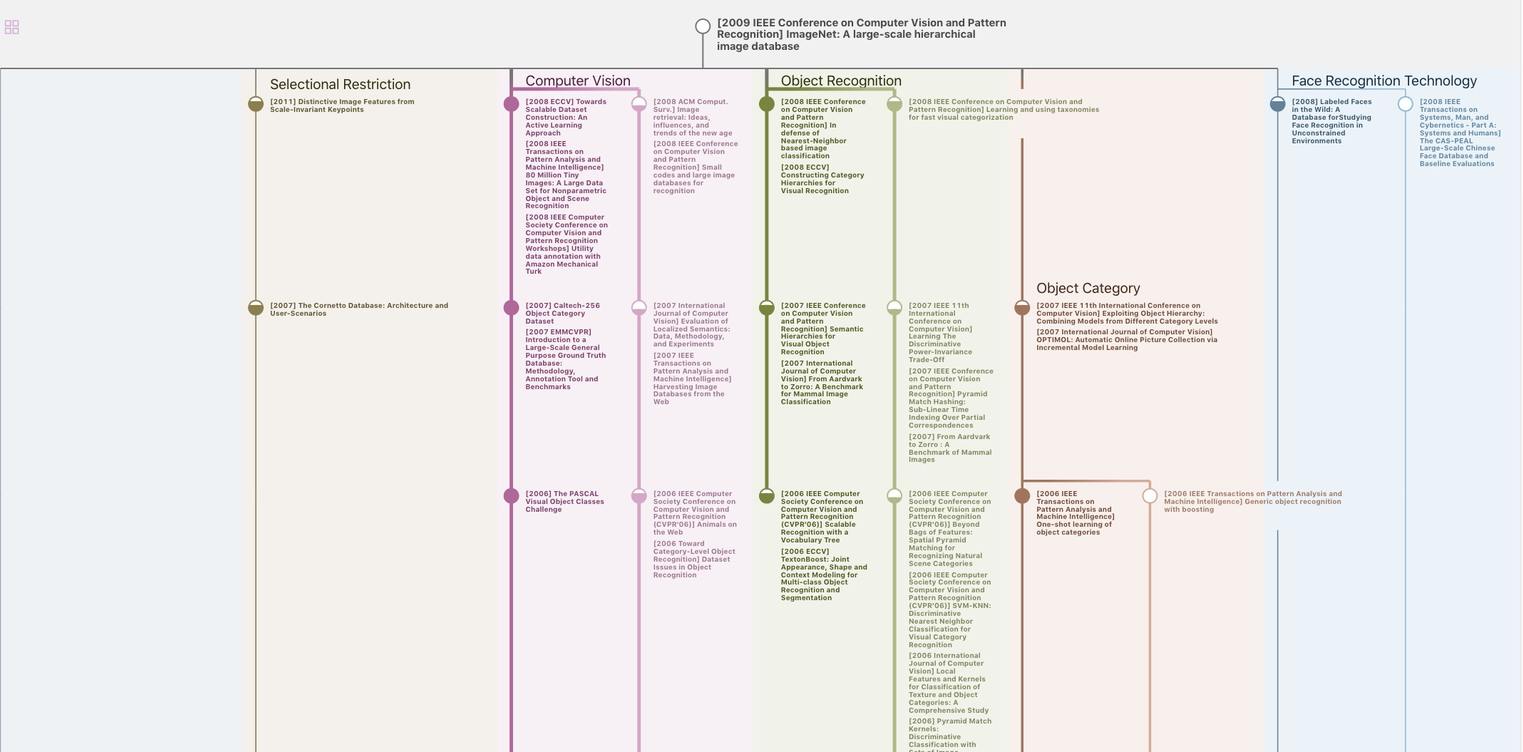
Generate MRT to find the research sequence of this paper
Chat Paper
Summary is being generated by the instructions you defined