Distinguishing between different percolation regimes in noisy dynamic networks with an application to epileptic seizures.
PLoS computational biology(2023)
摘要
In clinical neuroscience, epileptic seizures have been associated with the sudden emergence of coupled activity across the brain. The resulting functional networks-in which edges indicate strong enough coupling between brain regions-are consistent with the notion of percolation, which is a phenomenon in complex networks corresponding to the sudden emergence of a giant connected component. Traditionally, work has concentrated on noise-free percolation with a monotonic process of network growth, but real-world networks are more complex. We develop a class of random graph hidden Markov models (RG-HMMs) for characterizing percolation regimes in noisy, dynamically evolving networks in the presence of edge birth and edge death. This class is used to understand the type of phase transitions undergone in a seizure, and in particular, distinguishing between different percolation regimes in epileptic seizures. We develop a hypothesis testing framework for inferring putative percolation mechanisms. As a necessary precursor, we present an EM algorithm for estimating parameters from a sequence of noisy networks only observed at a longitudinal subsampling of time points. Our results suggest that different types of percolation can occur in human seizures. The type inferred may suggest tailored treatment strategies and provide new insights into the fundamental science of epilepsy.
更多查看译文
关键词
noisy dynamic networks,different percolation regimes,epileptic seizures
AI 理解论文
溯源树
样例
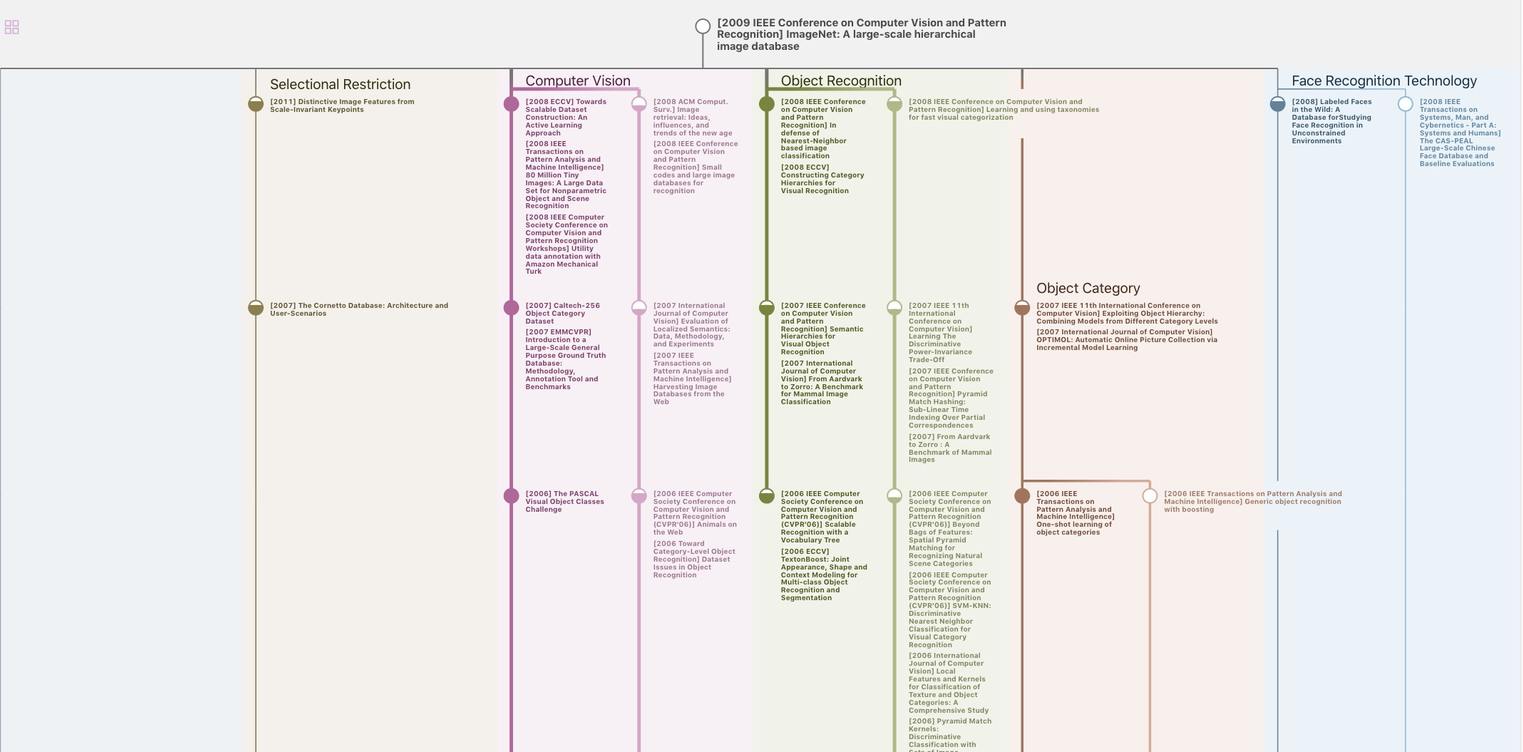
生成溯源树,研究论文发展脉络
Chat Paper
正在生成论文摘要