WPConvNet: An Interpretable Wavelet Packet Kernel-Constrained Convolutional Network for Noise-Robust Fault Diagnosis.
IEEE transactions on neural networks and learning systems(2023)
摘要
Deep learning (DL) has present great diagnostic results in fault diagnosis field. However, the poor interpretability and noise robustness of DL-based methods are still the main factors limiting their wide application in industry. To address these issues, an interpretable wavelet packet kernel-constrained convolutional network (WPConvNet) is proposed for noise-robust fault diagnosis, which combines the feature extraction ability of wavelet bases and the learning ability of convolutional kernels together. First, the wavelet packet convolutional (WPConv) layer is proposed, and constraints are imposed to convolutional kernels, so that each convolution layer is a learnable discrete wavelet transform. Second, a soft threshold activation is proposed to reduce the noise component in feature maps, whose threshold is adaptively learned by estimating the standard deviation of noise. Third, we link the cascaded convolutional structure of convolutional neutral network (CNN) with wavelet packet decomposition and reconstruction using Mallat algorithm, which is interpretable in model architecture. Extensive experiments are carried out on two bearing fault datasets, and the results show that the proposed architecture outperforms other diagnosis models in terms of interpretability and noise robustness.
更多查看译文
关键词
fault diagnosis,wavelet,kernel-constrained,noise-robust
AI 理解论文
溯源树
样例
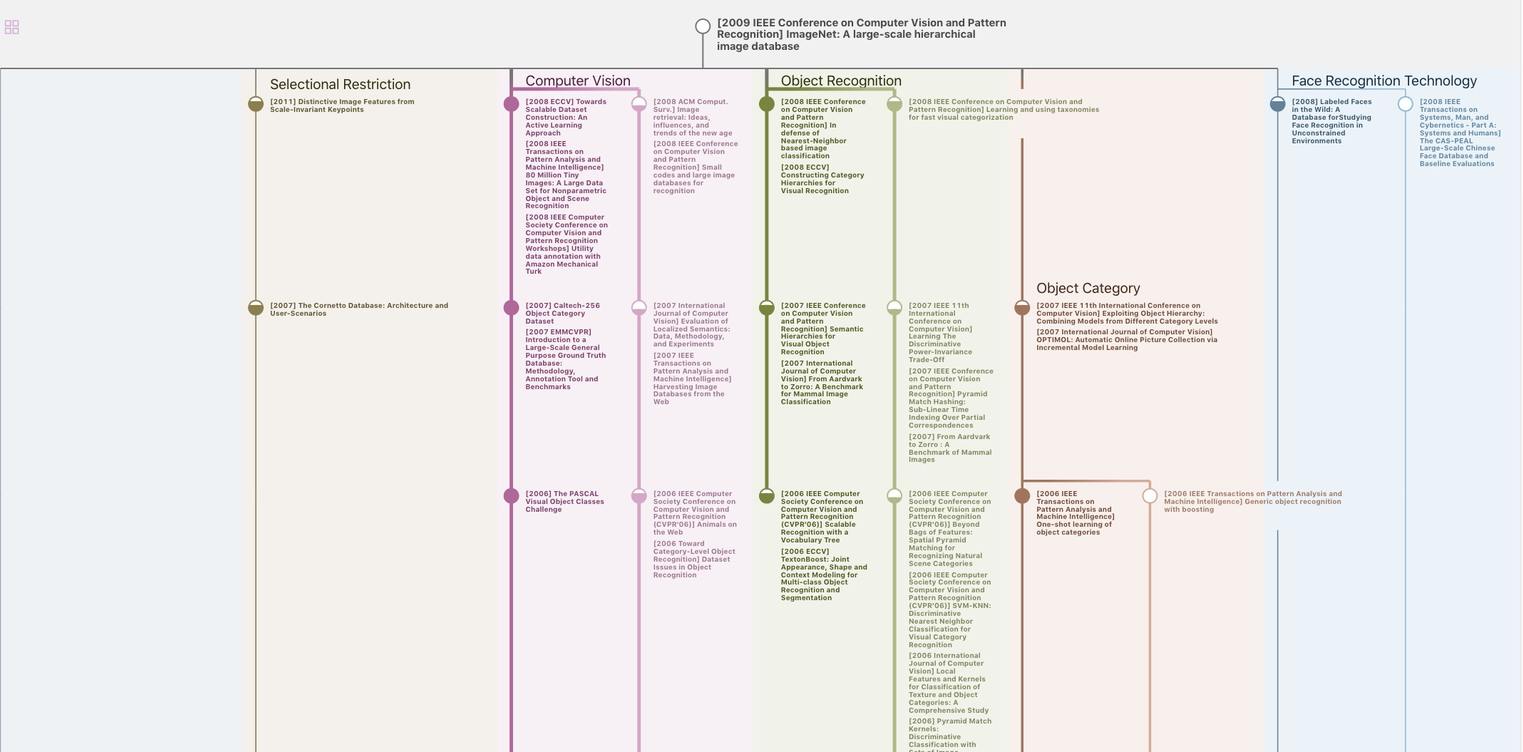
生成溯源树,研究论文发展脉络
Chat Paper
正在生成论文摘要