Pulmonary contusion: automated deep learning-based quantitative visualization
Emergency radiology(2023)
摘要
Purpose Rapid automated CT volumetry of pulmonary contusion may predict progression to Acute Respiratory Distress Syndrome (ARDS) and help guide early clinical management in at-risk trauma patients. This study aims to train and validate state-of-the-art deep learning models to quantify pulmonary contusion as a percentage of total lung volume (Lung Contusion Index, or auto-LCI) and assess the relationship between auto-LCI and relevant clinical outcomes. Methods 302 adult patients (age ≥ 18) with pulmonary contusion were retrospectively identified from reports between 2016 and 2021. nnU-Net was trained on manual contusion and whole-lung segmentations. Point-of-care candidate variables for multivariate regression included oxygen saturation, heart rate, and systolic blood pressure on admission. Logistic regression was used to assess ARDS risk, and Cox proportional hazards models were used to determine differences in ICU length of stay and mechanical ventilation time. Results Mean Volume Similarity Index and mean Dice scores were 0.82 and 0.67. Interclass correlation coefficient and Pearson r between ground-truth and predicted volumes were 0.90 and 0.91. 38 (14%) patients developed ARDS. In bivariate analysis, auto-LCI was associated with ARDS ( p < 0.001), ICU admission ( p < 0.001), and need for mechanical ventilation ( p < 0.001). In multivariate analyses, auto-LCI was associated with ARDS ( p = 0.04), longer length of stay in the ICU ( p = 0.02) and longer time on mechanical ventilation ( p = 0.04). AUC of multivariate regression to predict ARDS using auto-LCI and clinical variables was 0.70 while AUC using auto-LCI alone was 0.68. Conclusion Increasing auto-LCI values corresponded with increased risk of ARDS, longer ICU admissions, and longer periods of mechanical ventilation.
更多查看译文
关键词
ARDS, Artificial intelligence, Pulmonary contusion, Quantitative imaging, Segmentation
AI 理解论文
溯源树
样例
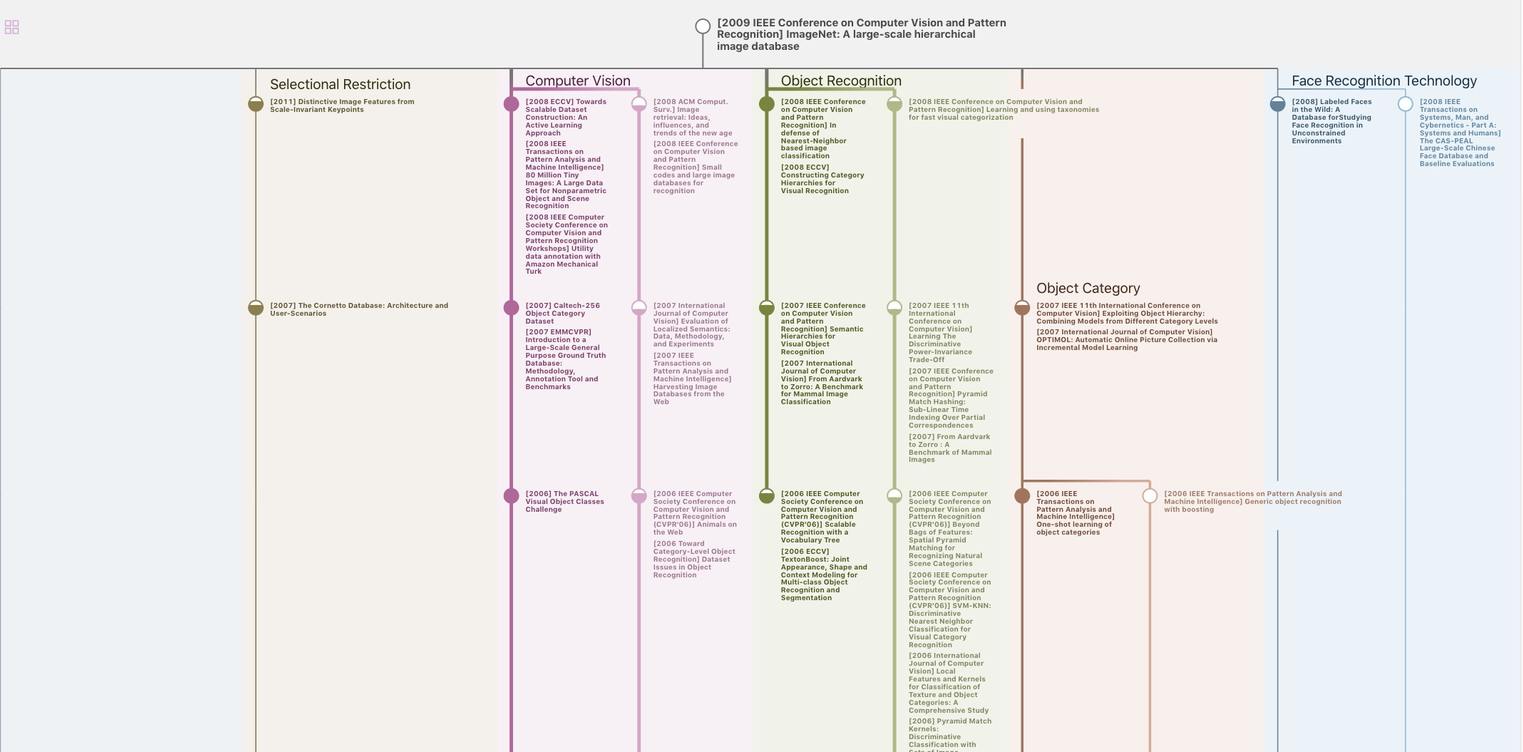
生成溯源树,研究论文发展脉络
Chat Paper
正在生成论文摘要