Fully Automated Longitudinal Assessment of Renal Stone Burden on Serial CT Imaging Using Deep Learning.
Journal of endourology(2023)
摘要
Use deep learning (DL) to automate the measurement and tracking of kidney stone burden over serial computed tomography (CT) scans. Materials and Methods This retrospective study included 259 scans from 113 symptomatic patients being treated for urolithiasis at a single medical center between 2006 and 2019. These patients underwent a standard low-dose (SLD) non-contrast CT scan followed by ultra-low dose (ULD) CT scans limited to the level of the kidneys. A DL model was used to detect, segment, and measure the volume of all stones in both initial and follow-up scans. The stone burden was characterized by the total volume of all stones in a scan (SV). The absolute and relative change of SV, (SVA and SVR, respectively) over serial scans were computed. The automated assessments were compared with manual assessments using Concordance Correlation Coefficient (CCC), and their agreement was visualized using Bland-Altman and scatter plots. Results 228/233 scans with stones were identified by the automated pipeline; per-scan sensitivity was 97.8% (95% CI: [96.0 - 99.7]). The per-scan positive predictive value was 96.6% (95% CI: [94.4 - 98.8]). The median SV, SVA and SVR were 476.5 mm3, -10 mm3 and 0.89, respectively. After removing outliers outside the 5th and 95th percentiles, the CCC measuring agreement on SV, SVA and SVR were 0.995 (0.992 - 0.996), 0.980 (0.972 - 0.986), and 0.915 (0.881 - 0.939), respectively. The mean effective dose was 4.1 mSv for the ULD compared to 13.4 mSv for the SLD (p<0.01). Conclusions The automated DL-based measurements showed good agreement with the manual assessments of the stone burden and its interval change on serial CT scans.
更多查看译文
关键词
renal stone burden,serial ct imaging,deep learning,automated longitudinal assessment
AI 理解论文
溯源树
样例
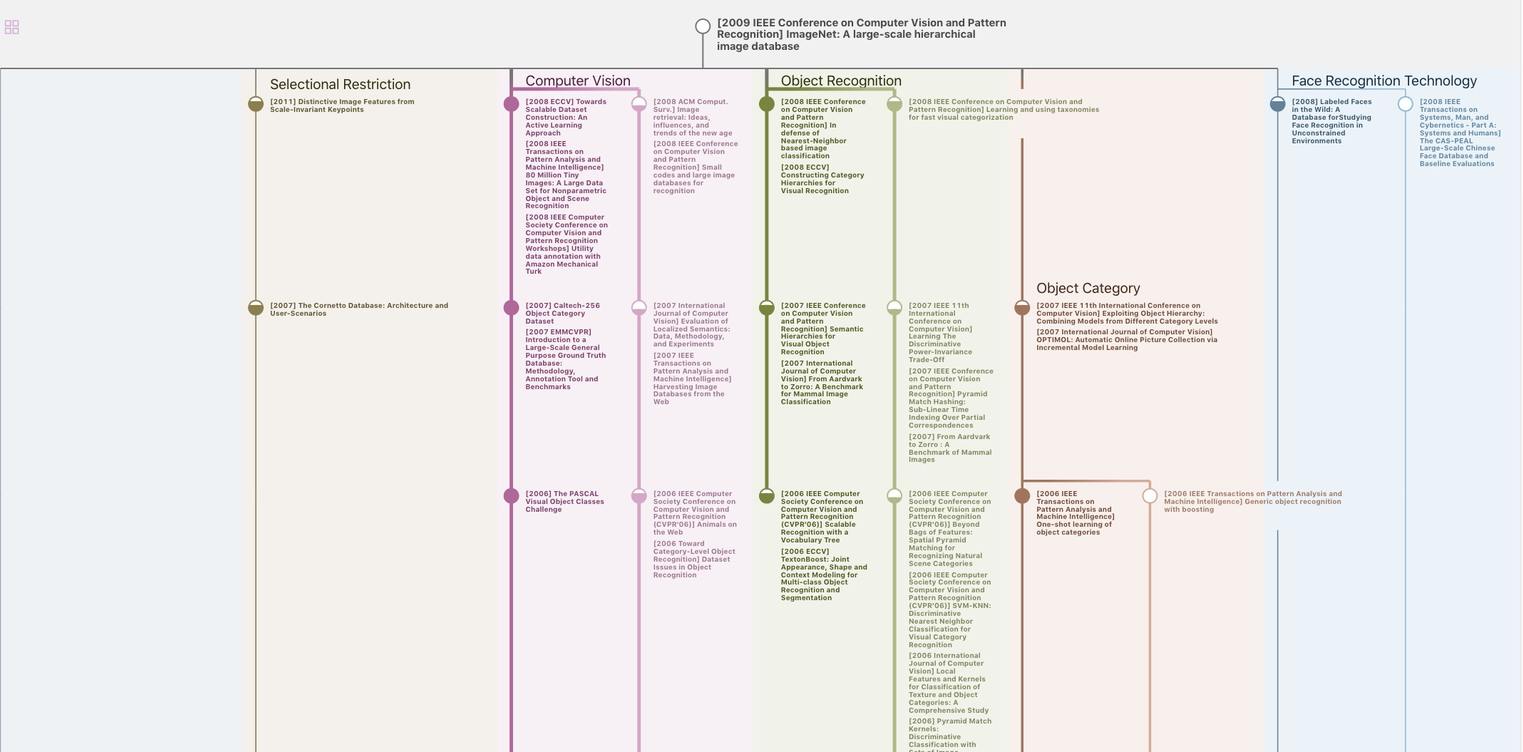
生成溯源树,研究论文发展脉络
Chat Paper
正在生成论文摘要